Real-Time Counterfactual Explanations For Robotic Systems With Multiple Continuous Outputs*
arxiv(2023)
摘要
Although many machine learning methods, especially from the field of deep learning, have been instrumental in addressing challenges within robotic applications, we cannot take full advantage of such methods before these can provide performance and safety guarantees. The lack of trust that impedes the use of these methods mainly stems from a lack of human understanding of what exactly machine learning models have learned, and how robust their behaviour is. This is the problem the field of explainable artificial intelligence aims to solve. Based on insights from the social sciences, we know that humans prefer contrastive explanations, i.e. explanations answering the hypothetical question “what if?”. In this paper, we show that linear model trees are capable of producing answers to such questions, so-called counterfactual explanations, for robotic systems, including in the case of multiple, continuous inputs and outputs. We demonstrate the use of this method to produce counterfactual explanations for two robotic applications. Additionally, we explore the issue of infeasibility, which is of particular interest in systems governed by the laws of physics.
更多查看译文
关键词
Explicability and transparency in Cyber-physical and human systems,Reinforcement learning and deep learning in control,data-driven control,autonomous robotic systems,explainable artificial intelligence for robotics,counterfactual explanations for robotic systems
AI 理解论文
溯源树
样例
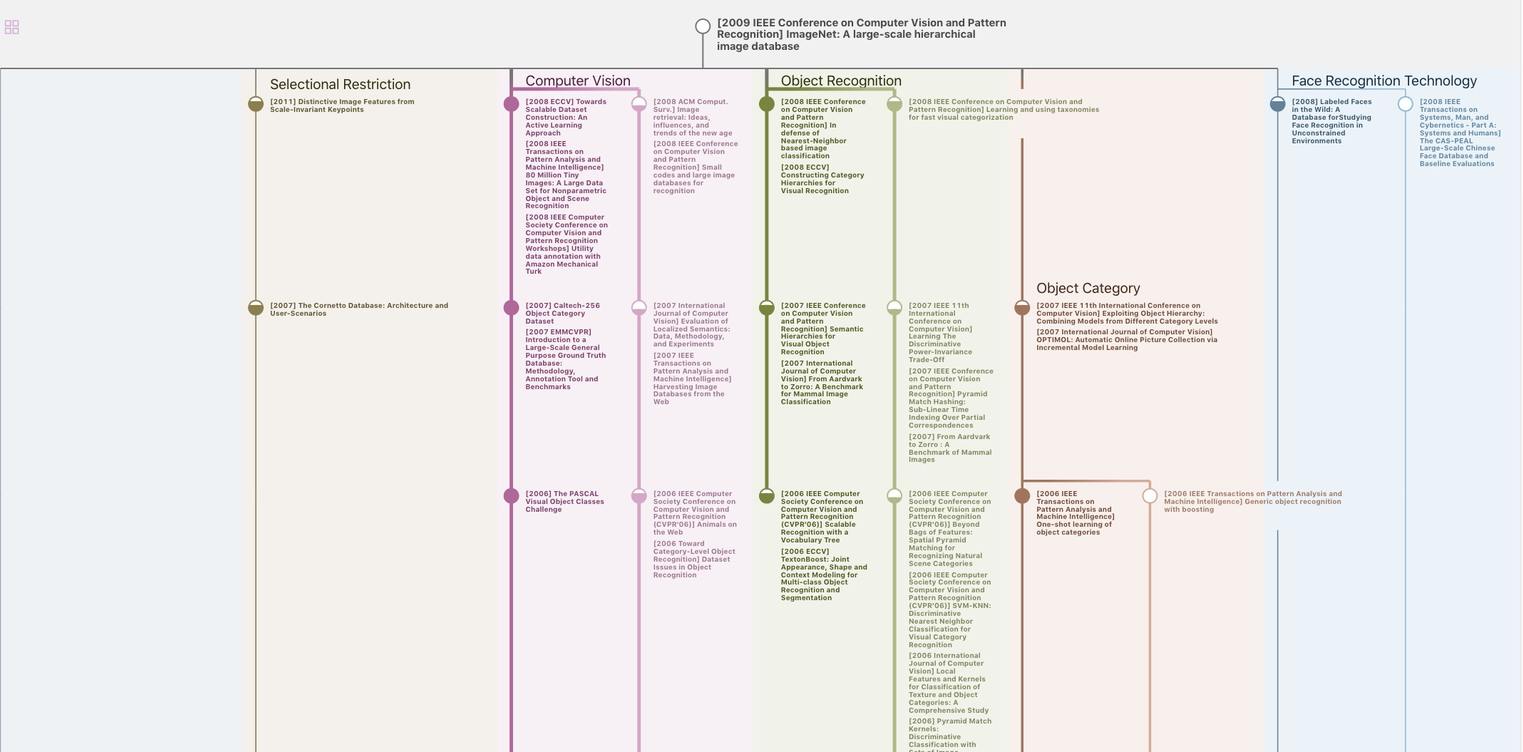
生成溯源树,研究论文发展脉络
Chat Paper
正在生成论文摘要