Machine learning-based clustering and classification of mouse behaviors via respiratory patterns.
iScience(2022)
摘要
Breathing is dynamically modulated by metabolic needs as well as by emotional states. Even though rodents are invaluable models for investigating the neural control of respiration, current literature lacks systematic characterization of breathing dynamics across a broad spectrum of rodent behaviors. Here we uncover a wide diversity in breathing patterns across spontaneous, attractive odor-, stress-, and fear-induced behaviors in mice. Direct recordings of intranasal pressure afford more detailed respiratory information than more traditional whole-body plethysmography. K-means clustering groups 11 well-defined behavioral states into four clusters with distinct key respiratory features. Furthermore, we implement RUSBoost (random undersampling boost) classification, a supervised machine learning model, and find that breathing patterns can separate these behaviors with an accuracy of 80%. Taken together, our findings highlight the tight relationship between breathing and behavior and the potential use of breathing patterns to aid in distinguishing similar behaviors and inform about their internal states.
更多查看译文
关键词
Artificial intelligence,Behavioral neuroscience,Neuroscience
AI 理解论文
溯源树
样例
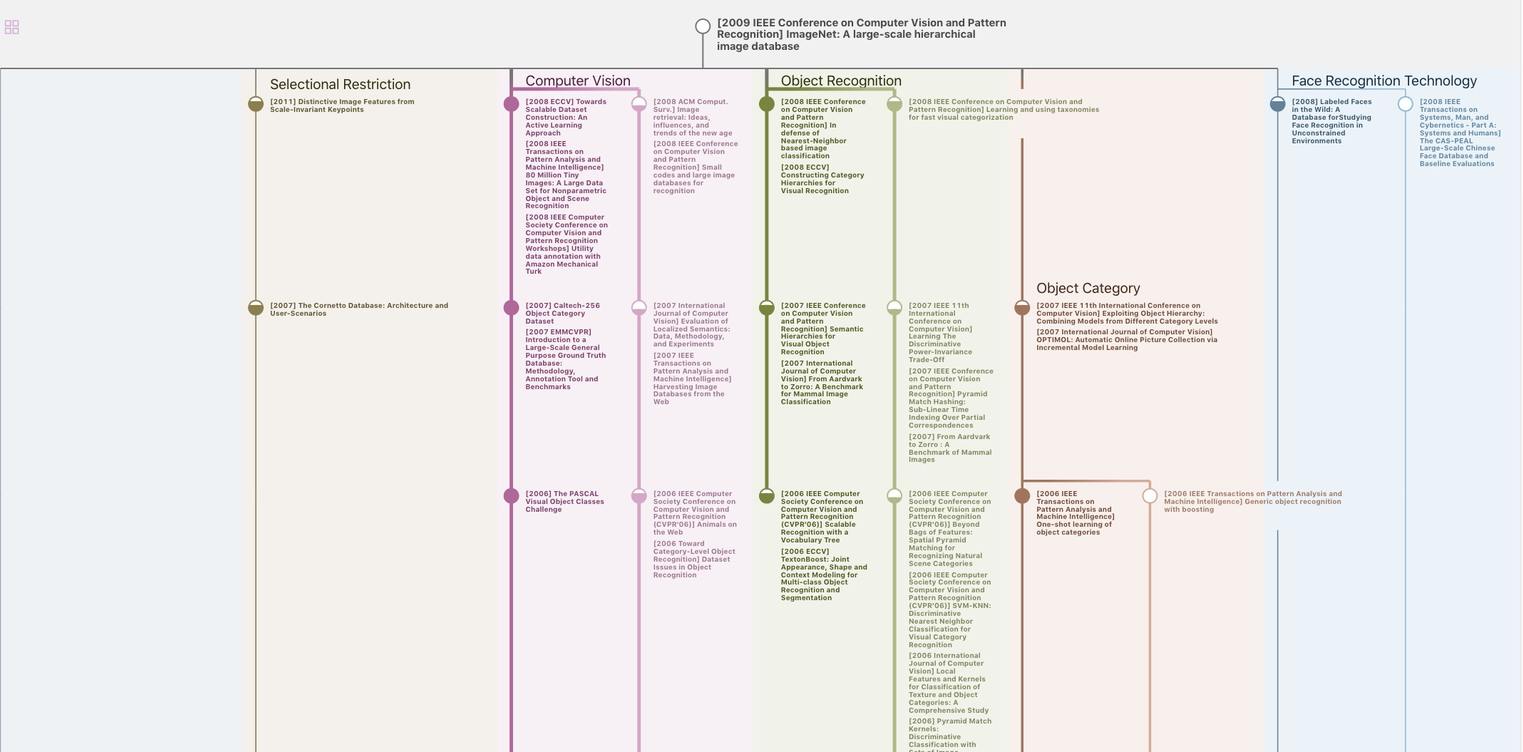
生成溯源树,研究论文发展脉络
Chat Paper
正在生成论文摘要