Reliable Contrastive Learning for Semi-supervised Change Detection in Remote Sensing Images
IEEE Transactions on Geoscience and Remote Sensing(2022)
摘要
With the development of deep learning in remote sensing (RS) image change detection (CD), the dependence of CD models on labeled data has become an important problem. To make better use of the comparatively resource-saving unlabeled data, the CD method based on semi-supervised learning (SSL) is worth further study. This article proposes a reliable contrastive learning (RCL) method for semi-supervised RS image CD. First, according to the task characteristics of CD, we design the contrastive loss based on the changed areas to enhance the model's feature extraction ability for changed objects. Then, to improve the quality of pseudo labels in SSL, we use the uncertainty of unlabeled data to select reliable pseudo labels for model training. Combining these methods, semi-supervised CD models can make full use of unlabeled data. Extensive experiments on three widely used CD datasets demonstrate the effectiveness of the proposed method. The results show that our semi-supervised approach has a better performance than related methods. The code is available at https://github.com/VCISwang/RC-Change-Detection.
更多查看译文
关键词
Feature extraction,Data models,Reliability,Remote sensing,Training,Predictive models,Uncertainty,Change detection (CD),contrastive learning,remote sensing (RS),semantic segmentation,semi-supervised learning (SSL)
AI 理解论文
溯源树
样例
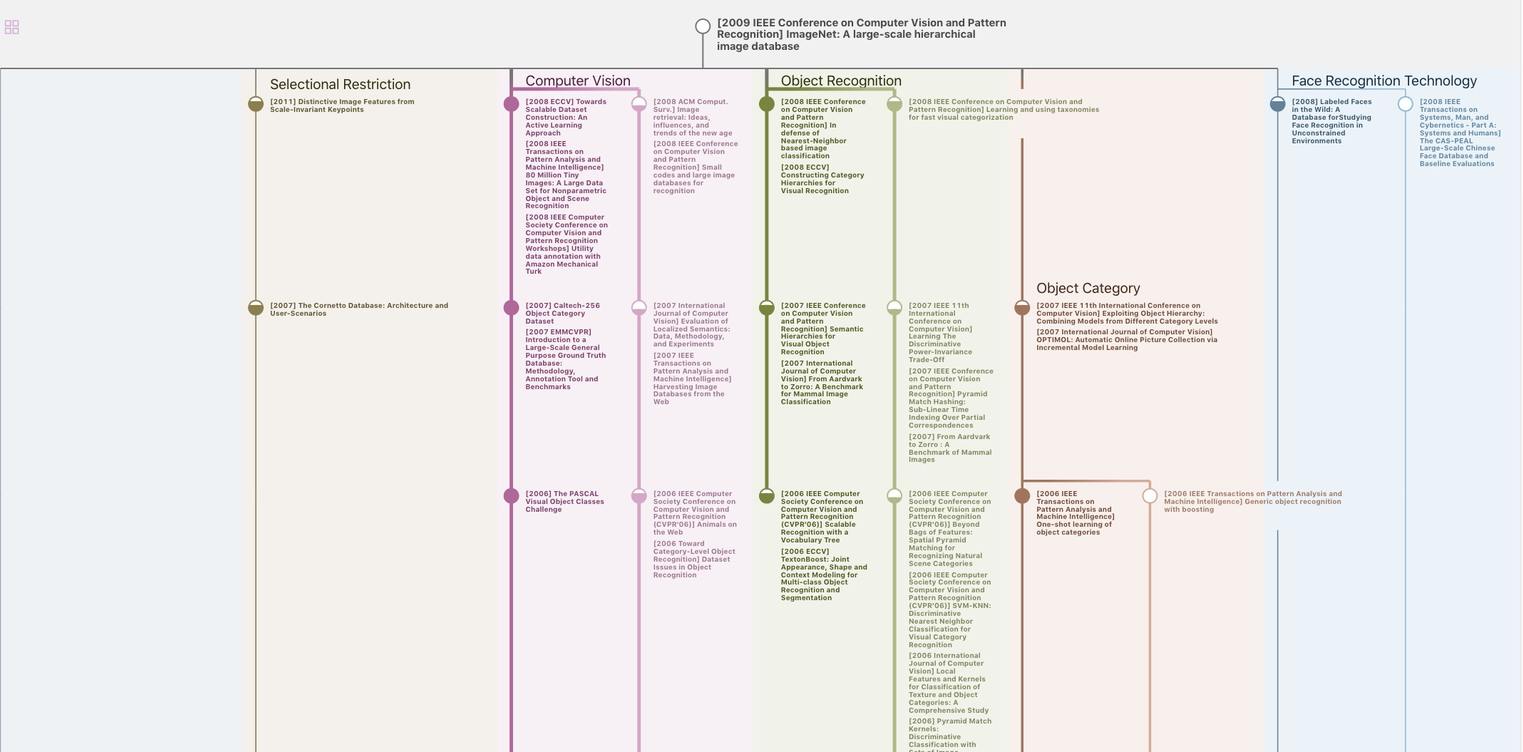
生成溯源树,研究论文发展脉络
Chat Paper
正在生成论文摘要