Radar-Jamming Classification in the Event of Insufficient Samples Using Transfer Learning.
Symmetry(2022)
摘要
Radar has played an irreplaceable role in modern warfare. A variety of radar-jamming methods have been applied in recent years, which makes the electromagnetic environment more complex. The classification of radar jamming is critical for electronic counter-countermeasures (ECCM). In the field of signal classification, machine learning-based methods take great effort to find proper features as well as classifiers, and deep learning-based methods depend on large training datasets. For the above reasons, an efficient transfer learning-based method is proposed in this paper. Firstly, one-dimensional radar signals were transformed into time-frequency images (TFIs) using linear and bilinear time-frequency analysis, which is inspired by symmetry theory. Secondly, pretrained AlexNet and SqueezeNet networks were modified to classify the processed TFIs. Finally, performance of this method was evaluated and compared using a simulated data set with nine types of radar-jamming signals. The results demonstrate that our proposed classification method performs well in accuracy and efficiency at a 1% training ratio, which is practical for anti-jamming.
更多查看译文
关键词
radar jamming,smoothed pseudo-Wigner-Ville distribution,transfer learning,small sample
AI 理解论文
溯源树
样例
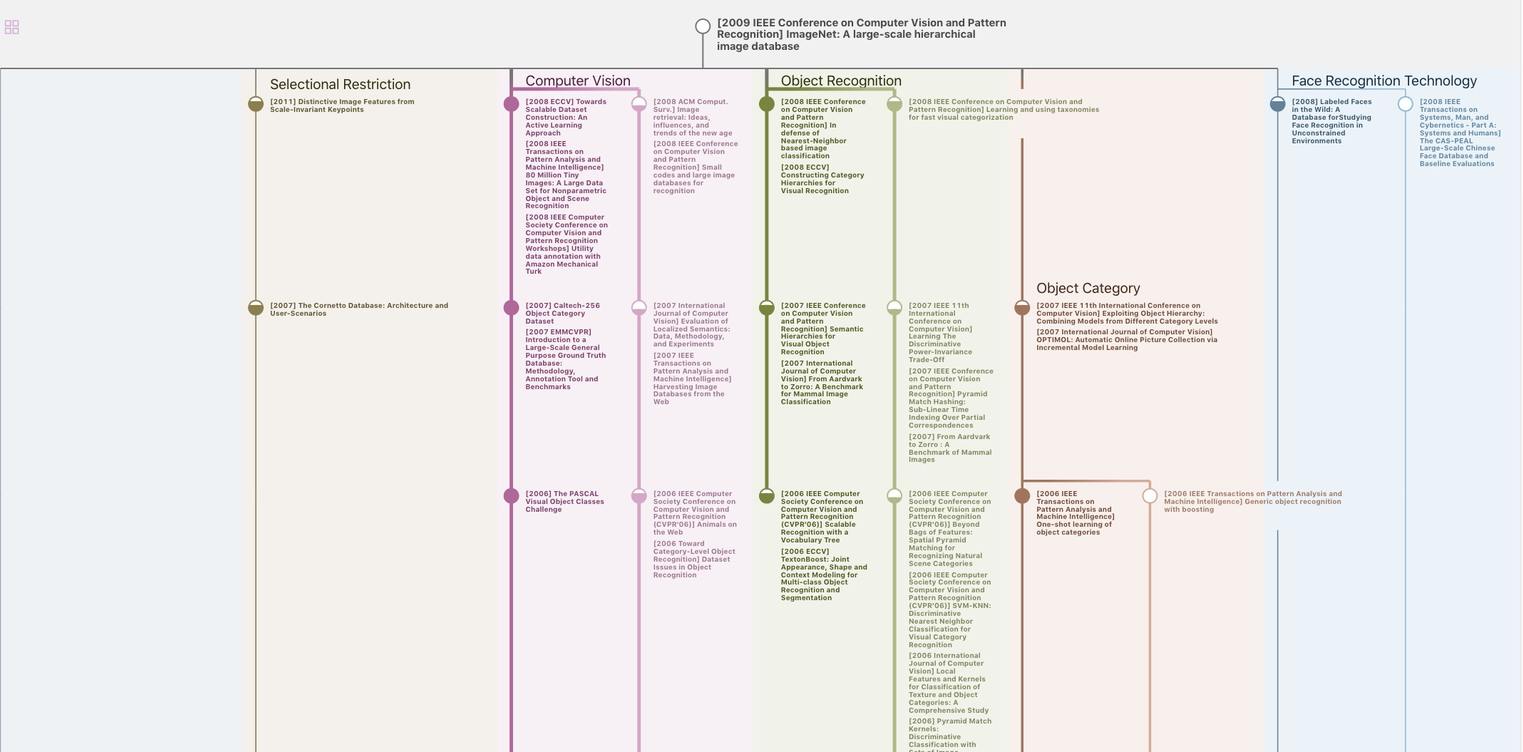
生成溯源树,研究论文发展脉络
Chat Paper
正在生成论文摘要