A Distributed Block Chebyshev-Davidson Algorithm for Parallel Spectral Clustering
Journal of Scientific Computing(2024)
摘要
We develop a distributed Block Chebyshev-Davidson algorithm to solve large-scale leading eigenvalue problems for spectral analysis in spectral clustering. First, the efficiency of the Chebyshev-Davidson algorithm relies on the prior knowledge of the eigenvalue spectrum, which could be expensive to estimate. This issue can be lessened by the analytic spectrum estimation of the Laplacian or normalized Laplacian matrices in spectral clustering, making the proposed algorithm very efficient for spectral clustering. Second, to make the proposed algorithm capable of analyzing big data, a distributed and parallel version has been developed with attractive scalability. The speedup by parallel computing is approximately equivalent to √(p) , where p denotes the number of processes. Numerical results will be provided to demonstrate its efficiency in spectral clustering and scalability advantage over existing eigensolvers used for spectral clustering in parallel computing environments.
更多查看译文
关键词
Sparse symmetric matrices,Parallel computing,Spectral analysis,Spectral clustering
AI 理解论文
溯源树
样例
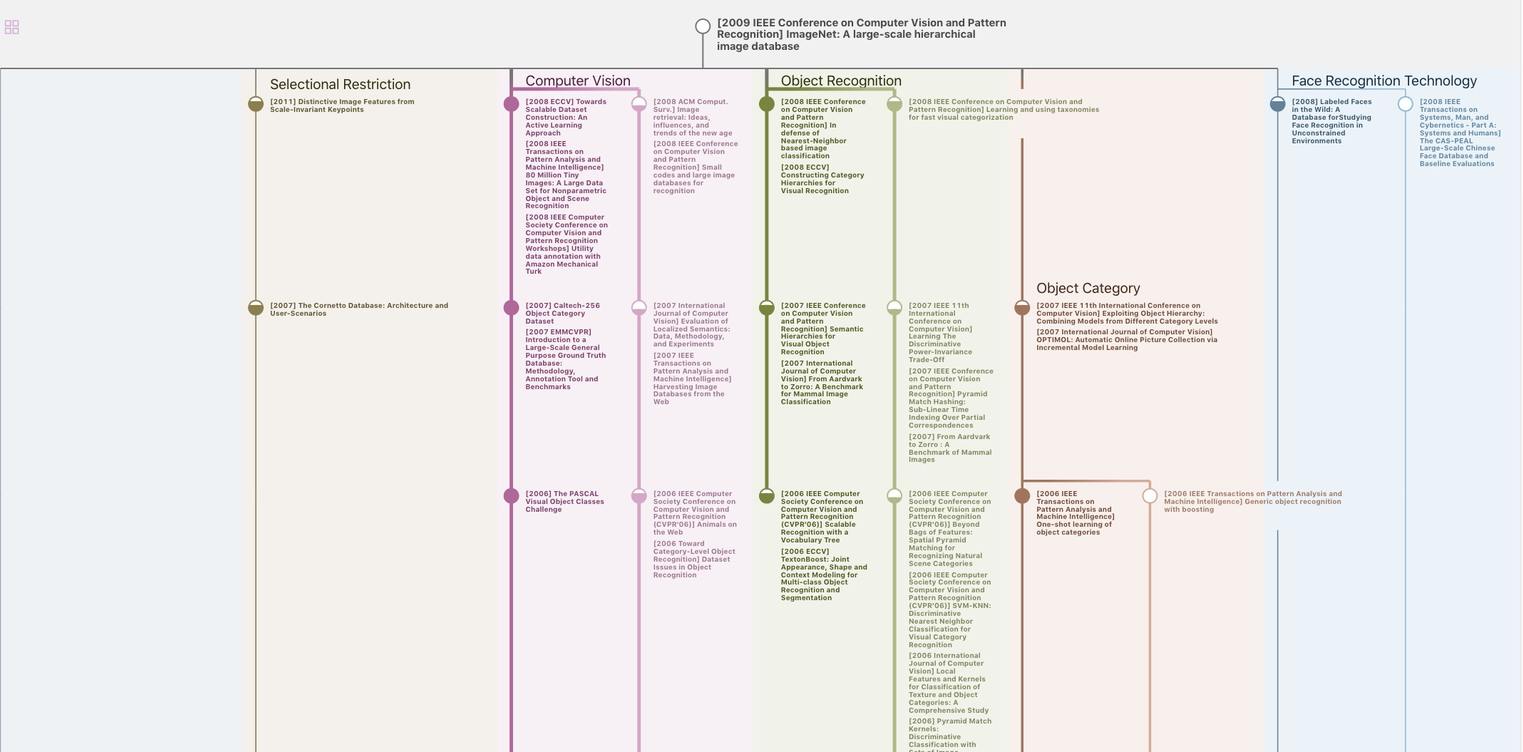
生成溯源树,研究论文发展脉络
Chat Paper
正在生成论文摘要