Equalization with Time Domain Preprocessing for OFDM and FBMC in Flat Fading Fast Varying Channels
2022 IEEE 6th International Symposium on Telecommunication Technologies (ISTT)(2022)
摘要
In Multi-Carrier (MC) systems, all equalization and detection procedures are classically performed at the sub-carrier level after the received signal is projected into the frequency domain. Besides, in the presence of rapid channel variation, conventional receivers suffer from critical performance degradation caused by interference. To address this specific problem, we propose to add a low-complexity time domain preprocessing prior to the frequency domain equalization process. We specifically study Orthogonal Frequency Division Multiplexing (OFDM) and Filter-Bank Multi-Carrier (FBMC) over single-path fast-varying Rayleigh channels when using the proposed scheme. Two time domain preprocessing techniques are considered. Their impact on equalization performance is evaluated in perfect and imperfect Channel State Information (CSI) scenarios, showing robustness to reasonable channel estimation errors. For both systems, a reduction in Bit Error Rate (BER) is obtained thanks to the preprocessing. Furthermore, the proposed scheme allows for capturing time diversity leading to improvement in performance for faster channel variation, rather than inducing performance degradation, showing the relevance of our approach. For OFDM, this preprocessing allows reaching the best performance compared to the existing equalizers at significant lower complexity. For FBMC, it permits to obtain a performance gain of 10 dB at $F_{d}T_{S}=0.25$, while avoiding the BER floor effect. This gain is observed at BER =$2\times$ $10^{-2}$ for scenarios accepting Inter-Symbol Interference (ISI), and at BER =1$0^{-3}$ when assuming perfect ISI cancellation. For the latter scenario, we obtain performance very close to the (computationally intractable) optimum Maximum-Likelihood equalizer.
更多查看译文
关键词
OFDM,FBMC,Doppler,Rayleigh,QR,Equalizer
AI 理解论文
溯源树
样例
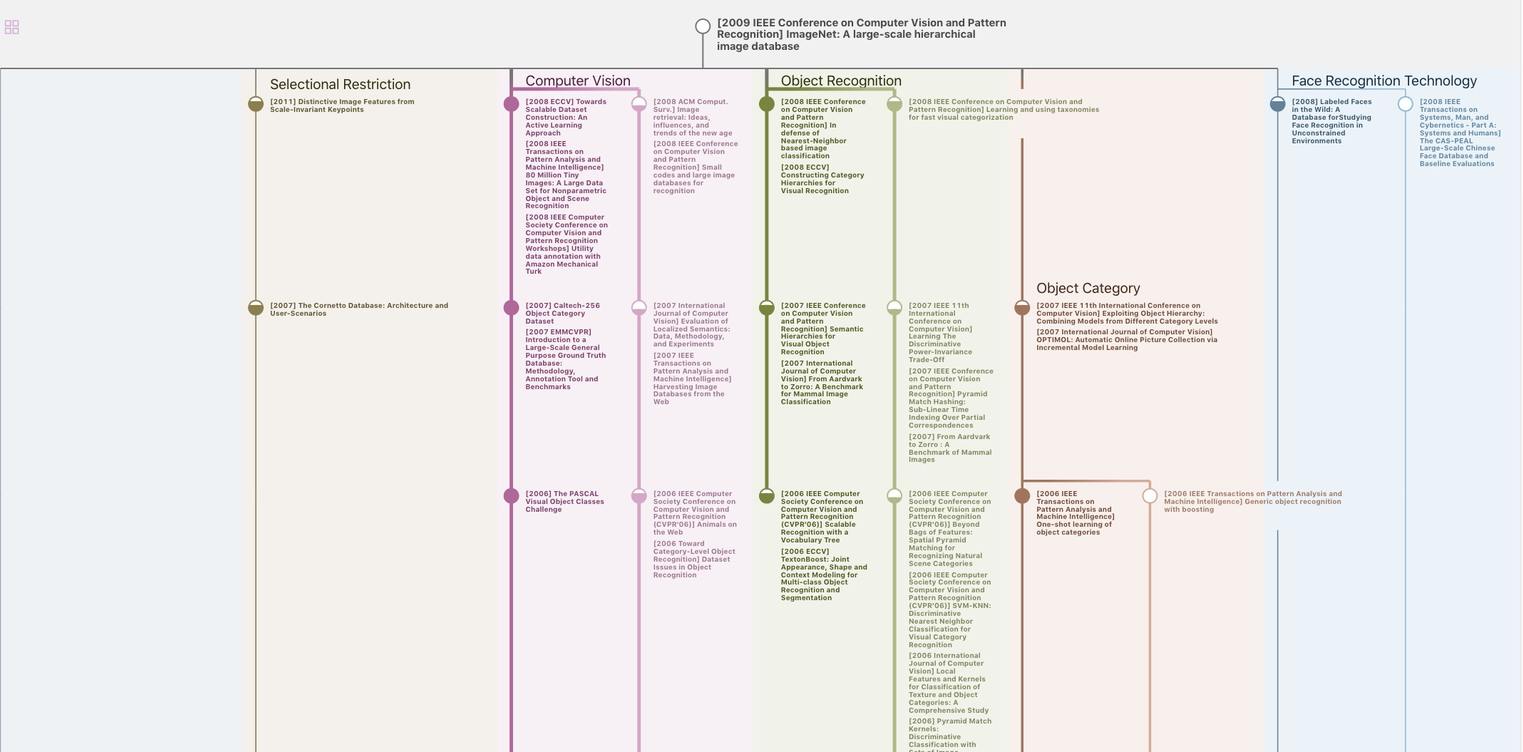
生成溯源树,研究论文发展脉络
Chat Paper
正在生成论文摘要