Exploring the use of topological data analysis to automatically detect data quality faults
FRONTIERS IN BIG DATA(2022)
摘要
Data quality problems may occur in various forms in structured and semi-structured data sources. This paper details an unsupervised method of analyzing data quality that is agnostic to the semantics of the data, the format of the encoding, or the internal structure of the dataset. A distance function is used to transform each record of a dataset into an n-dimensional vector of real numbers, which effectively transforms the original data into a high-dimensional point cloud. The shape of the point cloud is then efficiently examined via topological data analysis to find high-dimensional anomalies that may signal quality issues. The specific quality faults examined in this paper are the detection of records that, while not exactly the same, refer to the same entity. Our algorithm, based on topological data analysis, provides similar accuracy for both higher and lower quality data and performs better than a baseline approach for data with poor quality.
更多查看译文
关键词
topological data analysis,Morse-Smale complex,entity resolution,unsupervised,distance-based point cloud
AI 理解论文
溯源树
样例
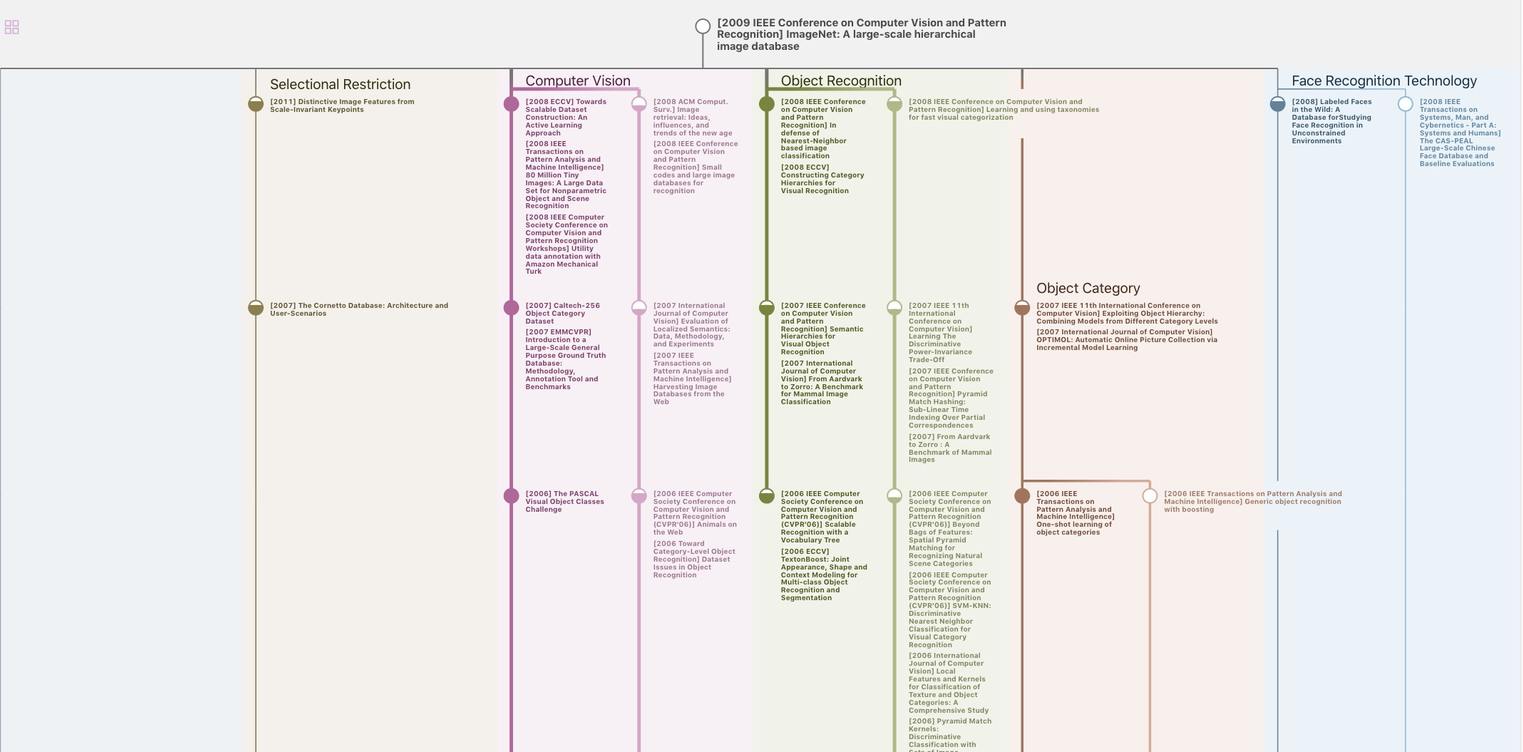
生成溯源树,研究论文发展脉络
Chat Paper
正在生成论文摘要