Automaton-Based Representations of Task Knowledge from Generative Language Models
arxiv(2023)
摘要
Automaton-based representations of task knowledge play an important role in control and planning for sequential decision-making problems. However, obtaining the high-level task knowledge required to build such automata is often difficult. Meanwhile, large-scale generative language models (GLMs) can automatically generate relevant task knowledge. However, the textual outputs from GLMs cannot be formally verified or used for sequential decision-making. We propose a novel algorithm named GLM2FSA, which constructs a finite state automaton (FSA) encoding high-level task knowledge from a brief natural-language description of the task goal. GLM2FSA first sends queries to a GLM to extract task knowledge in textual form, and then it builds an FSA to represent this text-based knowledge. The proposed algorithm thus fills the gap between natural-language task descriptions and automaton-based representations, and the constructed FSA can be formally verified against user-defined specifications. We accordingly propose a method to iteratively refine the queries to the GLM based on the outcomes, e.g., counter-examples, from verification. We demonstrate GLM2FSA's ability to build and refine automaton-based representations of everyday tasks (e.g., crossing a road or making a phone call), and also of tasks that require highly-specialized knowledge (e.g., executing secure multi-party computation).
更多查看译文
关键词
task knowledge,language models,generative
AI 理解论文
溯源树
样例
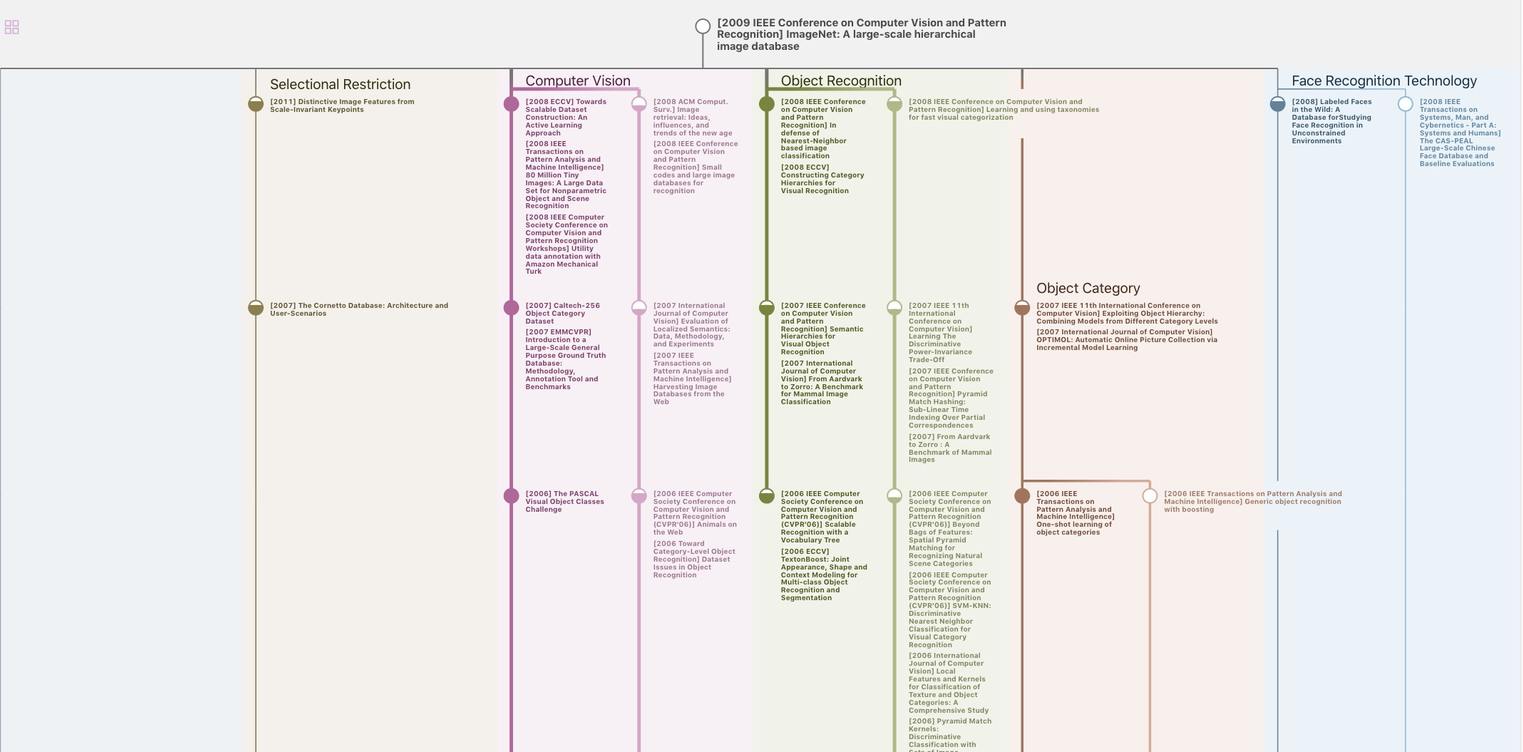
生成溯源树,研究论文发展脉络
Chat Paper
正在生成论文摘要