Reward Shaping-based Double Deep Q-networks for Unmanned Surface Vessel Navigation and Obstacle Avoidance
IECON 2022 – 48th Annual Conference of the IEEE Industrial Electronics Society(2022)
摘要
In this paper, a method for navigation and obstacle avoidance of unmanned surface vessel (USV) based on reinforcement learning and reward shaping is proposed. This approach uses double deep Q networks (DDQN) to make decisions based on the continuous states observed from sensors in USV. In addition, a new reward function is designed based on prior knowledge to accelerate the convergence of the algorithm and improve the performance. For training the neural networks, a simulation platform is developed, in which a 3 degree of freedom mathematical model describes USV dynamic system and two-dimension actions are required to control USV. Simulation results on the platform demonstrate the DDQN hoists USV’s capabilities of navigation and obstacle avoidance, and reward shaping technique improves the speed of convergence.
更多查看译文
关键词
Unmanned surface vessel,navigation,obstacle avoidance,reinforcement learning,reward shaping
AI 理解论文
溯源树
样例
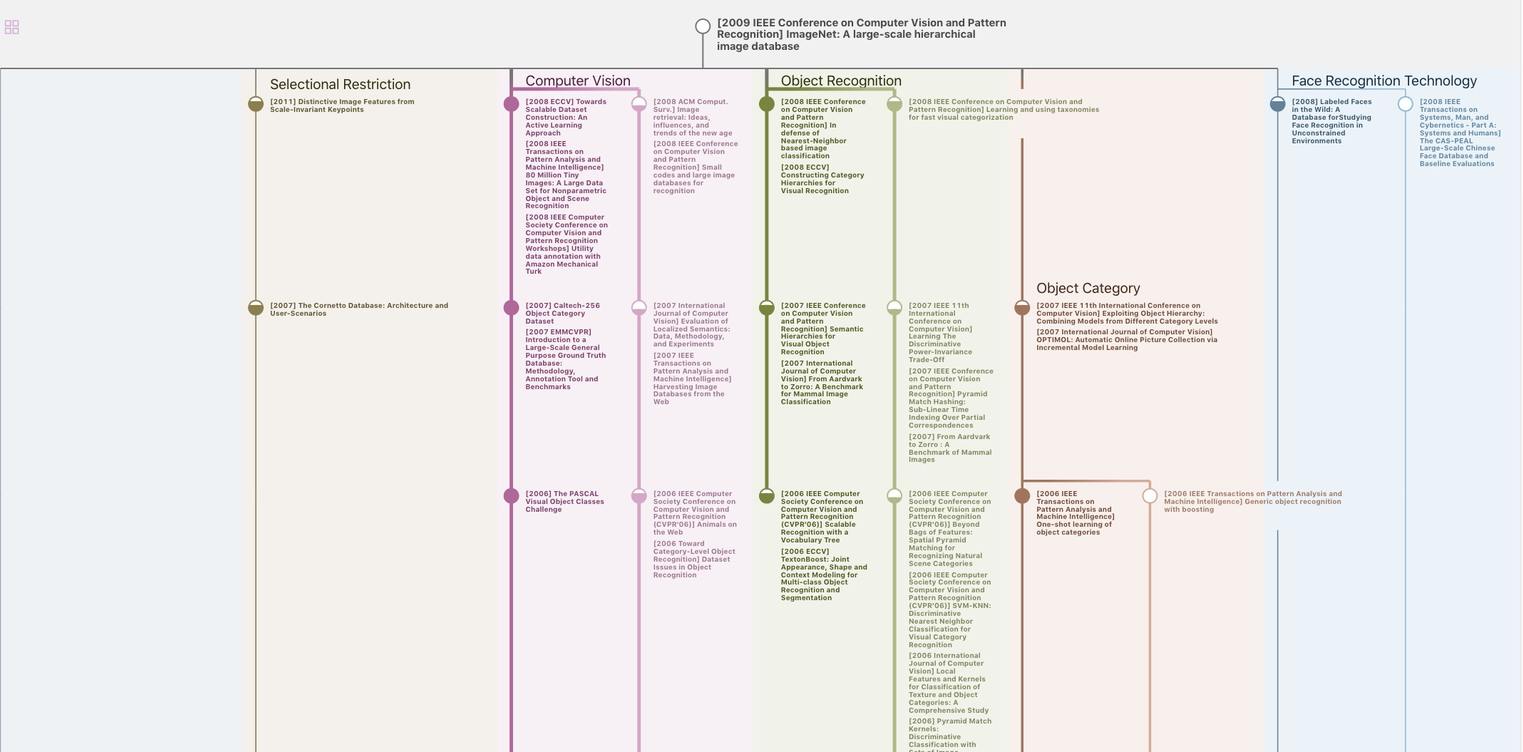
生成溯源树,研究论文发展脉络
Chat Paper
正在生成论文摘要