Residual Time-restricted Self-Attentive TDNN Speaker Embedding for Noisy and Far-field Conditions
2022 IEEE Canadian Conference on Electrical and Computer Engineering (CCECE)(2022)
摘要
One of the emerging challenges in automatic speaker recognition is the development of systems that are robust to noisy and far-field conditions. The current standard for x-vector speaker embedding is based on a time-delay neural network (TDNN) and is less robust than systems based on a residual network (ResNet) and other baseline systems that use signal enhancement preprocessing in presence of these conditions. In this study, we improve the performance of TDNN-based embedding by integrating a residual block with a time-restricted self-attention option (AttResBlock) into the TDNN frame level. Experiments using the Voices Obscured in Complex Environmental Settings (VOiCES) corpus are carried out to evaluate the proposed speaker embedding extractor (AttResBlock-TDNN). The experimental results show that AttResBlock-TDNN outperforms state-of-the-art systems under many adverse conditions. For instance, the proposed AttResBlock-TDNN produces relative improvements in the minDCF and EER of 11.4% and 15.5%, respectively, over the original TDNN-based encoder.
更多查看译文
关键词
speaker recognition,noisy conditions,far-field,speaker embedding,residual block,self-attention mechanism
AI 理解论文
溯源树
样例
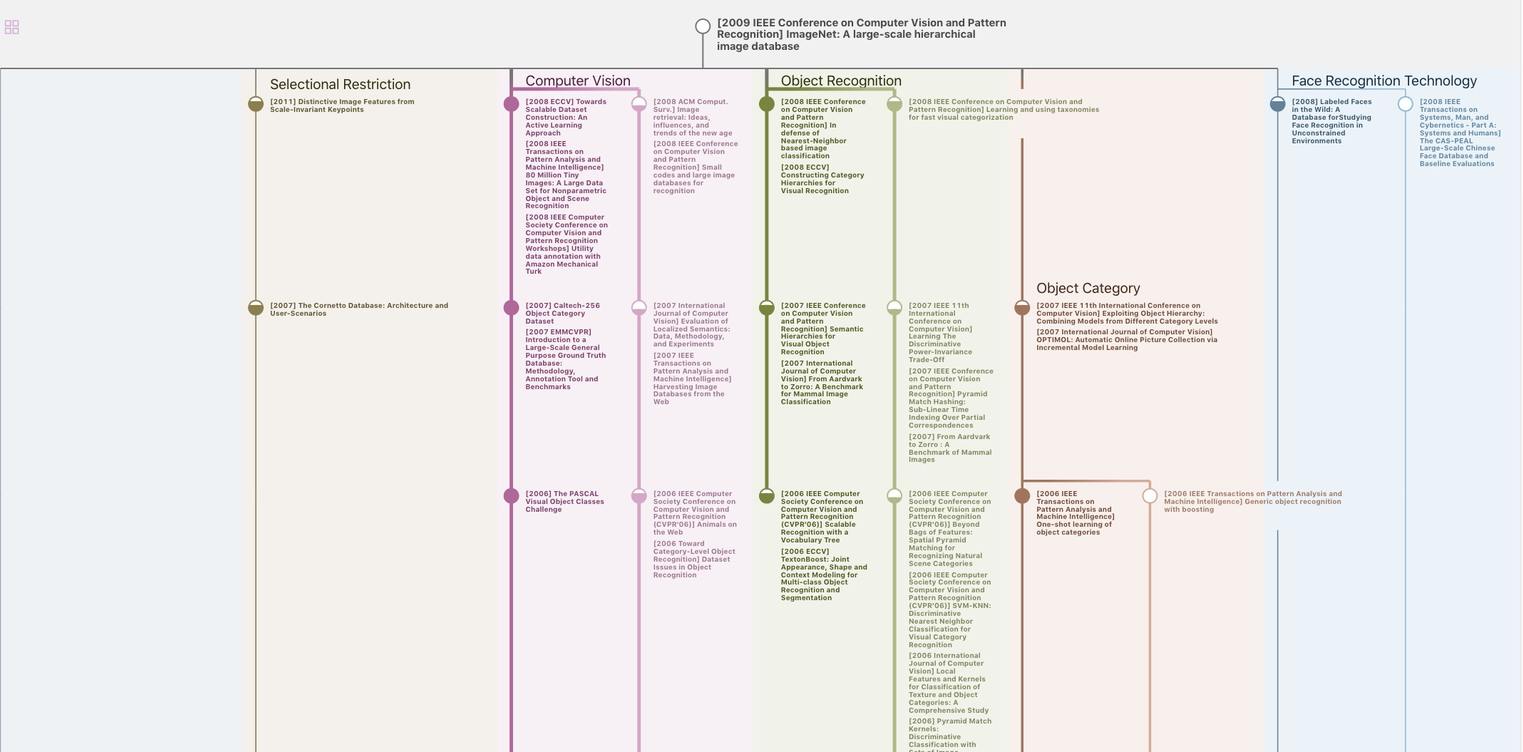
生成溯源树,研究论文发展脉络
Chat Paper
正在生成论文摘要