SI-SA GAN: A Generative Adversarial Network Combined With Spatial Information and Self-Attention for Removing Thin Cloud in Optical Remote Sensing Images.
IEEE Access(2022)
摘要
In agricultural remote sensing monitoring, climate often affects the quality of optical remote sensing image data acquisition. The acquired satellite imagery results usually contain cloud information, leading to a lack of ground data information. Unlike thick clouds, the semi-transparent nature of thin clouds prevents thin clouds from completely obscuring the ground scene. In order to remove thin clouds in the cultivated land and restore the actual ground information as much as possible, we proposed a cloud removal method of spatial information fusion self-attention generative adversarial network (SI-SA GAN) based on multi-directional perceptual attention and self-attention mechanism. The proposed method identifies and focuses on cloud regions using spatial attention, channel attention, and self-attention mechanism, which can enhance image information. The modules of the discriminator utilize residual networks and self-attention non-local neural networks to guide image information output. The generative adversarial network (GAN) is applied to remove clouds and restore the corresponding irregular occlusion area according to the depth characteristics of the input information. A gradient penalty is applied to improve the robustness of the generative network. In this paper, we compared the evaluation indexes of other advanced models. The qualitative and quantitative results of Sentinel-2A and public RICE datasets confirmed that the proposed method could enhance image quality effectively after cloud removal. The model has excellent thin cloud removal performance with small-scale training data.
更多查看译文
关键词
Generative adversarial networks,Spatial resolution,Agriculture,Remote sensing,Feature extraction,Optical sensors,Remote sensing,thin cloud removal,GAN,self-attention,spatial multi-directional perception
AI 理解论文
溯源树
样例
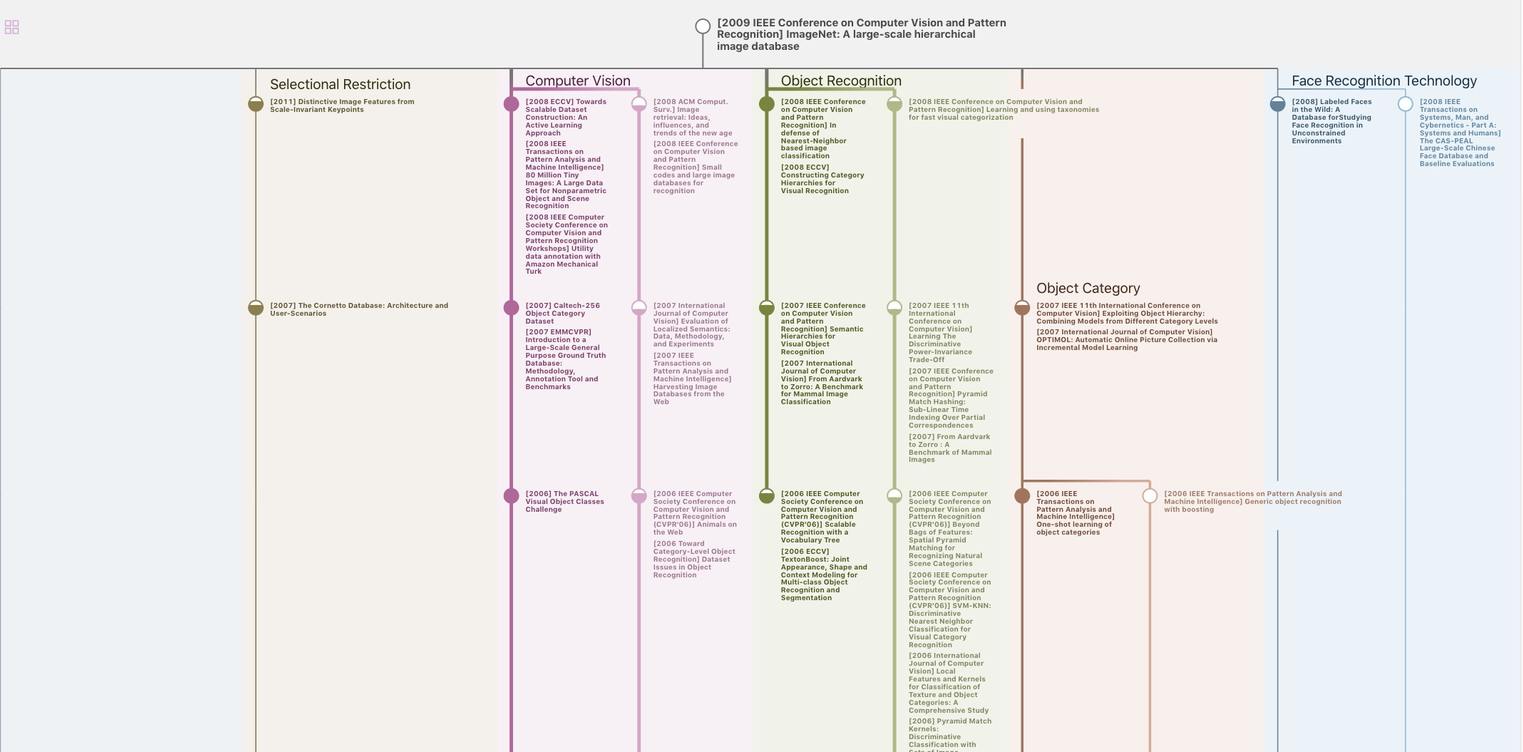
生成溯源树,研究论文发展脉络
Chat Paper
正在生成论文摘要