Learning to Caching Under the Partial-feedback Regime
2022 18th International Conference on Network and Service Management (CNSM)(2022)
摘要
We consider the caching problem in an online learning perspective, i.e., no model assumptions and prior knowledge for the file request sequence. Our goal is to design an efficient on-line caching policy with minimal regret, i.e, minimizing the total number of cache-miss with respect to the best static configuration in hindsight. Previous studies such as Follow-The-Perturbed-Leader (FTPL) caching policy, have provided some near-optimal results, but their theoretical performance guarantees only valid for the regime wherein all arrival requests could be seen by the cache, which is not the case in some practical scenarios like caching at cellular base station, content dissemination via DNS, etc. Hence our work study the partial-feedback regime wherein only requests for currently cached files are seen by the cache, which is more challenging and has not been studied before in the online learning perspective. We propose an online caching policy combining the FTPL with a novel popularity estimation procedure called Geometric Resampling (GR), and show that it yields the first sublinear regret guarantee in this regime. We also conduct numerical experiments to validate the theoretical guarantees of our caching policy.
更多查看译文
关键词
Online learning,Online caching policies,Quality of service (QoS)
AI 理解论文
溯源树
样例
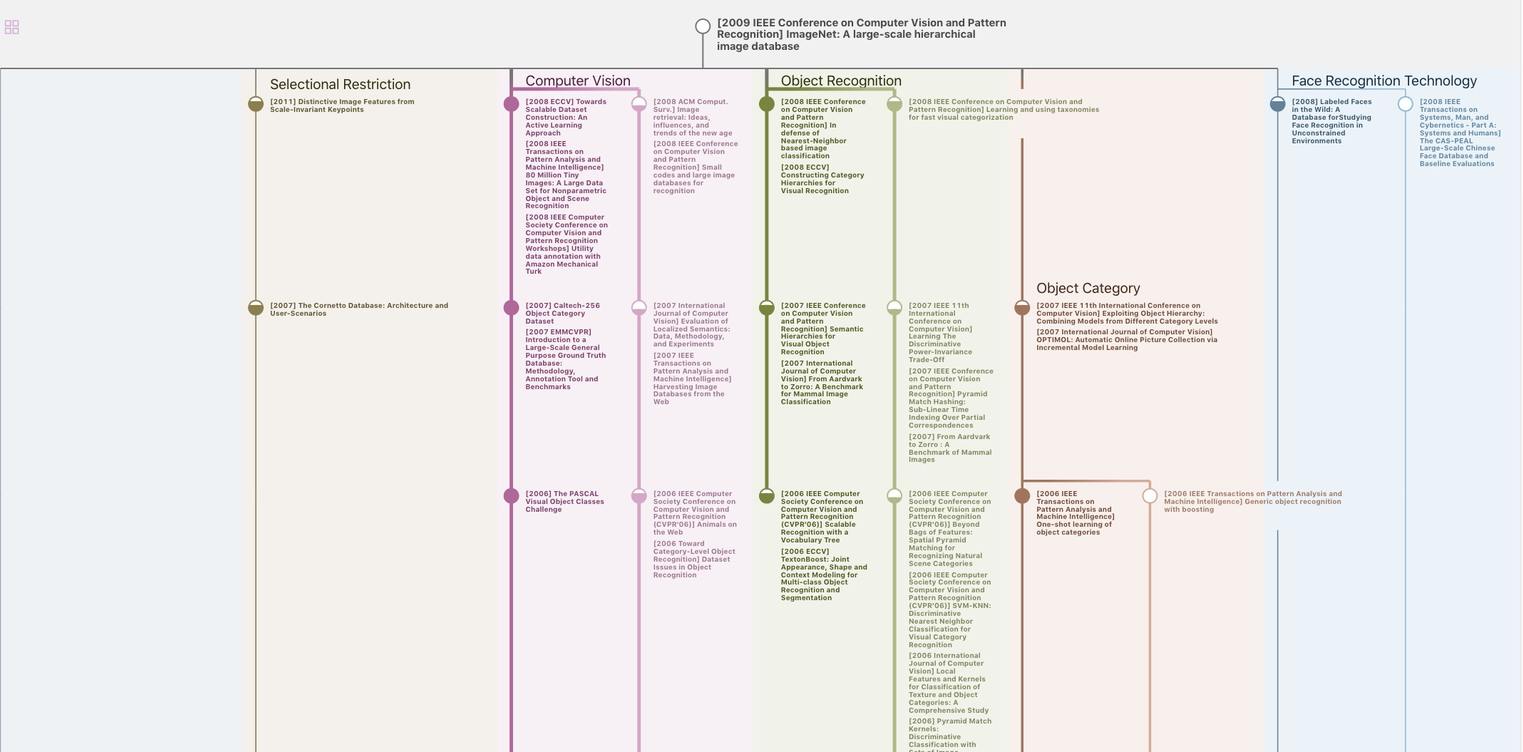
生成溯源树,研究论文发展脉络
Chat Paper
正在生成论文摘要