Closing the life-cycle of normative modeling using federated hierarchical Bayesian regression.
PloS one(2022)
摘要
Clinical neuroimaging data availability has grown substantially in the last decade, providing the potential for studying heterogeneity in clinical cohorts on a previously unprecedented scale. Normative modeling is an emerging statistical tool for dissecting heterogeneity in complex brain disorders. However, its application remains technically challenging due to medical data privacy issues and difficulties in dealing with nuisance variation, such as the variability in the image acquisition process. Here, we approach the problem of estimating a reference normative model across a massive population using a massive multi-center neuroimaging dataset. To this end, we introduce a federated probabilistic framework using hierarchical Bayesian regression (HBR) to complete the life-cycle of normative modeling. The proposed model provides the possibilities to learn, update, and adapt the model parameters on decentralized neuroimaging data. Our experimental results confirm the superiority of HBR in deriving more accurate normative ranges on large multi-site neuroimaging datasets compared to the current standard methods. In addition, our approach provides the possibility to recalibrate and reuse the learned model on local datasets and even on datasets with very small sample sizes. The proposed method will facilitate applications of normative modeling as a medical tool for screening the biological deviations in individuals affected by complex illnesses such as mental disorders.
更多查看译文
关键词
hierarchical bayesian regression,normative modeling,life-cycle
AI 理解论文
溯源树
样例
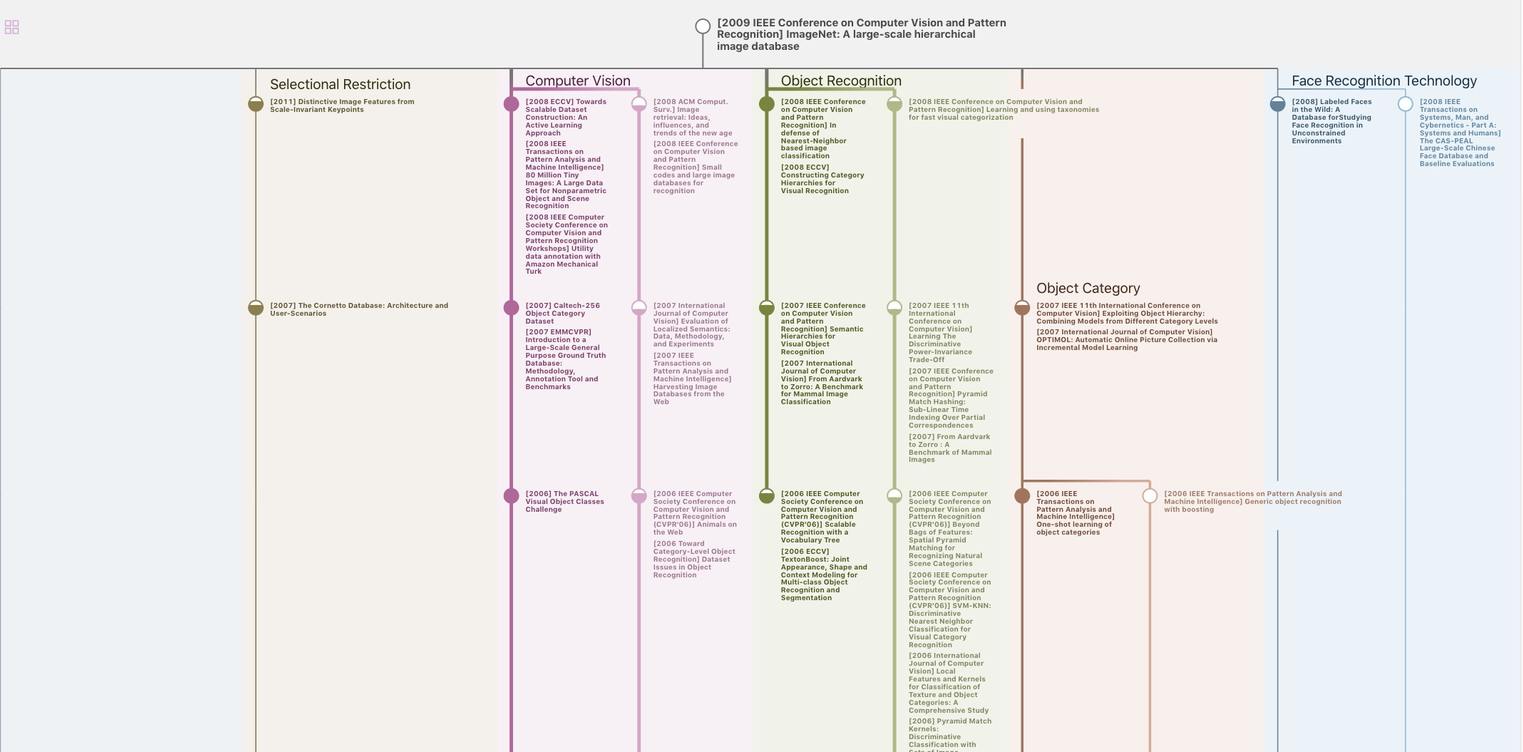
生成溯源树,研究论文发展脉络
Chat Paper
正在生成论文摘要