Discovering Class-Specific GAN Controls for Semantic Image Synthesis
arxiv(2023)
摘要
Prior work has extensively studied the latent space structure of GANs for unconditional image synthesis, enabling global editing of generated images by the unsupervised discovery of interpretable latent directions. However, the discovery of latent directions for conditional GANs for semantic image synthesis (SIS) has remained unexplored. In this work, we specifically focus on addressing this gap. We propose a novel optimization method for finding spatially disentangled class-specific directions in the latent space of pretrained SIS models. We show that the latent directions found by our method can effectively control the local appearance of semantic classes, e.g., changing their internal structure, texture or color independently from each other. Visual inspection and quantitative evaluation of the discovered GAN controls on various datasets demonstrate that our method discovers a diverse set of unique and semantically meaningful latent directions for class-specific edits.
更多查看译文
关键词
gan,synthesis,controls,class-specific
AI 理解论文
溯源树
样例
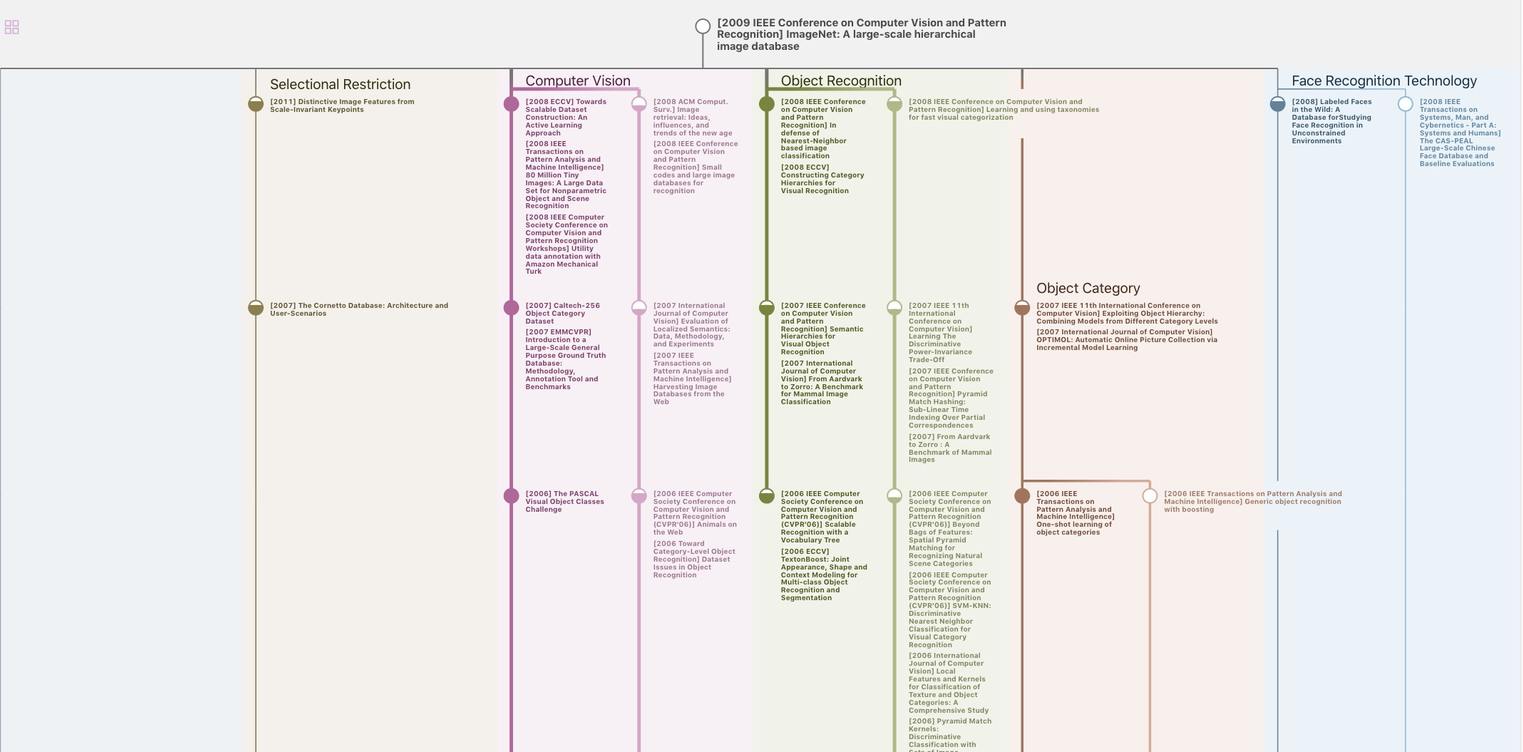
生成溯源树,研究论文发展脉络
Chat Paper
正在生成论文摘要