Discovering Latent Knowledge in Language Models Without Supervision
ICLR 2023(2022)
摘要
Existing techniques for training language models can be misaligned with the truth: if we train models with imitation learning, they may reproduce errors that humans make; if we train them to generate text that humans rate highly, they may output errors that human evaluators can't detect. We propose circumventing this issue by directly finding latent knowledge inside the internal activations of a language model in a purely unsupervised way. Specifically, we introduce a method for accurately answering yes-no questions given only unlabeled model activations. It works by finding a direction in activation space that satisfies logical consistency properties, such as that a statement and its negation have opposite truth values. We show that despite using no supervision and no model outputs, our method can recover diverse knowledge represented in large language models: across 6 models and 10 question-answering datasets, it outperforms zero-shot accuracy by 4\% on average. We also find that it cuts prompt sensitivity in half and continues to maintain high accuracy even when models are prompted to generate incorrect answers. Our results provide an initial step toward discovering what language models know, distinct from what they say, even when we don't have access to explicit ground truth labels.
更多查看译文
关键词
AI safety,AI alignment,truthfulness,large language models,honesty,interpretability
AI 理解论文
溯源树
样例
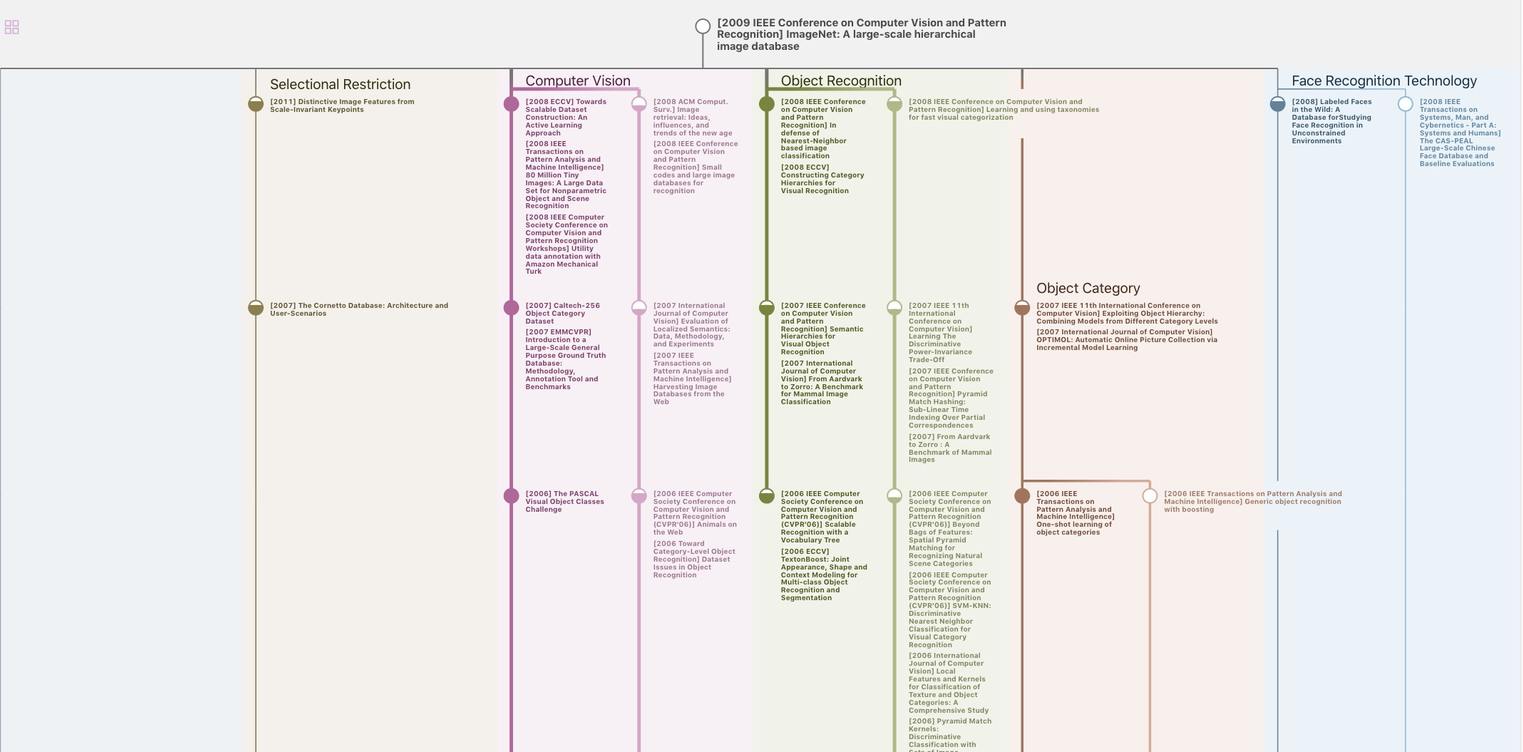
生成溯源树,研究论文发展脉络
Chat Paper
正在生成论文摘要