High-Dimensional Yield Estimation using Shrinkage Deep Features and Maximization of Integral Entropy Reduction
2023 28th Asia and South Pacific Design Automation Conference (ASP-DAC)(2023)
摘要
Despite the fast advances in high-sigma yield analysis with the help of machine learning techniques in the past decade, one of the main challenges, the curse of “dimensionality”, which is inevitable when dealing with modern large-scale circuits, remains unsolved. To resolve this challenge, we propose an absolute shrinkage deep kernel learning, ASDK, which automatically identifies the dominant process variation parameters in a nonlinear-correlated deep kernel and acts as a surrogate model to emulate the expensive SPICE simulation. To further improve the yield estimation efficiency, we propose a novel maximization of approximated entropy reduction for an efficient model update, which is also enhanced with parallel batch sampling for parallel computing, making it ready for practical deployment. Experiments on SRAM column circuits demonstrate the superiority of ASDK over the state-of-the-art (SOTA) approaches in terms of accuracy and efficiency with up to 11.1x speedup over SOTA methods.
更多查看译文
关键词
Yield Analysis,Bayesian Optimization,Failure Probability
AI 理解论文
溯源树
样例
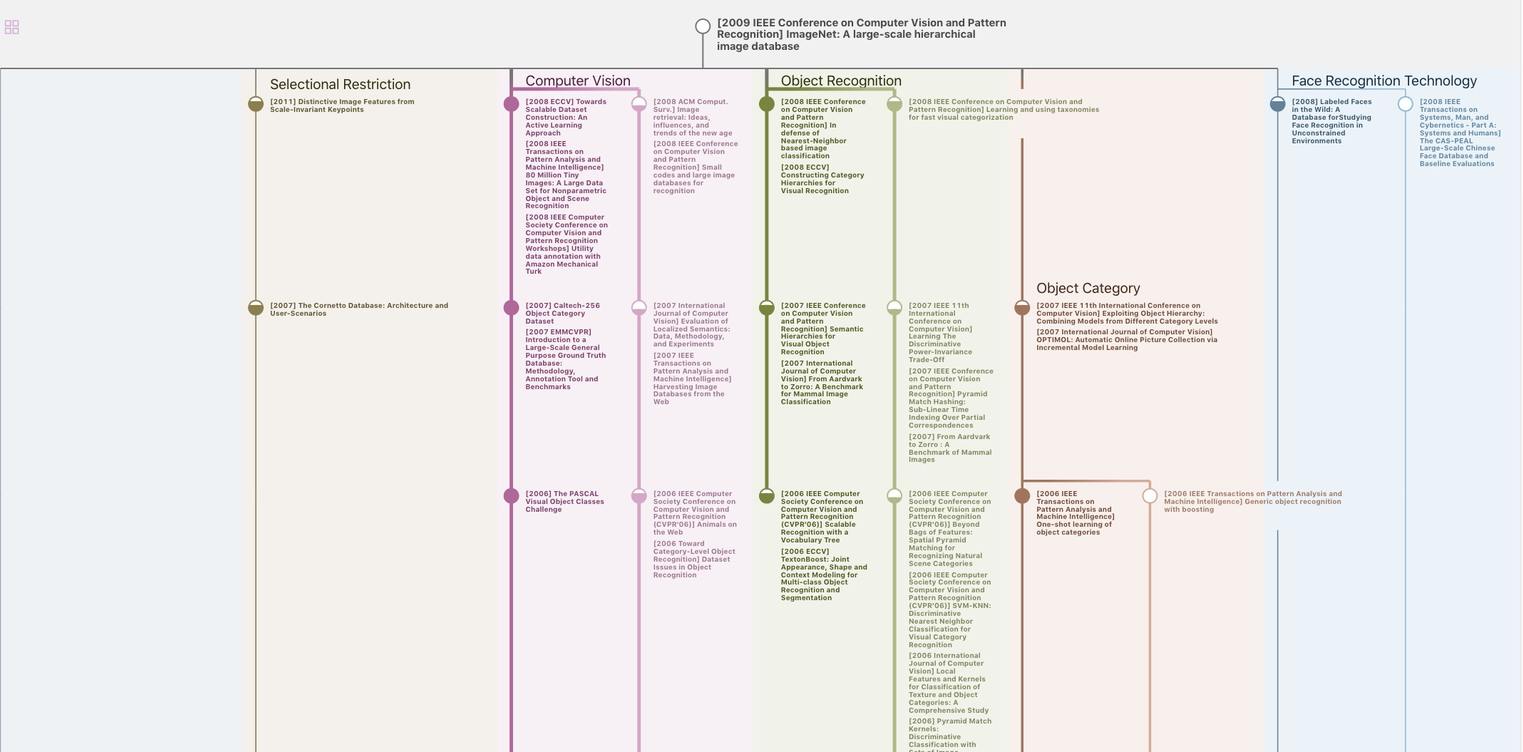
生成溯源树,研究论文发展脉络
Chat Paper
正在生成论文摘要