Reconstructing particles in jets using set transformer and hypergraph prediction networks
arxiv(2023)
摘要
The task of reconstructing particles from low-level detector response data to predict the set of final state particles in collision events represents a set-to-set prediction task requiring the use of multiple features and their correlations in the input data. We deploy three separate set-to-set neural network architectures to reconstruct particles in events containing a single jet in a fully-simulated calorimeter. Performance is evaluated in terms of particle reconstruction quality, properties regression, and jet-level metrics. The results demonstrate that such a high-dimensional end-to-end approach succeeds in surpassing basic parametric approaches in disentangling individual neutral particles inside of jets and optimizing the use of complementary detector information. In particular, the performance comparison favors a novel architecture based on learning hypergraph structure, HGPflow , which benefits from a physically-interpretable approach to particle reconstruction.
更多查看译文
关键词
jets,hypergraph
AI 理解论文
溯源树
样例
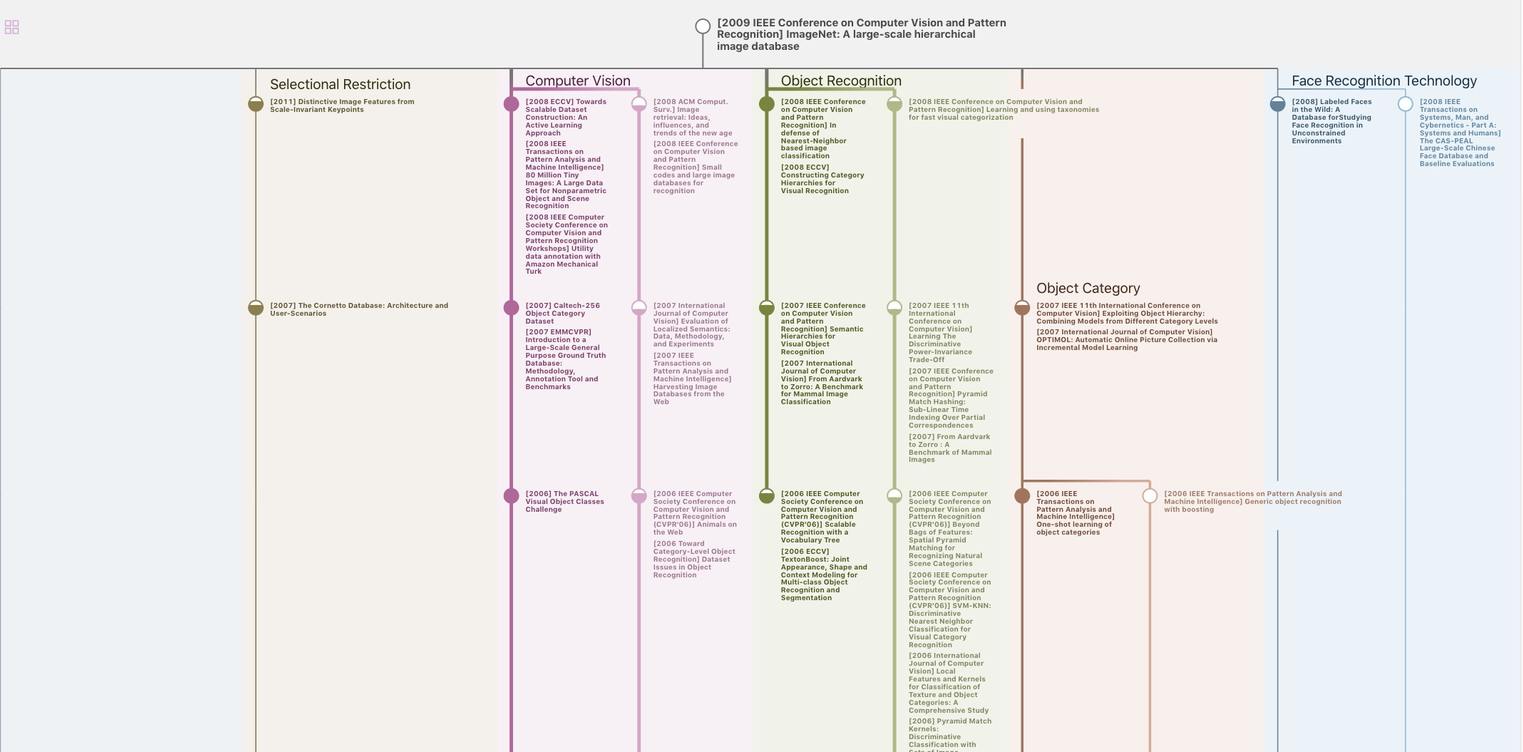
生成溯源树,研究论文发展脉络
Chat Paper
正在生成论文摘要