Distributed, Private, Sparse Histograms in the Two-Server Model
Conference on Computer and Communications Security (CCS)(2022)
摘要
ABSTRACTWe consider the computation of sparse, (ε, ϑ)-differentially private~(DP) histograms in the two-server model of secure multi-party computation~(MPC), which has recently gained traction in the context of privacy-preserving measurements of aggregate user data. We introduce protocols that enable two semi-honest non-colluding servers to compute histograms over the data held by multiple users, while only learning a private view of the data. Our solution achieves the same asymptotic l∞-error of O(log(1/ϑoverε) as in the central model of DP, but without relying on a trusted curator. The server communication and computation costs of our protocol are independent of the number of histogram buckets, and are linear in the number of users, while the client cost is independent of the number of users, ε, and ϑ. Its linear dependence on the number of users lets our protocol scale well, which we confirm using microbenchmarks: for a billion users, ε = 0.5, and ϑ = 10-11, the per-user cost of our protocol is only 1.08 ms of server computation and 339 bytes of communication. In contrast, a baseline protocol using garbled circuits only allows up to 106 users, where it requires 600 KB communication per user.
更多查看译文
关键词
sparse histograms,private,two-server
AI 理解论文
溯源树
样例
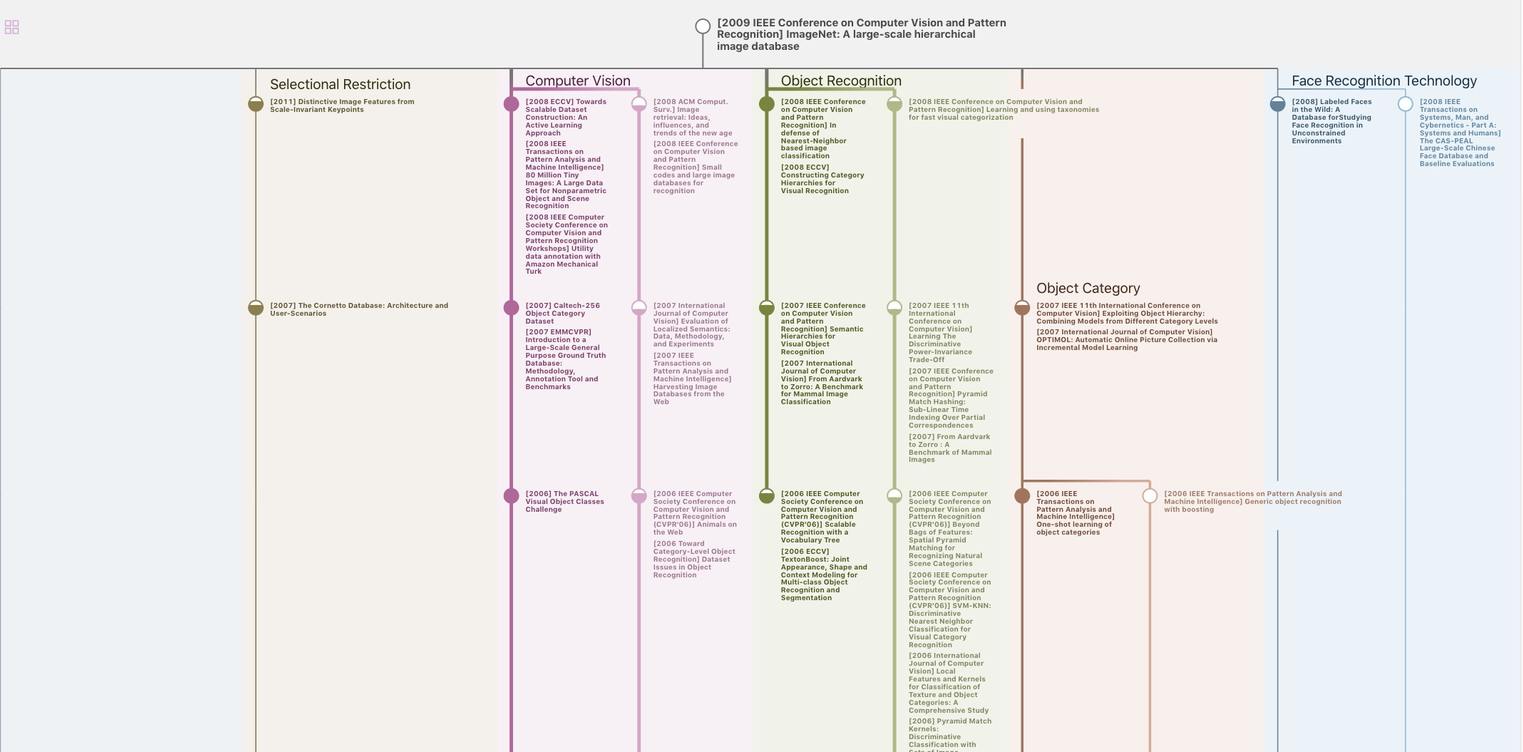
生成溯源树,研究论文发展脉络
Chat Paper
正在生成论文摘要