Countering Physical Eavesdropper Evasion with Adversarial Training.
IEEE Open Journal of the Communications Society(2022)
摘要
Signal classification is a universal problem in adversarial wireless scenarios, especially when an eavesdropping radio receiver attempts to glean information about a target transmitter's patterns, attributes, and contents over a wireless channel. In recent years, research surrounding the idea of Machine Learning (ML)-based signal classification has focused on modulation classification, with the downstream objective of demodulation. However, while the computer vision data domain has made significant progress in ensuring robust classification of images despite crafted perturbations, this success has not been translated to secure modulation classification. In this work, we perform the first-ever physical test of an eavesdropping ML-based modulation classifier radio, which we trained offline using a ensemble of i.i.d. models. Each model is trained with a weighted mixture of data perturbed by iterative, "least likely" white box attacks and non-attacked data. We then tested the ensemble online using coaxial-connected Software Defined Radios (SDRs). We conducted a case study comparing our results to the state-of-the-art computer vision approaches to investigate the presence of "label leaking", model capacity sensitivity, understand the viability of parallel and sequential variations on perturbation training, and assess the effectiveness of iterative attack training. Our results show that perturbations can result in guessing-level classification performance from eavesdroppers, and that varying levels of robustness can be achieved against all presented attacks. These findings confirm that any receiver presents a new attack vector by utilizing ML techniques for classification tasks, and can be vulnerable to evasion attacks at little-to-no cost to transmitters. Consequently, we argue for the use of our training scheme in all ML-based classifying radios where security is a concern.
更多查看译文
关键词
Perturbation methods,Training,Wireless communication,Modulation,Radio transmitters,Computational modeling,Communication system security,Adversarial perturbations,adversarial training,modulation classification,supervised learning,software defined radio
AI 理解论文
溯源树
样例
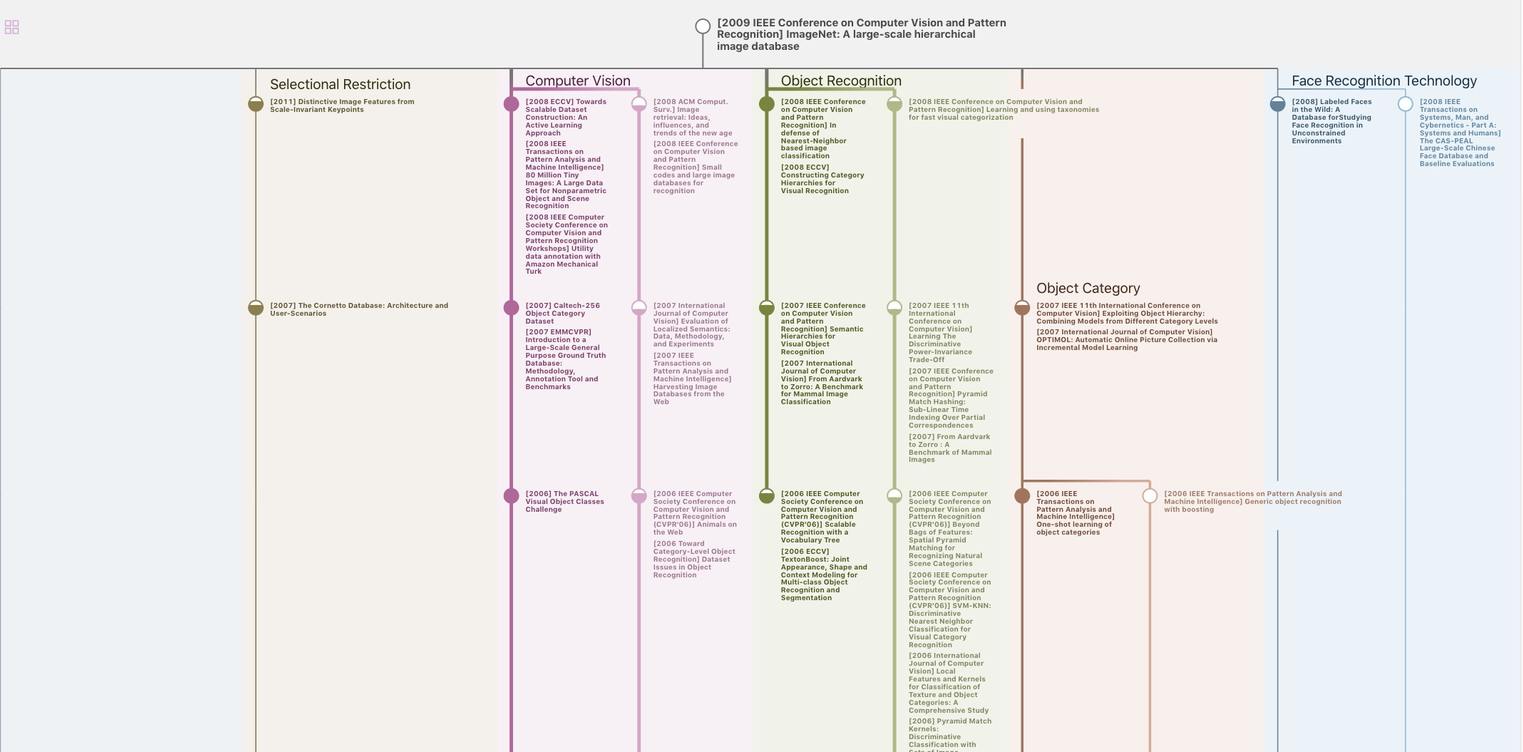
生成溯源树,研究论文发展脉络
Chat Paper
正在生成论文摘要