Comparative Study of Marine Ranching Recognition in Multi-Temporal High-Resolution Remote Sensing Images Based on DeepLab-v3+ and U-Net.
Remote Sensing(2022)
摘要
The recognition and monitoring of marine ranching help to determine the type, spatial distribution and dynamic change of marine life, as well as promote the rational utilization of marine resources and marine ecological environment protection, which has important research significance and application value. In the study of marine ranching recognition based on high-resolution remote sensing images, the convolutional neural network (CNN)-based deep learning method, which can adapt to different water environments, can be used to identify multi-scale targets and restore the boundaries of extraction results with high accuracy. However, research based on deep learning still has problems such as rarely considering the feature complexity of marine ranching and inadequate discussion of model generalization for multi-temporal images. In this paper, we construct a multi-temporal dataset that describes multiple features of marine ranching to explore the recognition and generalization ability of the semantic segmentation models DeepLab-v3+ and U-Net (used in large-area marine ranching) based on GF-1 remote sensing images with a 2 m spatial resolution. Through experiments, we find that the F-score of the U-Net extraction results from multi-temporal test images is basically stable at more than 90%, while the F-score of DeepLab-v3+ fluctuates around 80%. The results show that, compared with DeepLab-v3+, U-Net has a stronger recognition and generalization ability for marine ranching. U-Net can identify floating raft aquaculture areas at different growing stages and distinguish between cage aquaculture areas with different colors but similar morphological characteristics.
更多查看译文
关键词
marine ranching,multi-temporal high-resolution remote sensing images,deep learning,semantic segmentation,DeepLab-v3+,U-Net
AI 理解论文
溯源树
样例
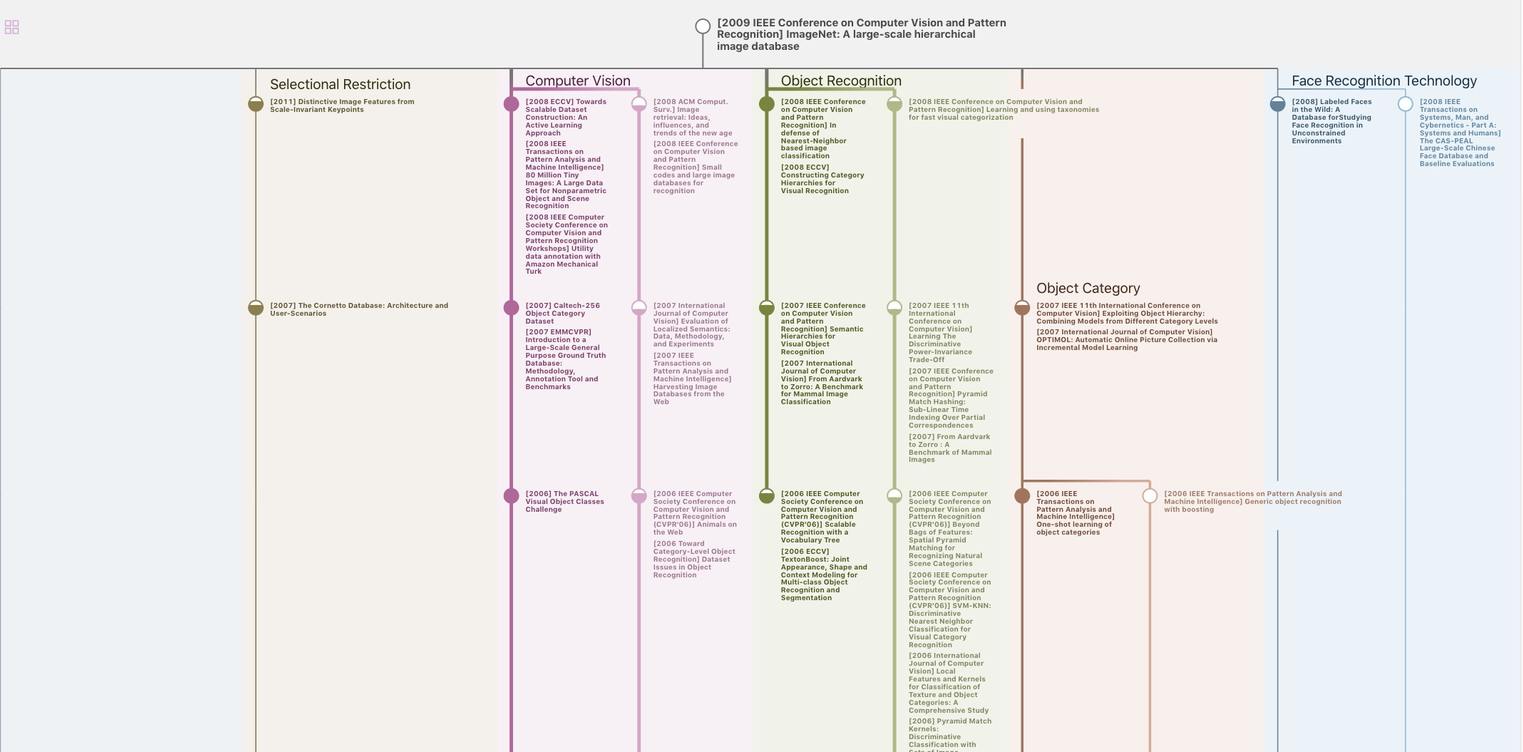
生成溯源树,研究论文发展脉络
Chat Paper
正在生成论文摘要