A Strongly Polynomial Algorithm for Approximate Forster Transforms and Its Application to Halfspace Learning
PROCEEDINGS OF THE 55TH ANNUAL ACM SYMPOSIUM ON THEORY OF COMPUTING, STOC 2023(2023)
摘要
The Forster transform is a method of regularizing a dataset by placing it in radial isotropic position while maintaining some of its essential properties. Forster transforms have played a key role in a diverse range of settings spanning computer science and functional analysis. Prior work had given weakly polynomial time algorithms for computing Forster transforms, when they exist. Our main result is the first strongly polynomial time algorithm to compute an approximate Forster transform of a given dataset or certify that no such transformation exists. By leveraging our strongly polynomial Forster algorithm, we obtain the first strongly polynomial time algorithm for distribution-free PAC learning of halfspaces. This learning result is surprising because proper PAC learning of halfspaces is equivalent to linear programming. Our learning approach extends to give a strongly polynomial halfspace learner in the presence of random classification noise and, more generally, Massart noise.
更多查看译文
关键词
PAC learning,Halfspaces,Massart Noise
AI 理解论文
溯源树
样例
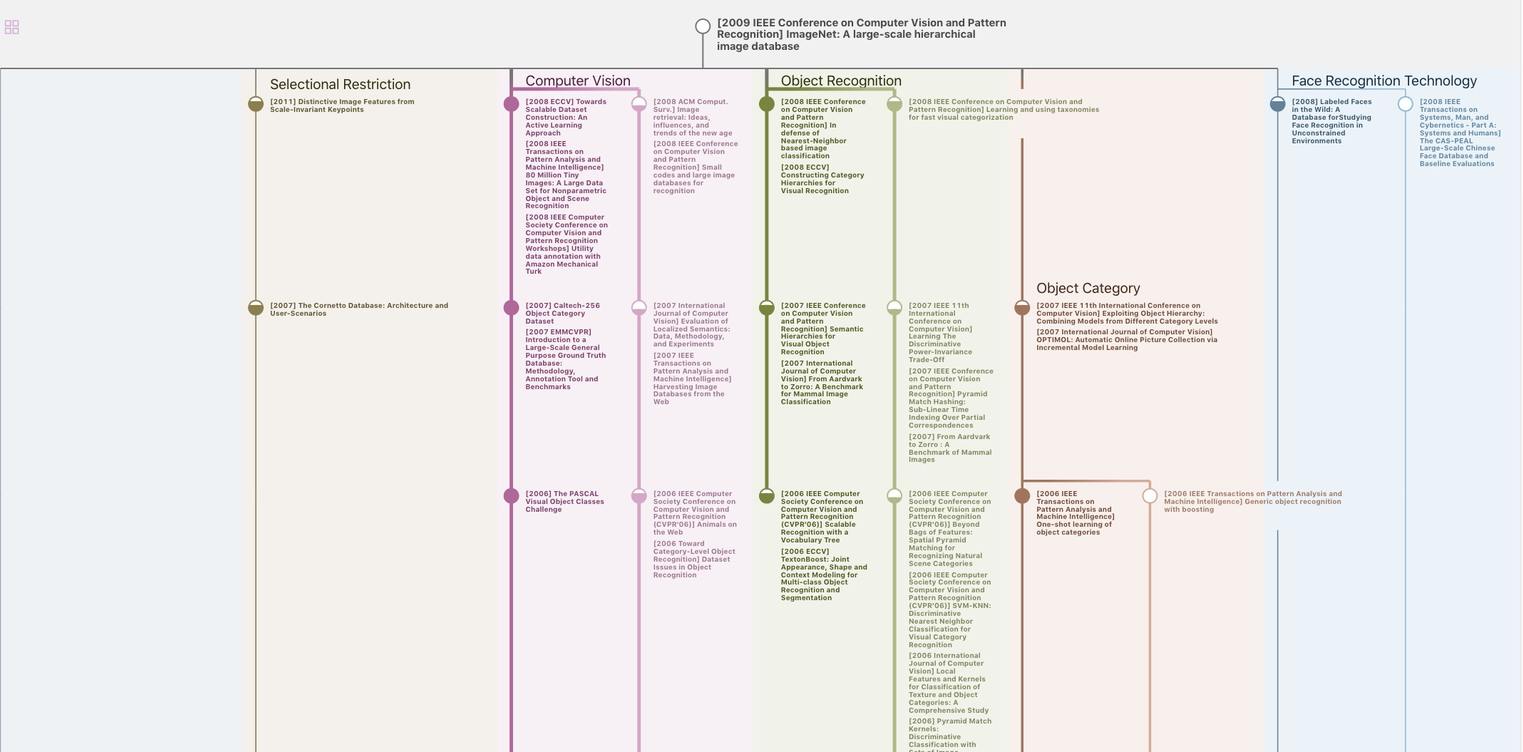
生成溯源树,研究论文发展脉络
Chat Paper
正在生成论文摘要