Robust Camera Pose Estimation for Unordered Road Scene Images in Varying Viewing Conditions
IEEE Transactions on Intelligent Vehicles(2020)
摘要
For continuous performance optimization of camera sensor systems in automated driving, training data from rare corner cases occurring in series production cars are required. In this article, we propose collaborative acquisition of camera images via connected car fleets for synthesis of image sequences from arbitrary road sections which are challenging for machine vision. While allowing a scalable hardware architecture inside the cars, this concept demands to reconstruct the recording locations of the individual images aggregated in the back-end. Varying environmental conditions, dynamic scenes, and small numbers of significant landmarks may hamper camera pose estimation through sparse reconstruction from unordered road scene images. Tackling those problems, we extend a state-of-the-art Structure from Motion pipeline by selecting keypoints based on a semantic image segmentation and removing GPS outliers. We present three challenging image datasets recorded on repetitive test drives under differing environmental conditions for evaluation of our method. The results demonstrate that our optimized pipeline is able to reconstruct the camera viewpoints robustly in the majority of road scenes observed while preserving high image registration rates. Reducing the median deviation from GPS measurements by over 48% for car fleet images, the method increases the accuracy of camera poses dramatically.
更多查看译文
关键词
Cameras,Automobiles,Roads,Image reconstruction,Global Positioning System,Visualization,Vehicle dynamics
AI 理解论文
溯源树
样例
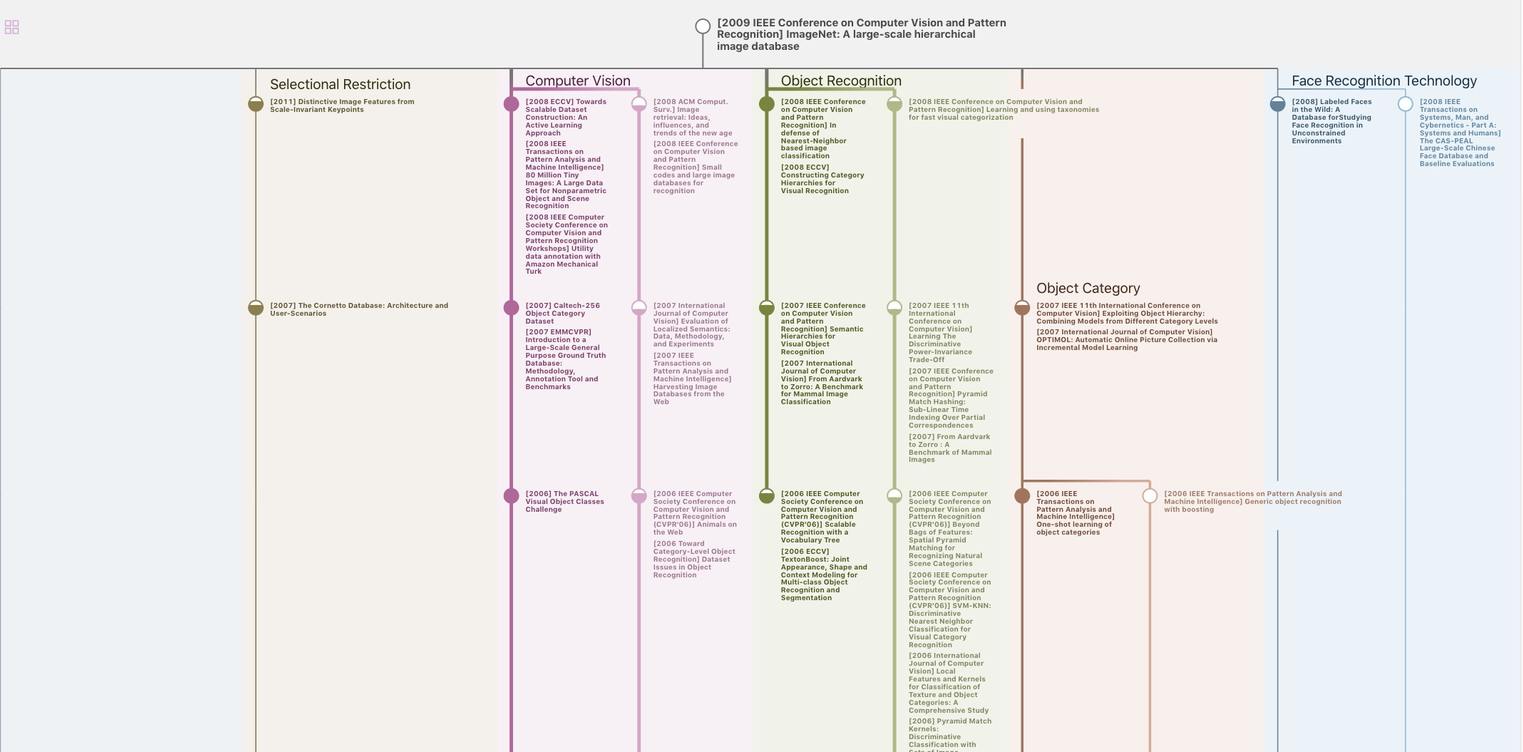
生成溯源树,研究论文发展脉络
Chat Paper
正在生成论文摘要