Progressive Multi-resolution Loss for Crowd Counting
arxiv(2022)
摘要
Crowd counting is usually handled in a density map regression fashion, which is supervised via a L2 loss between the predicted density map and ground truth. To effectively regulate models, various improved L2 loss functions have been proposed to find a better correspondence between predicted density and annotation positions. In this paper, we propose to predict the density map at one resolution but measure the density map at multiple resolutions. By maximizing the posterior probability in such a setting, we obtain a log-formed multi-resolution L2-difference loss, where the traditional single-resolution L2 loss is its particular case. We mathematically prove it is superior to a single-resolution L2 loss. Without bells and whistles, the proposed loss substantially improves several baselines and performs favorably compared to state-of-the-art methods on four crowd counting datasets, ShanghaiTech A & B, UCF-QNRF, and JHU-Crowd++.
更多查看译文
关键词
loss,multi-resolution
AI 理解论文
溯源树
样例
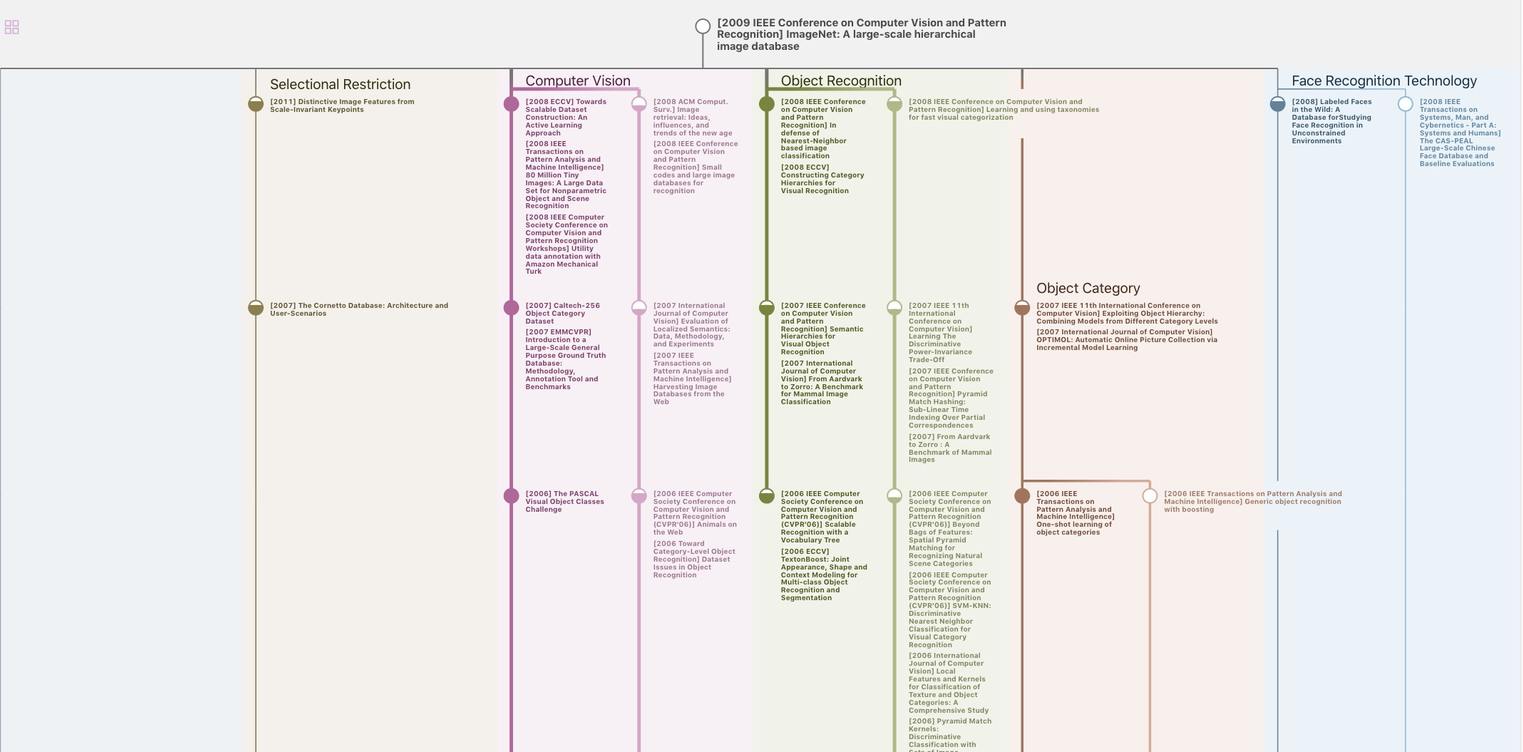
生成溯源树,研究论文发展脉络
Chat Paper
正在生成论文摘要