Paragraph-level Tibetan Question Generation for Machine Reading Comprehension
2022 International Conference on Asian Language Processing (IALP)(2022)
摘要
The question generation task can automatically generate large-scale questions to provide training data for reading comprehension tasks and QA systems, which are crucial for low-resource languages such as Tibetan. At present, due to the emergence of large-scale datasets and pre-trained language models in the Chinese and English domains, the task of question generation in the Chinese and English domains has been well developed, while the research on question generation in the Tibetan is still in its infancy. The main reason is the lack of datasets and the relatively backward development of various models in Tibetan. To solve the above questions, this paper constructs a Tibetan pre-trained language model TiBERT to provide a basis for the development of various downstream tasks, to expand the datasets of Tibetan machine reading comprehension, this paper proposes a Tibetan question generation model named TQGR. The model consists of two parts, the question generation and question quality assessment. The question generation adopts the classic seq2seq architecture to generate questions, and the question quality assessment improves the quality of generated questions by evaluating the fluency reward score, word repetition rate reward score and interrogative words classification auxiliary task. Finally, the experimental results show that our model has higher performance than baseline models, and ablation experiments demonstrate the effectiveness of the three mechanism.
更多查看译文
关键词
Tibetan Question Generation,Seq2Seq Frame-work,Reward Scores,Auxiliary Task
AI 理解论文
溯源树
样例
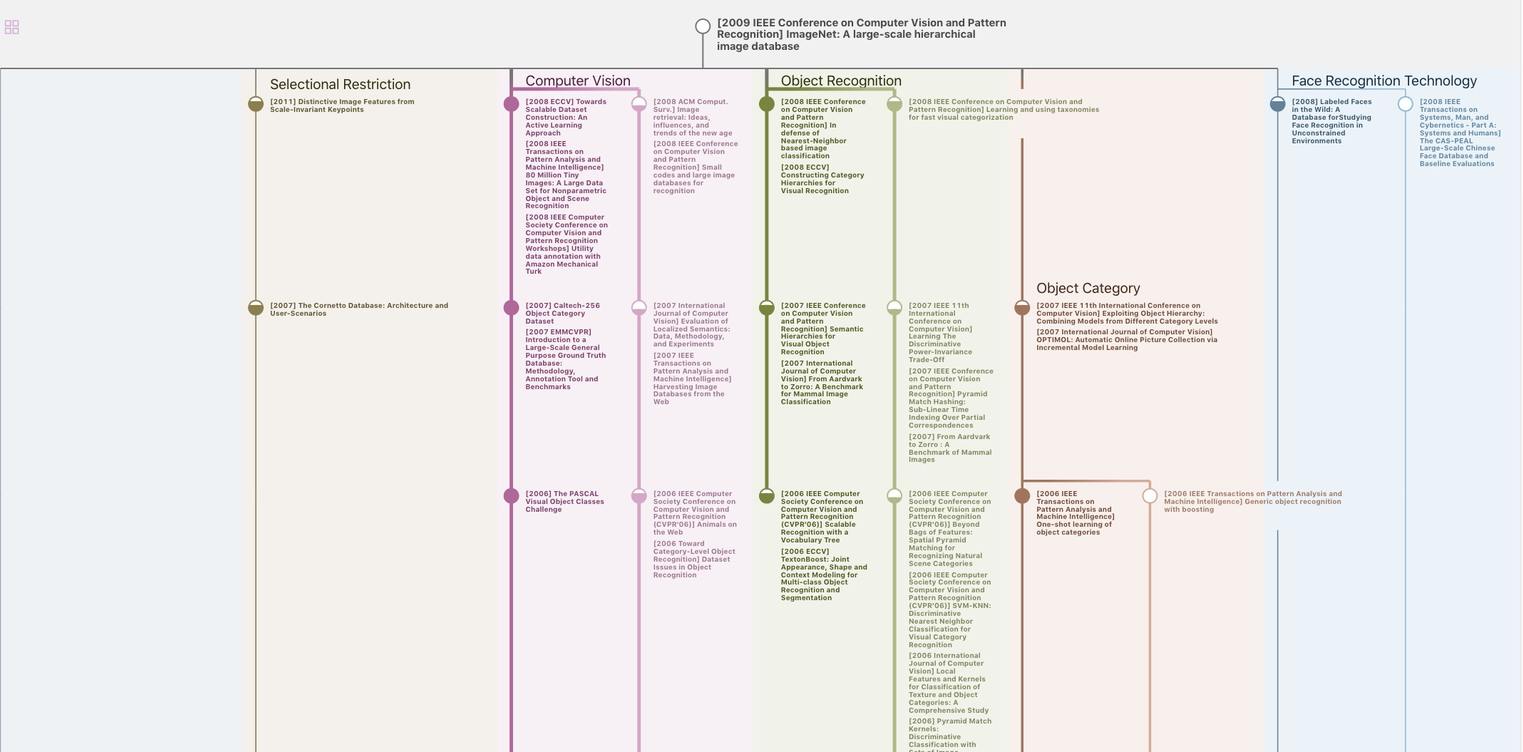
生成溯源树,研究论文发展脉络
Chat Paper
正在生成论文摘要