Fast Learning-Based 3-D Lidar Localization with Multiscale Feature Recursive Matching for Autonomous Driving
2022 International Automatic Control Conference (CACS)(2022)
摘要
Robust localization is crucial for autonomous driving. In this study, we proposed a fast lidar (light detection and ranging) localization architecture that uses the multiscale local features of point clouds to select keypoints in a point cloud frame. We also designed a method called multiscale recursion space compression block (MRSCB) to generate reference points. Next, we used a deep neural network to learn the feature similarity between keypoints and reference points. By calculating the generated point pairs, the model can determine the location of point cloud frames in pre-built point cloud maps. Overall, the design of the MRSCB allowed our model to reduce hardware usage and thus improved the runtime speed. In conclusion, the experimental results obtained with a public autonomous driving data set called KITTI indicated that our method outperformed the five state-of-the-art lidar localization methods in terms of not only localization accuracy but also runtime speed reduction.
更多查看译文
AI 理解论文
溯源树
样例
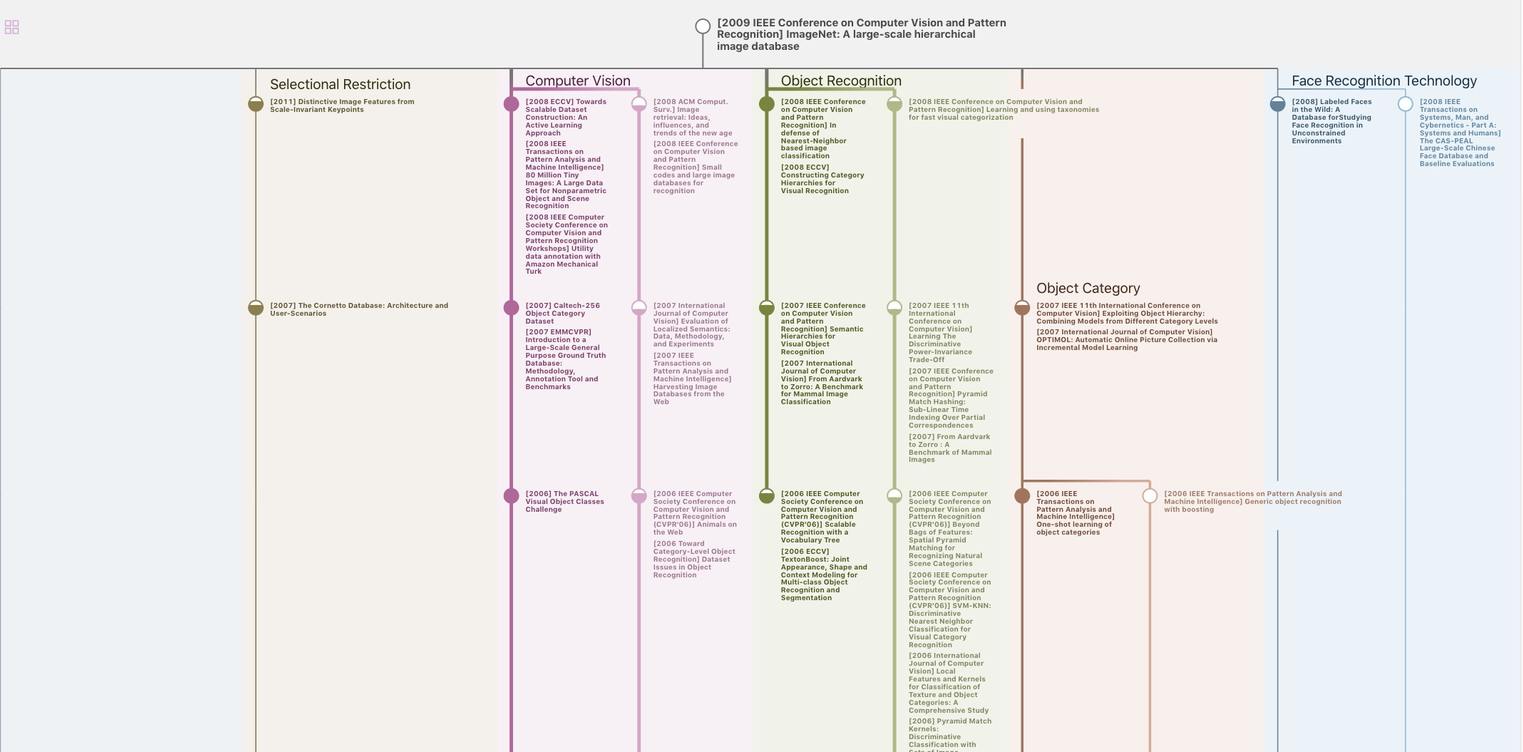
生成溯源树,研究论文发展脉络
Chat Paper
正在生成论文摘要