Optimization of Surrogate function using Extremum Seeking Control
2022 Australian & New Zealand Control Conference (ANZCC)(2022)
摘要
Real-time robust optimization of unknown expensive functions has been a challenging problem for data-driven based control applications. Most of the model-free control applications use function approximation technique based on measurements which makes it independent of the mathematical model. In surrogate optimization, the function is approximated using Gaussian Process Regression (GPR) which is a non-parametric approach. GPR based surrogate function makes the system robust by incorporating variance around the mean value, making the system tolerant against disturbances and noise. To make the optimization problem completely model-free, a zeroth-order gradient estimator is used to optimize the objective function. Extremum Seeking Control (ESC) is gradient-free technique that estimates the gradient by incorporating perturbation signals that drive the optimizer towards the optimal value. Using extremum seeking control, this paper provides a model-free, real-time, and robust optimization technique for optimising the surrogate function.
更多查看译文
关键词
Extremum Seeking Control (ESC),Gaussian Process Regression (GPR),Optimization,Surrogate Optimization (SO)
AI 理解论文
溯源树
样例
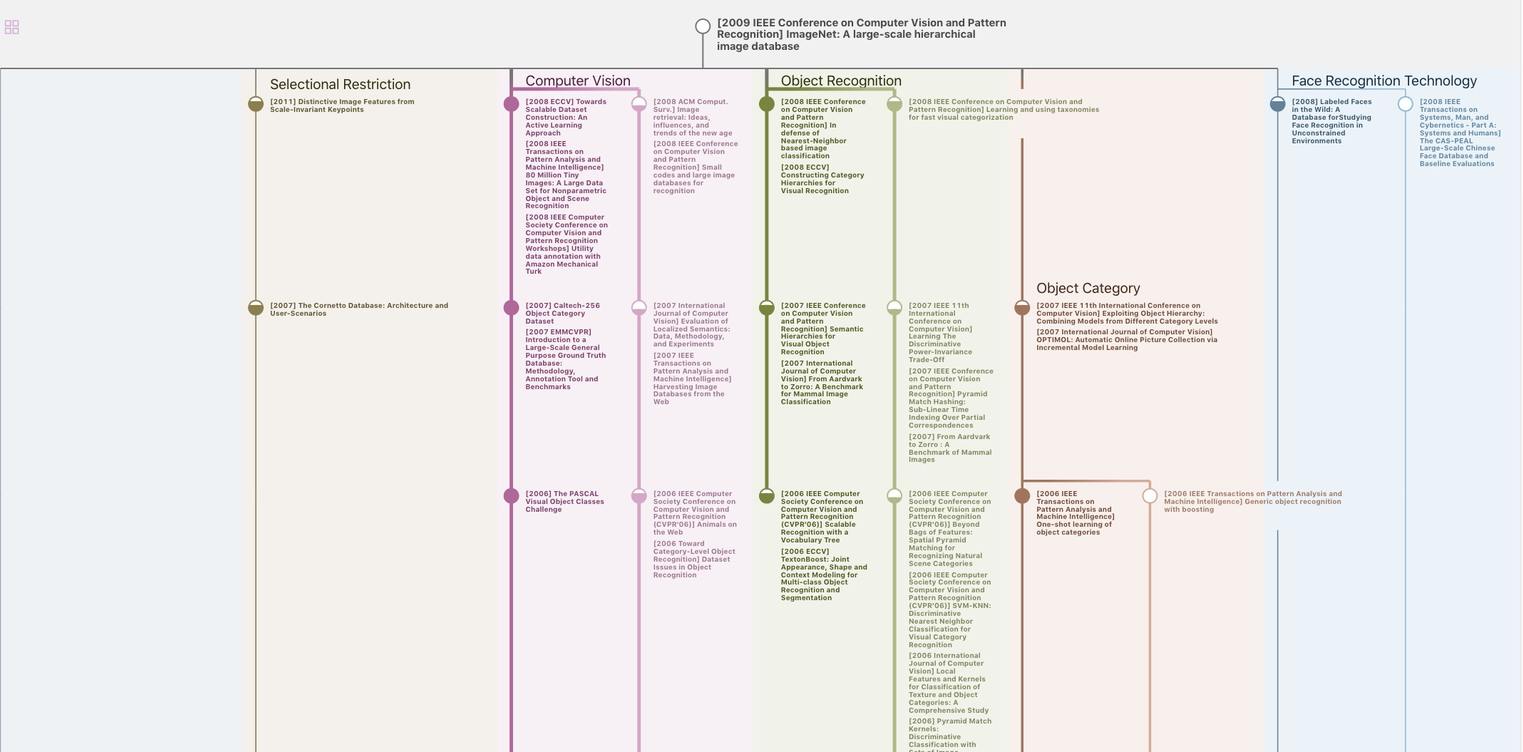
生成溯源树,研究论文发展脉络
Chat Paper
正在生成论文摘要