Gait Phase Estimation of Unsupervised Outdoors Walking Using IMUs and a Linear Regression Model
IEEE ACCESS(2022)
摘要
Human gait analysis and detection are critical for many applications, including wearable and rehabilitation robotic devices, reducing or tracking injury risk. The proposed work allows researchers to study the gait phase of human subjects in an unsupervised outdoor environment without the need for fixed thresholds and sensor-embedded insoles. We present an experimental protocol to label gait events based on patterns in human subjects from two body-worn inertial measurement units (IMUs). Gait patterns are developed using a force plate and a motion capture system. Upon defining the gait pattern, human subjects walk outdoors for forty minutes to train and test a principal component analysis (PCA)-based linear regression model. Next, gait phase estimation is performed using the defined patterns from other human subjects to accommodate cases where motion capture and force plate data are unavailable. Results showed a minimum normalized gait phase estimation error of 1.81 %, a maximum of 2.48 %, and an average of 2.21 +/- 0.258 % for all subjects involved. Results are particularly significant because the proposed work can be expanded to precise control of human-assistive devices, rehabilitation devices, and clinical gait analysis.
更多查看译文
关键词
Biomechanics,gait analysis,heel strike,PCA,machine learning,prosthesis,IMUs,motion capture,linear regression
AI 理解论文
溯源树
样例
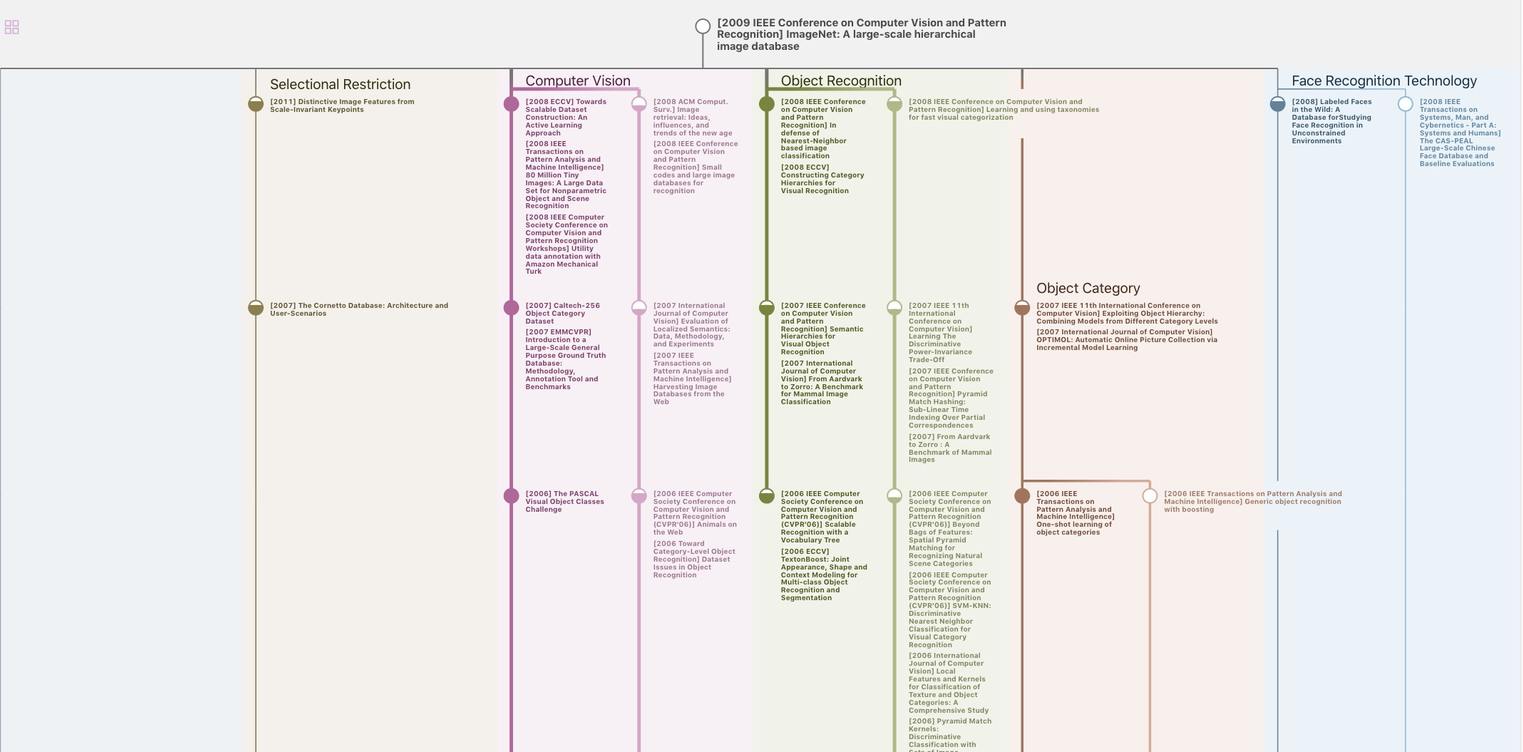
生成溯源树,研究论文发展脉络
Chat Paper
正在生成论文摘要