Superiority of the Neural Network Dynamic Regression Models for Ontario Electricity Demand Forecasting
2022 IEEE Canadian Conference on Electrical and Computer Engineering (CCECE)(2022)
摘要
Electricity demand forecasting is an essential step in balancing the electricity grid. A balanced electricity grid helps make efficient decisions in production planning and scheduling. The study of uncertainty in electricity demand requires more advanced hybrid forecasting approaches, which combine statistical methods and machine learning techniques. In electricity demand forecasting, the features such as temperature and day-type are suggested as the most influential features in the literature. The novelty of this paper is to use Canadian electricity demand data and explore the effect of temperature and day-type on electricity demand using hybrid models. The hybrid neural network dynamic regression (NNDR) model is used to obtain the point forecasts/predictions for Ontario electricity demand data. The experimental results show the superiority of the proposed NNDR model over the commonly used dynamic regression models with the Seasonal Autoregressive Integrated Moving Average (DRSARIMA) errors model and the Prophet model. Moreover, long-term point forecasts and innovations are used to obtain two classes of prediction intervals (PIs) using data-driven probabilistic innovation distribution and bootstrapping for NNDR, DRSARIMA and Prophet models.
更多查看译文
关键词
Innovations,Forecasting,Prediction intervals,Neural Network Dynamic Regression,Prophet Model,Ontario Electricity Demand
AI 理解论文
溯源树
样例
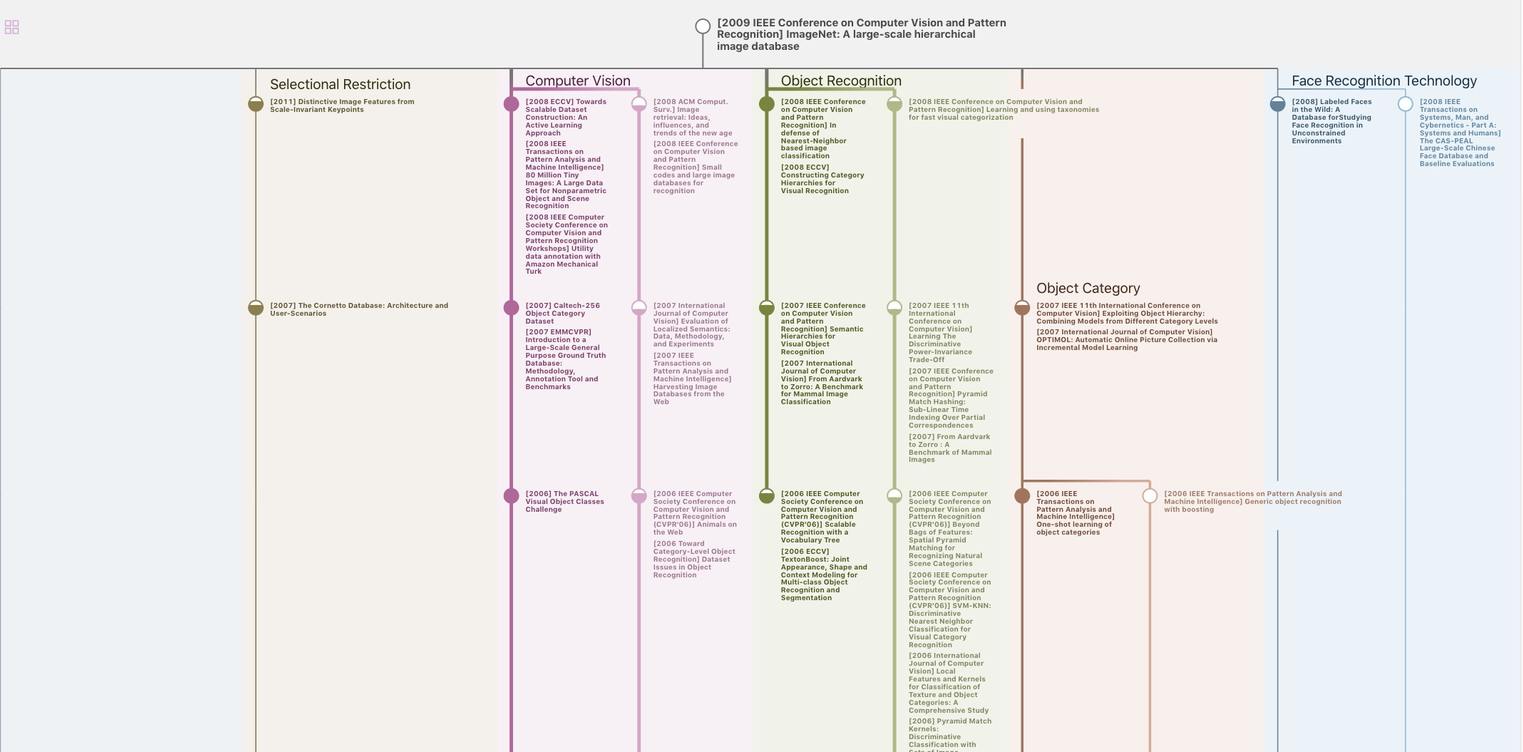
生成溯源树,研究论文发展脉络
Chat Paper
正在生成论文摘要