D2F2WOD: Learning Object Proposals for Weakly-Supervised Object Detection via Progressive Domain Adaptation
arxiv(2023)
摘要
Weakly-supervised object detection (WSOD) models attempt to leverage image-level annotations in lieu of accurate but costly-to-obtain object localization labels. This oftentimes leads to substandard object detection and localization at inference time. To tackle this issue, we propose D(2)F2WOD, a Dual-Domain Fully-to-Weakly Supervised Object Detection framework that leverages synthetic data, annotated with precise object localization, to supplement a natural image target domain, where only image-level labels are available. In its warm-up domain adaptation stage, the model learns a fully-supervised object detector (FSOD) to improve the precision of the object proposals in the target domain, and at the same time learns target-domain-specific and detection-aware proposal features. In its main WSOD stage, a WSOD model is specifically tuned to the target domain. The feature extractor and the object proposal generator of the WSOD model are built upon the fine-tuned FSOD model. We test D(2)F2WOD on five dual-domain image benchmarks. The results show that our method results in consistently improved object detection and localization compared with state-of-the-art methods.
更多查看译文
关键词
Algorithms: Image recognition and understanding (object detection,categorization,segmentation),Machine learning architectures,formulations,and algorithms (including transfer,low-shot,semi-,self-,and un-supervised learning)
AI 理解论文
溯源树
样例
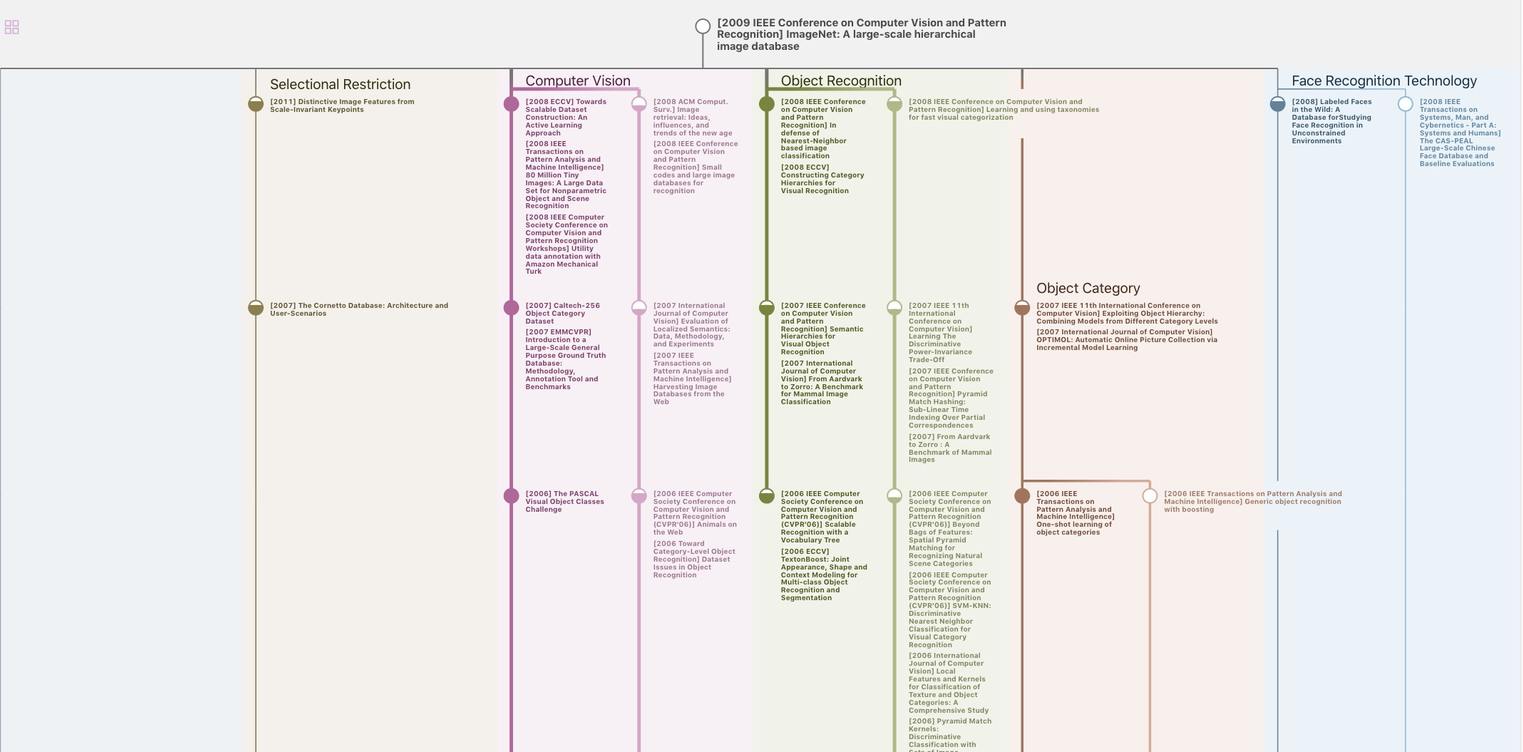
生成溯源树,研究论文发展脉络
Chat Paper
正在生成论文摘要