Solving Rearrangement Puzzles using Path Defragmentation in Factored State Spaces
arxiv(2022)
摘要
Rearrangement puzzles are variations of rearrangement problems in which the elements of a problem are potentially logically linked together. To efficiently solve such puzzles, we develop a motion planning approach based on a new state space that is logically factored, integrating the capabilities of the robot through factors of simultaneously manipulatable joints of an object. Based on this factored state space, we propose less-actions RRT (LA-RRT), a planner which optimizes for a low number of actions to solve a puzzle. At the core of our approach lies a new path defragmentation method, which rearranges and optimizes consecutive edges to minimize action cost. We solve six rearrangement scenarios with a Fetch robot, involving planar table puzzles and an escape room scenario. LA-RRT significantly outperforms the next best asymptotically-optimal planner by 4.01 to 6.58 times improvement in final action cost.
更多查看译文
关键词
Constrained motion planning, manipulation planning, task and motion planning
AI 理解论文
溯源树
样例
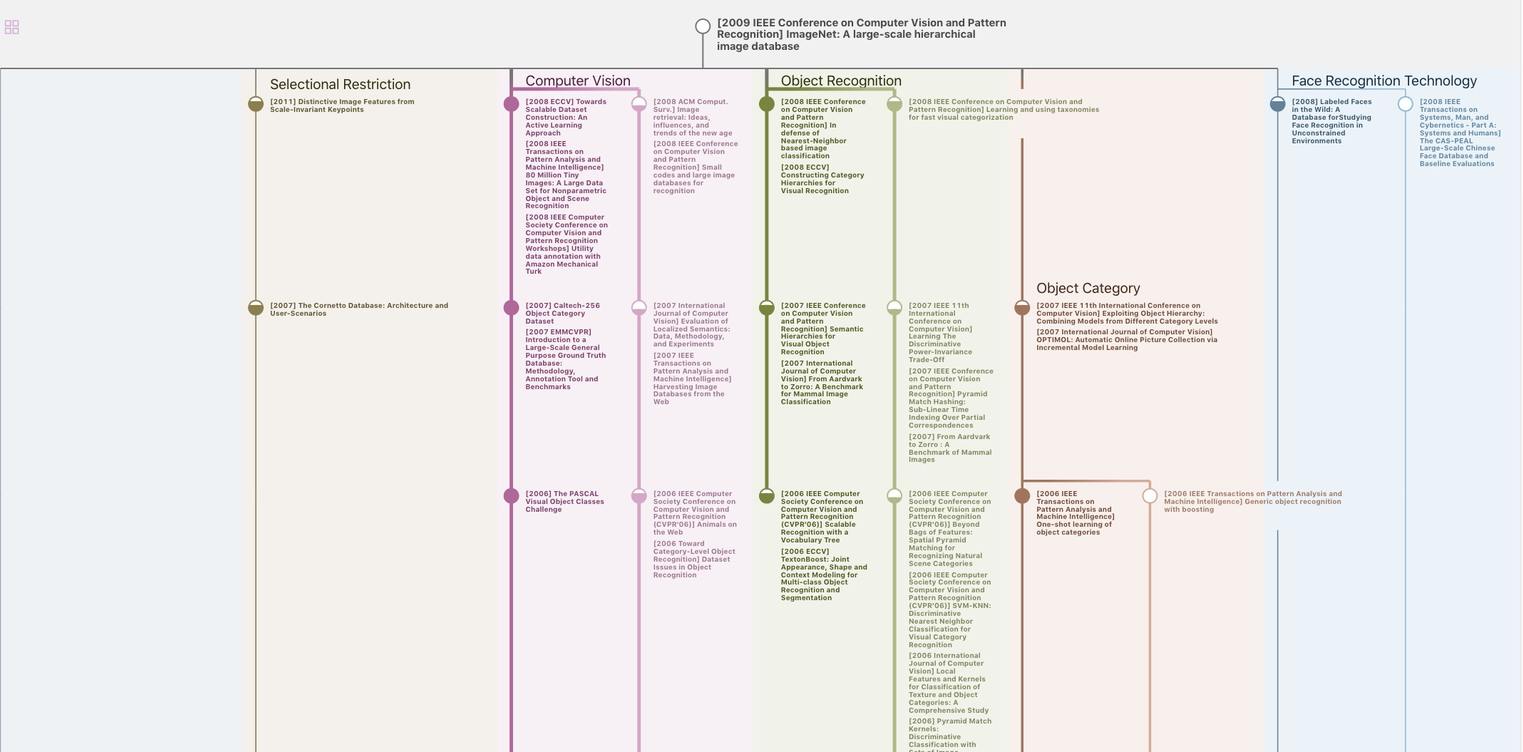
生成溯源树,研究论文发展脉络
Chat Paper
正在生成论文摘要