Detecting multiple retinal diseases in ultra-widefield fundus imaging and data-driven identification of informative regions with deep learning
Nature Machine Intelligence(2022)
摘要
Ultra-widefield (UWF) imaging is a promising modality that captures a larger retinal field of view compared with traditional fundus photography. Previous studies have shown that deep learning models are effective for detecting retinal disease in UWF images, but primarily considered individual diseases under less-than-realistic conditions (excluding images with other diseases, artefacts, comorbidities or borderline cases; and balancing healthy and diseased images) and did not systematically investigate which regions of the UWF images are relevant for disease detection. Here we first improve on the state of the field by proposing a deep learning model that can recognize multiple retinal diseases under more realistic conditions than what has previously been considered. We then use global explainability methods to identify which regions of the UWF images the model generally attends to. Our model performs very well, separating between healthy and diseased retinas with an area under the receiver operating characteristic curve (AUC) of 0.9196 (±0.0001) on an internal test set, and an AUC of 0.9848 (±0.0004) on a challenging, external test set. When diagnosing specific diseases, the model attends to regions where we would expect those diseases to occur. We further identify the posterior pole as the most important region in a purely data-driven fashion. Surprisingly, 10% of the image around the posterior pole is sufficient for achieving comparable performance across all labels to having the full images available.
更多查看译文
关键词
Eye diseases,Machine learning,Medical imaging,Engineering,general
AI 理解论文
溯源树
样例
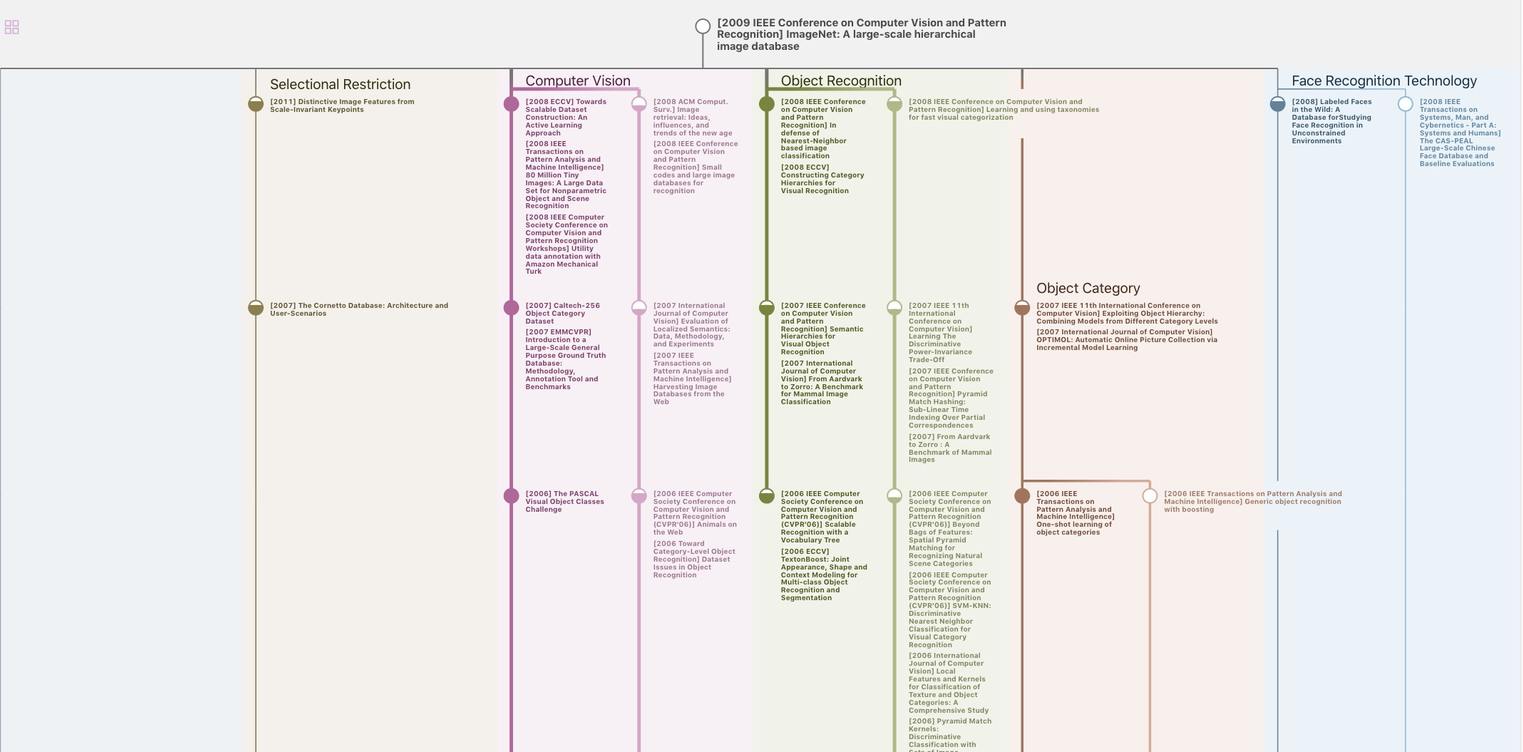
生成溯源树,研究论文发展脉络
Chat Paper
正在生成论文摘要