Translating Big Data to Clinical Outcomes in Anxiety: Potential for Multimodal Integration
Current psychiatry reports(2022)
摘要
Purpose of the Review This review describes approaches to research on anxiety that attempt to link neural correlates to treatment response and novel therapies. The review emphasizes pediatric anxiety disorders since most anxiety disorders begin before adulthood. Recent Findings Recent literature illustrates how current treatments for anxiety manifest diverse relations with a range of neural markers. While some studies demonstrate post-treatment normalization of markers in anxious individuals, others find persistence of group differences. For other markers, which show no pretreatment association with anxiety, the markers nevertheless distinguish treatment-responders from non-responders. Heightened error related negativity represents the risk marker discussed in the most depth; however, limitations in measures related to error responding necessitate multimodal and big-data approaches. Summary Single risk markers show limits as correlates of treatment response. Large-scale, multimodal data analyzed with predictive models may illuminate additional risk markers related to anxiety disorder treatment outcomes. Such work may identify novel targets and eventually guide improvements in treatment response/outcomes.
更多查看译文
关键词
Anxiety,Treatment,Big data,MRI,Neuroimaging,ERN
AI 理解论文
溯源树
样例
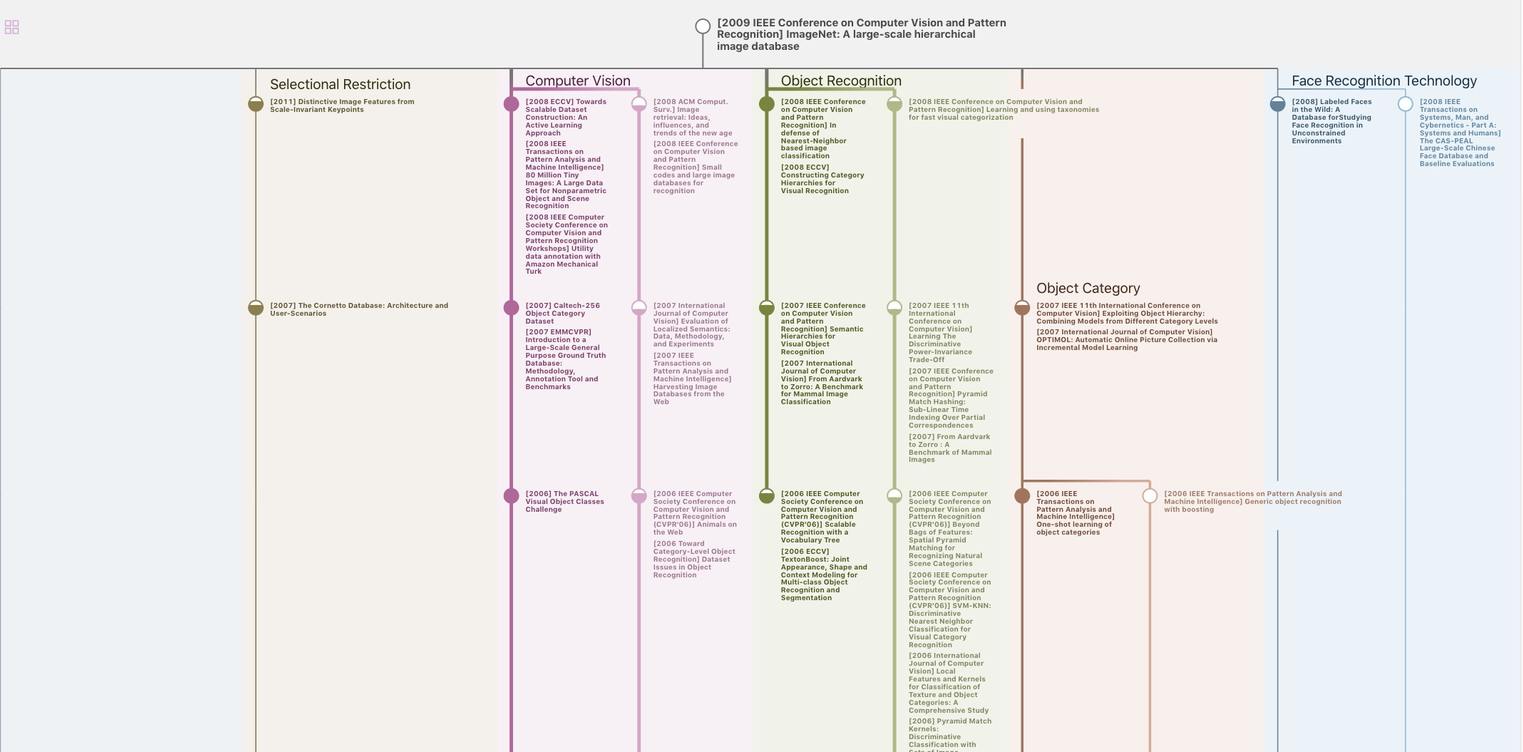
生成溯源树,研究论文发展脉络
Chat Paper
正在生成论文摘要