Learning Action-Effect Dynamics for Hypothetical Vision-Language Reasoning Task
EMNLP (Findings)(2022)
摘要
'Actions' play a vital role in how humans interact with the world. Thus, autonomous agents that would assist us in everyday tasks also require the capability to perform 'Reasoning about Actions & Change' (RAC). This has been an important research direction in Artificial Intelligence (AI) in general, but the study of RAC with visual and linguistic inputs is relatively recent. The CLEVR_HYP (Sampat et. al., 2021) is one such testbed for hypothetical vision-language reasoning with actions as the key focus. In this work, we propose a novel learning strategy that can improve reasoning about the effects of actions. We implement an encoder-decoder architecture to learn the representation of actions as vectors. We combine the aforementioned encoder-decoder architecture with existing modality parsers and a scene graph question answering model to evaluate our proposed system on the CLEVR_HYP dataset. We conduct thorough experiments to demonstrate the effectiveness of our proposed approach and discuss its advantages over previous baselines in terms of performance, data efficiency, and generalization capability.
更多查看译文
关键词
learning,action-effect,vision-language
AI 理解论文
溯源树
样例
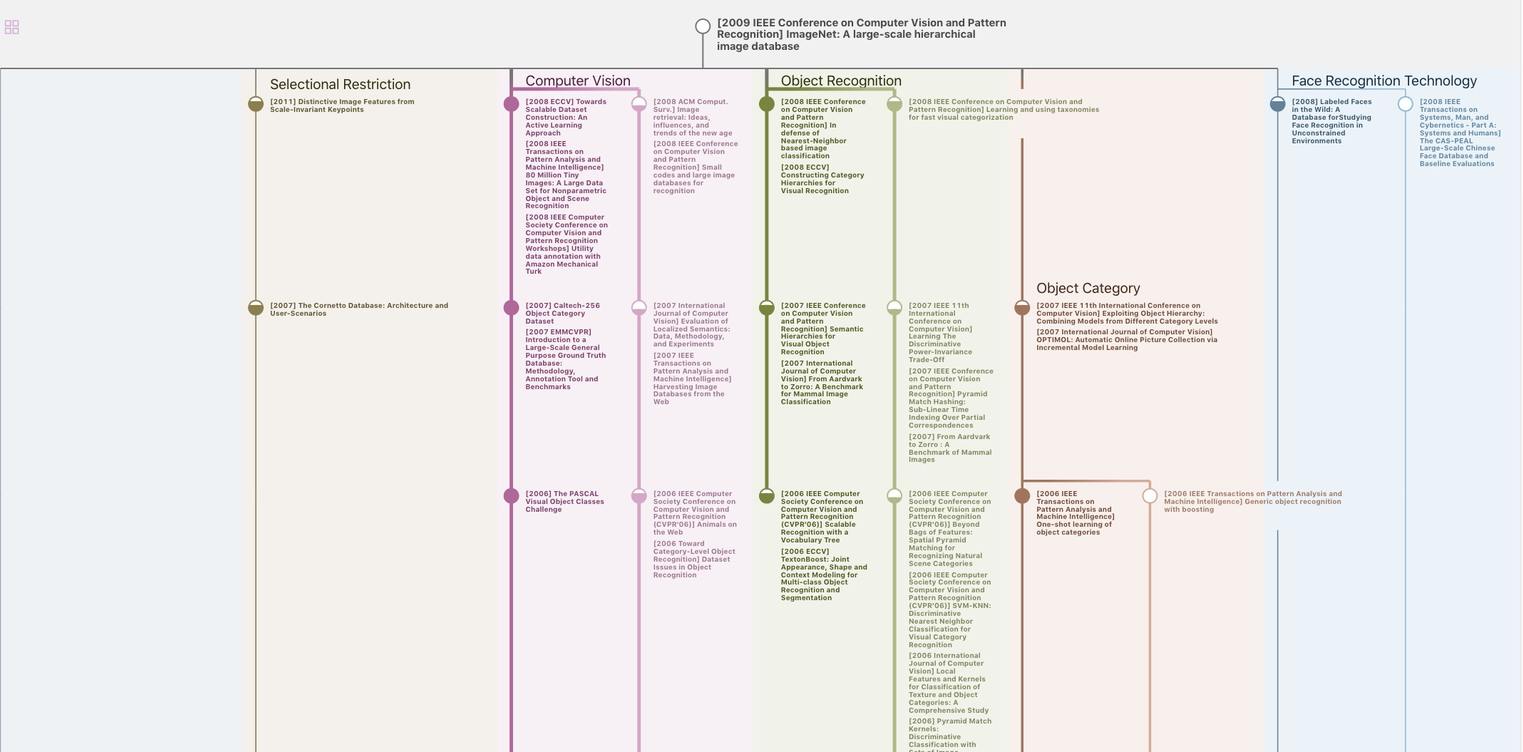
生成溯源树,研究论文发展脉络
Chat Paper
正在生成论文摘要