Self-Supervised Audio-Visual Speech Representations Learning By Multimodal Self-Distillation
arxiv(2022)
摘要
In this work, we present a novel method, named AV2vec, for learning audio-visual speech representations by multimodal self-distillation. AV2vec has a student and a teacher module, in which the student performs a masked latent feature regression task using the multimodal target features generated online by the teacher. The parameters of the teacher model are a momentum update of the student. Since our target features are generated online, AV2vec needs no iteration step like AV-HuBERT and the total training time cost is reduced to less than one-fifth. We further propose AV2vec-MLM in this study, which augments AV2vec with a masked language model (MLM)-style loss using multitask learning. Our experimental results show that AV2vec achieved comparable performance to the AV-HuBERT baseline. When combined with an MLM-style loss, AV2vec-MLM outperformed baselines and achieved the best performance on the downstream tasks.
更多查看译文
关键词
self-supervised learning,audio-visual speech recognition,lipreading
AI 理解论文
溯源树
样例
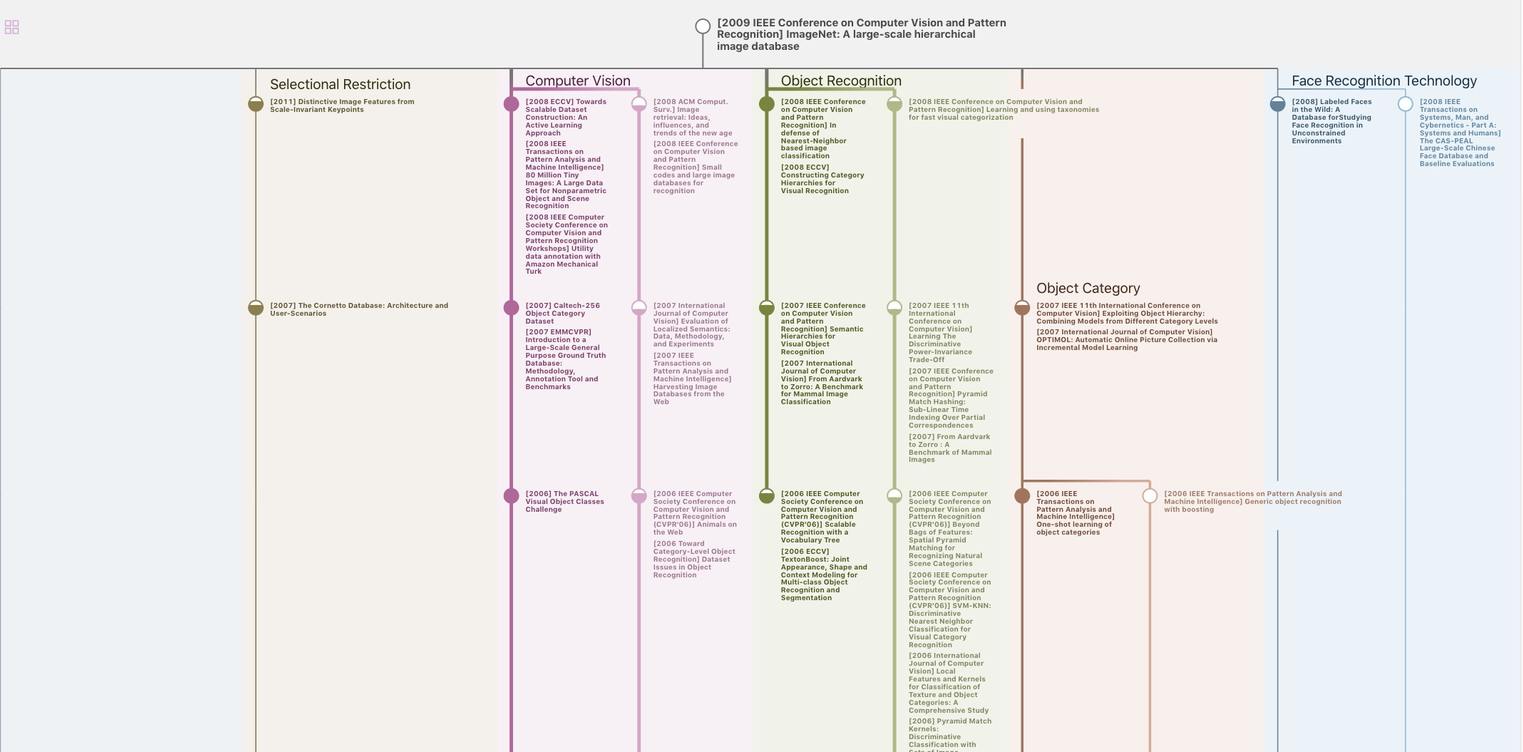
生成溯源树,研究论文发展脉络
Chat Paper
正在生成论文摘要