Fast and accurate factorized neural transducer for text adaption of end-to-end speech recognition models
arxiv(2023)
摘要
Neural transducer is now the most popular end-to-end model for speech recognition, due to its naturally streaming ability. However, it is challenging to adapt it with text-only data. Factorized neural transducer (FNT) model was proposed to mitigate this problem. The improved adaptation ability of FNT on text-only adaptation data came at the cost of lowered accuracy compared to the standard neural transducer model. We propose several methods to improve the performance of the FNT model. They are: adding CTC criterion during training, adding KL divergence loss during adaptation, using a pre-trained language model to seed the vocabulary predictor, and an efficient adaptation approach by interpolating the vocabulary predictor with the n-gram language model. A combination of these approaches results in a relative word-error-rate reduction of 9.48\% from the standard FNT model. Furthermore, n-gram interpolation with the vocabulary predictor improves the adaptation speed hugely with satisfactory adaptation performance.
更多查看译文
关键词
neural transducer model,factorized transducer model,KL divergence,n-gram
AI 理解论文
溯源树
样例
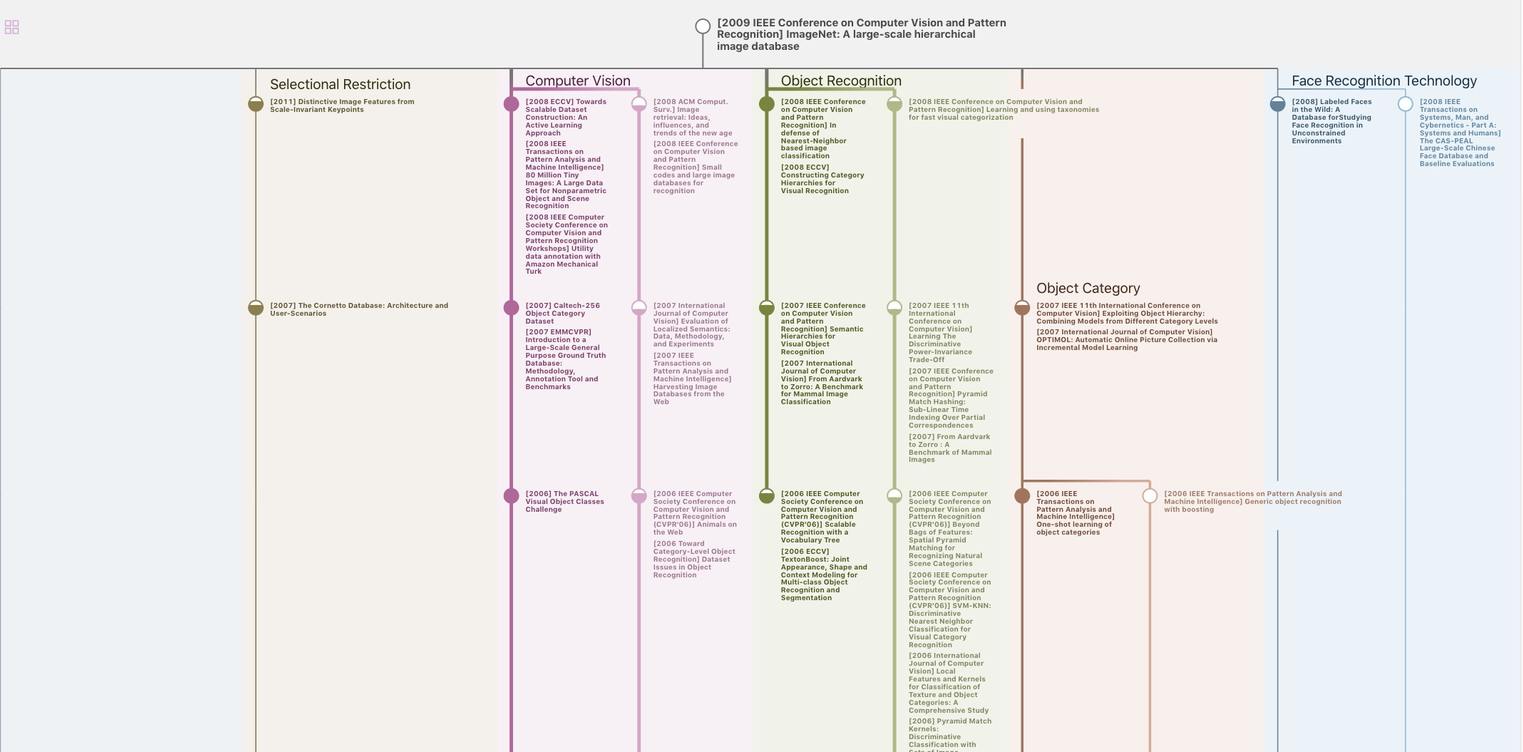
生成溯源树,研究论文发展脉络
Chat Paper
正在生成论文摘要