Efficient Malware Analysis Using Metric Embeddings
arxiv(2022)
摘要
In this paper, we explore the use of metric learning to embed Windows PE files in a low-dimensional vector space for downstream use in a variety of applications, including malware detection, family classification, and malware attribute tagging. Specifically, we enrich labeling on malicious and benign PE files using computationally expensive, disassembly-based malicious capabilities. Using these capabilities, we derive several different types of metric embeddings utilizing an embedding neural network trained via contrastive loss, Spearman rank correlation, and combinations thereof. We then examine performance on a variety of transfer tasks performed on the EMBER and SOREL datasets, demonstrating that for several tasks, low-dimensional, computationally efficient metric embeddings maintain performance with little decay, which offers the potential to quickly retrain for a variety of transfer tasks at significantly reduced storage overhead. We conclude with an examination of practical considerations for the use of our proposed embedding approach, such as robustness to adversarial evasion and introduction of task-specific auxiliary objectives to improve performance on mission critical tasks.
更多查看译文
关键词
efficient malware analysis
AI 理解论文
溯源树
样例
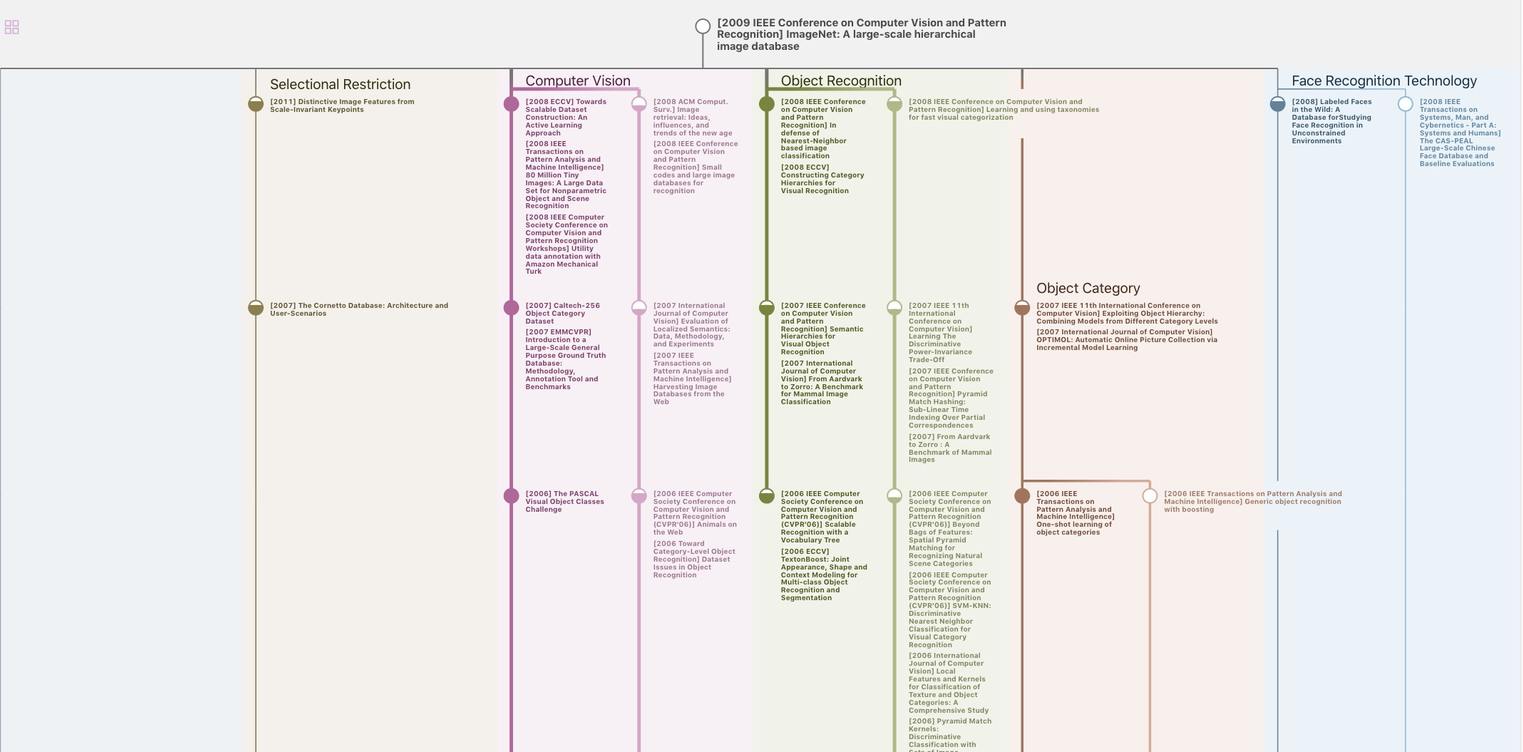
生成溯源树,研究论文发展脉络
Chat Paper
正在生成论文摘要