Rapid online plant leaf area change detection with high-throughput plant image data
JOURNAL OF APPLIED STATISTICS(2023)
摘要
High-throughput plant phenotyping (HTPP) has become an emerging technique to study plant traits due to its fast, labor-saving, accurate and non-destructive nature. It has wide applications in plant breeding and crop management. However, the resulting massive image data has raised a challenge associated with efficient plant traits prediction and anomaly detection. In this paper, we propose a two-step image-based online detection framework for monitoring and quick change detection of the individual plant leaf area via real-time imaging data. Our proposed method is able to achieve a smaller detection delay compared with some baseline methods under some predefined false alarm rate constraint. Moreover, it does not need to store all past image information and can be implemented in real time. The efficiency of the proposed framework is validated by a real data analysis.
更多查看译文
关键词
Supervised learning,ADMM algorithm,online detection,adaptive cusum,plant leaf area,high-throughput plant phenotyping (HTPP)
AI 理解论文
溯源树
样例
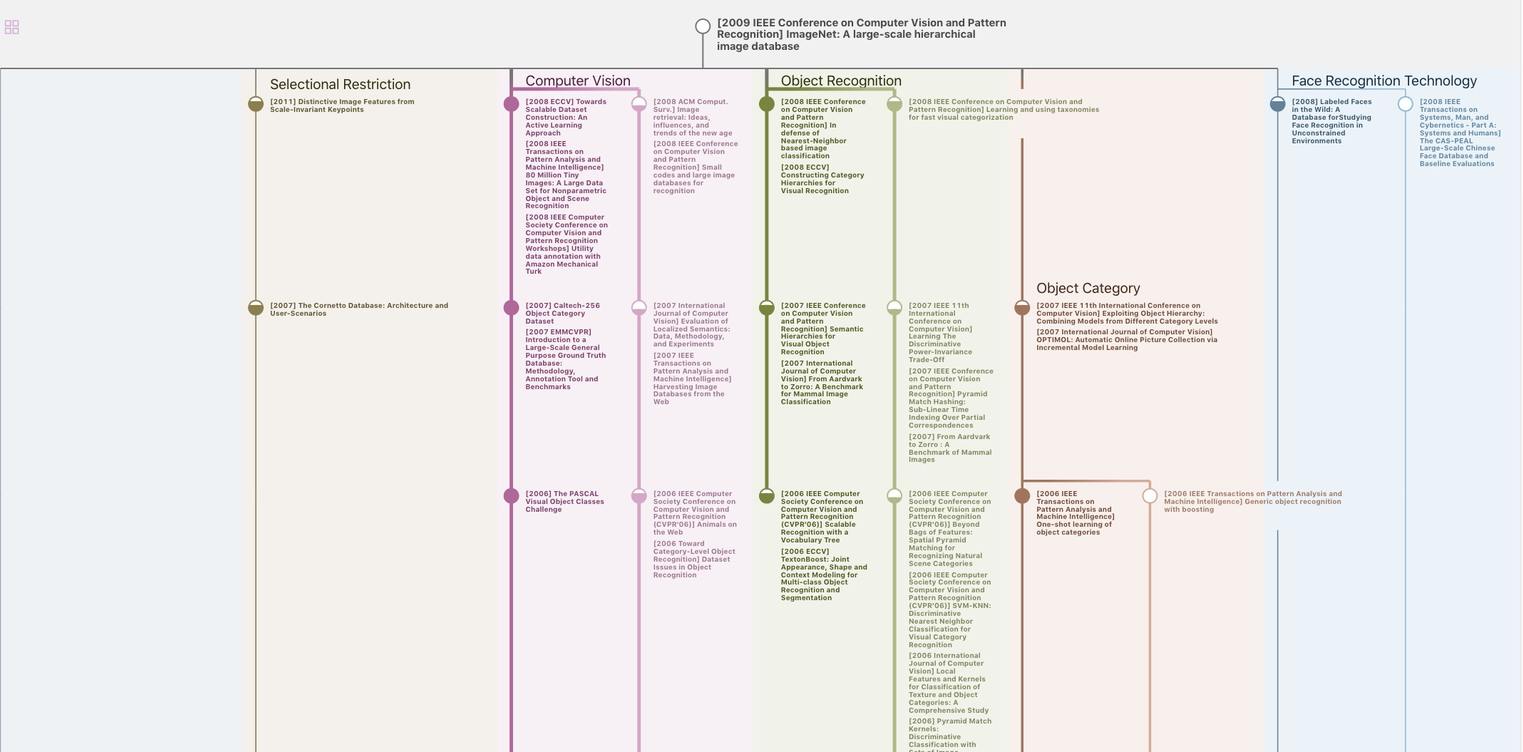
生成溯源树,研究论文发展脉络
Chat Paper
正在生成论文摘要