An Efficient Approach to Large-Scale Ab Initio Conformational Energy Profiles of Small Molecules
MOLECULES(2022)
摘要
Accurate conformational energetics of molecules are of great significance to understand maby chemical properties. They are also fundamental for high-quality parameterization of force fields. Traditionally, accurate conformational profiles are obtained with density functional theory (DFT) methods. However, obtaining a reliable energy profile can be time-consuming when the molecular sizes are relatively large or when there are many molecules of interest. Furthermore, incorporation of data-driven deep learning methods into force field development has great requirements for high-quality geometry and energy data. To this end, we compared several possible alternatives to the traditional DFT methods for conformational scans, including the semi-empirical method GFN2-xTB and the neural network potential ANI-2x. It was found that a sequential protocol of geometry optimization with the semi-empirical method and single-point energy calculation with high-level DFT methods can provide satisfactory conformational energy profiles hundreds of times faster in terms of optimization.
更多查看译文
关键词
conformational energy profile,computational efficiency,semi-empirical method,neural network potential,AMOEBA force field
AI 理解论文
溯源树
样例
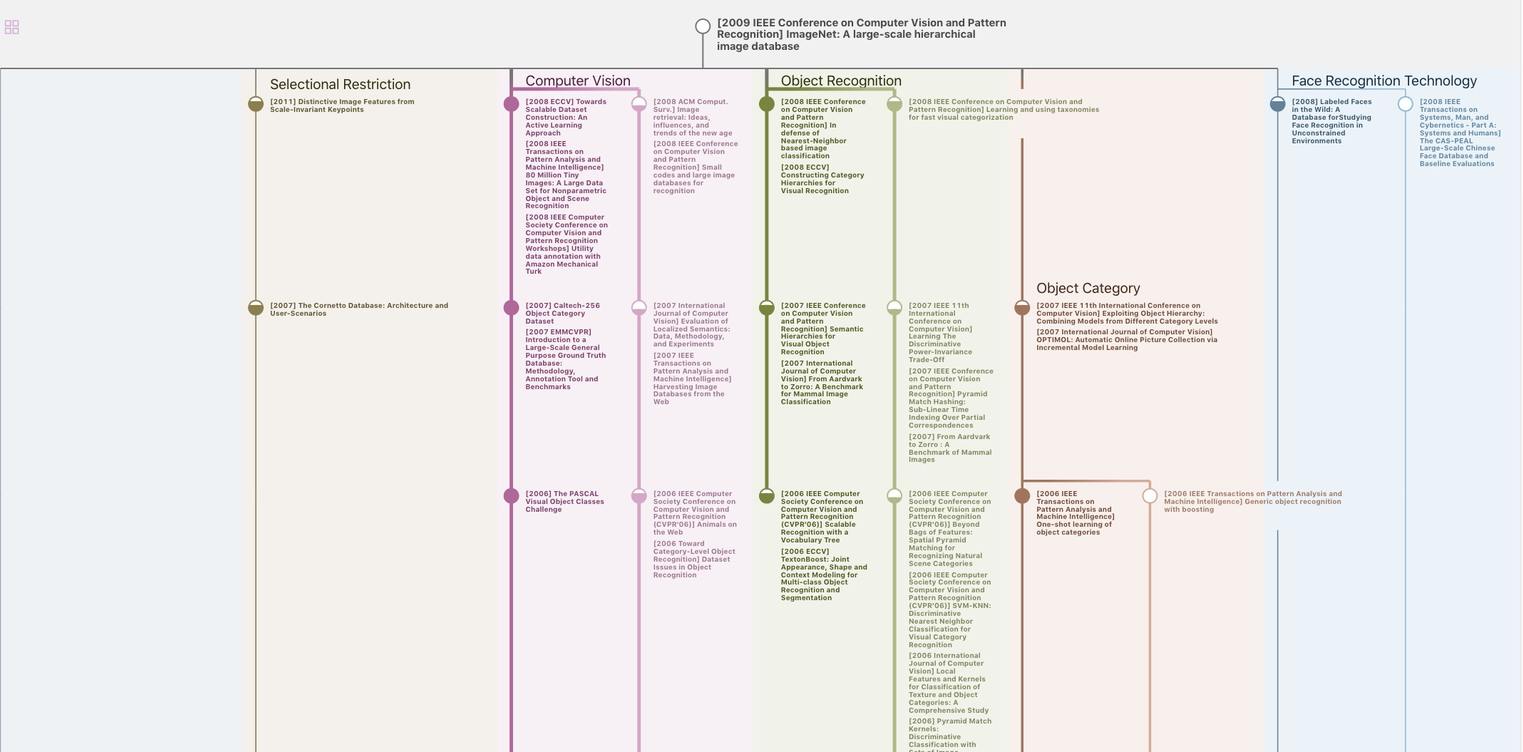
生成溯源树,研究论文发展脉络
Chat Paper
正在生成论文摘要