Aircraft Braking Performance Classification Through Braking Rollout Clustering
2022 IEEE/AIAA 41st Digital Avionics Systems Conference (DASC)(2022)
摘要
The IATA safety report shows that runway excursions are the major cause of accidents when organized by flight phase. Additionally, runway overrun of the available runway length is the dominant accident type in the runway excursion category. To better evaluate runway overrun events, it is important to understand the behavior of the aircraft on the runway. During landing, this behavior is largely determined by the aircraft braking effectiveness. Aircraft braking may be considered effective when the braking coefficient is sufficient to drain all of the mechanical energy of the aircraft within a given amount of runway. Aircraft braking effectiveness may vary significantly during each landing event due to external factors such as runway contamination, runway material composition, and the proper use of ground spoilers. Aircraft braking effectiveness may be evaluated using a physics-based and data-based approach. Furthermore, as more and more aircraft fly and as more and more of these aircraft are equipped with a variety of sensors, digital data recording of aircraft parameters become more readily available. Advancements in machine learning techniques make it possible to analyze and make sense of such large amounts of data more easily. In particular, machine learning allows "big data" analysis of aircraft braking effectiveness. This study introduces an unsupervised machine learning technique to evaluate braking performance, in terms of aircraft braking coefficient, by clustering braking roll-out data. The technique clusters flights by quantifying the pairwise similarity of each braking roll-out trajectory. The clustering labels are used to compare the relative effectiveness in braking for peer flights. This technique may be used to identify the nominal braking behavior based on safely landed flights for a given aircraft type. The identified nominal behavior may be used to better evaluate future flights. The material in this paper demonstrates the practicality of a clustering analysis of aircraft behavior during roll-out.
更多查看译文
关键词
braking,landing,clustering,machine learning,classification
AI 理解论文
溯源树
样例
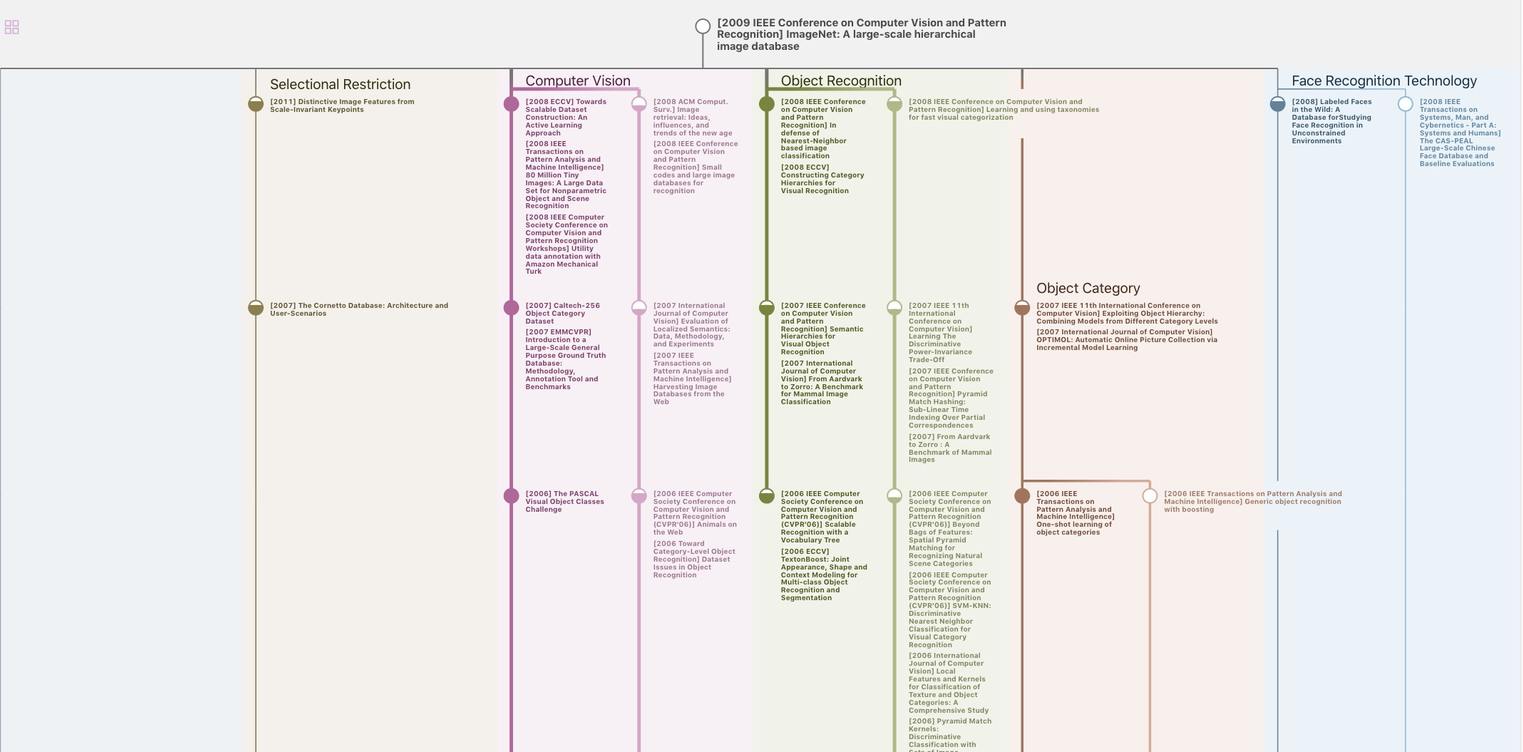
生成溯源树,研究论文发展脉络
Chat Paper
正在生成论文摘要