Comparison of Oncologist and Model Estimates of Risk for Hospitalization During Systemic Therapy for Advanced Cancer
JCO oncology practice(2023)
摘要
PURPOSE:A validated risk model with inputs of pretreatment sodium and albumin can identify patients at risk for hospitalization during cancer treatment. We evaluated how the model compares with risk estimates from treating oncologists. METHODS:We evaluated the 30-day risk of hospitalization or death in patients starting palliative-intent systemic therapy for solid tumor malignancy. For each patient, we prospectively recorded categorical estimates of 30-day hospitalization risk (bottom third, middle third, top third) generated by a treating oncologist and by the two-variable model; a third hybrid risk estimate represented a composite of the oncologist and model risk assessments. We analyzed the agreement of oncologist and model-based risk estimates and compared discrimination, sensitivity, and specificity of each risk assessment method. RESULTS:We collected oncologist, model, and hybrid estimates of hospitalization risk for 120 patients. The 30-day rate of hospitalization or death was 20%. There was minimal agreement between oncologist and model risk estimates (weighted kappa = 0.27). The c-statistic (a measure of discrimination) was 0.69 (95% CI, 0.57 to 0.81) for the clinician assessment, 0.77 for the model assessment (CI, 0.67 to 0.86; P = .24 compared with the oncologist assessment), and 0.79 for the hybrid assessment (CI, 0.69 to 0.90; P = .007 compared with the oncologist assessment). Sensitivity and specificity of the high-risk categorization did not differ significantly between the oncologist and model assessments; the hybrid assessment was significantly more sensitive (P = .02) and less specific (P = .03) than the oncologist assessment. CONCLUSION:A model with inputs of pretreatment sodium and albumin improves oncologists' predictions of hospitalization risk during cancer treatment.
更多查看译文
关键词
oncologist,hospitalization,cancer,systemic therapy
AI 理解论文
溯源树
样例
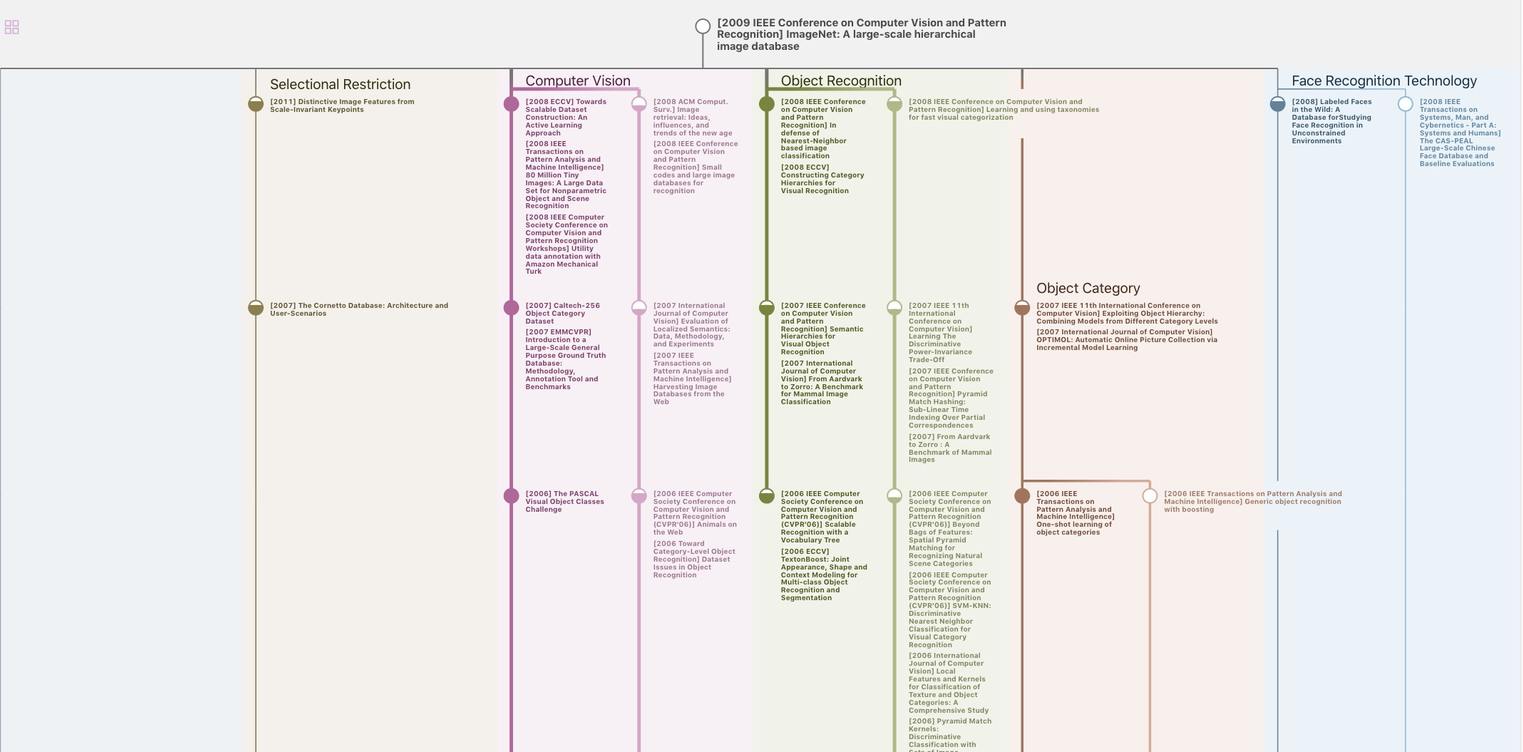
生成溯源树,研究论文发展脉络
Chat Paper
正在生成论文摘要