Initial Results for Pairwise Causal Discovery Using Quantitative Information Flow
arxiv(2022)
摘要
Pairwise Causal Discovery is the task of determining causal, anticausal, confounded or independence relationships from pairs of variables. Over the last few years, this challenging task has promoted not only the discovery of novel machine learning models aimed at solving the task, but also discussions on how learning the causal direction of variables may benefit machine learning overall. In this paper, we show that Quantitative Information Flow (QIF), a measure usually employed for measuring leakages of information from a system to an attacker, shows promising results as features for the task. In particular, experiments with real-world datasets indicate that QIF is statistically tied to the state of the art. Our initial results motivate further inquiries on how QIF relates to causality and what are its limitations.
更多查看译文
关键词
pairwise causal discovery,quantitative,information,flow
AI 理解论文
溯源树
样例
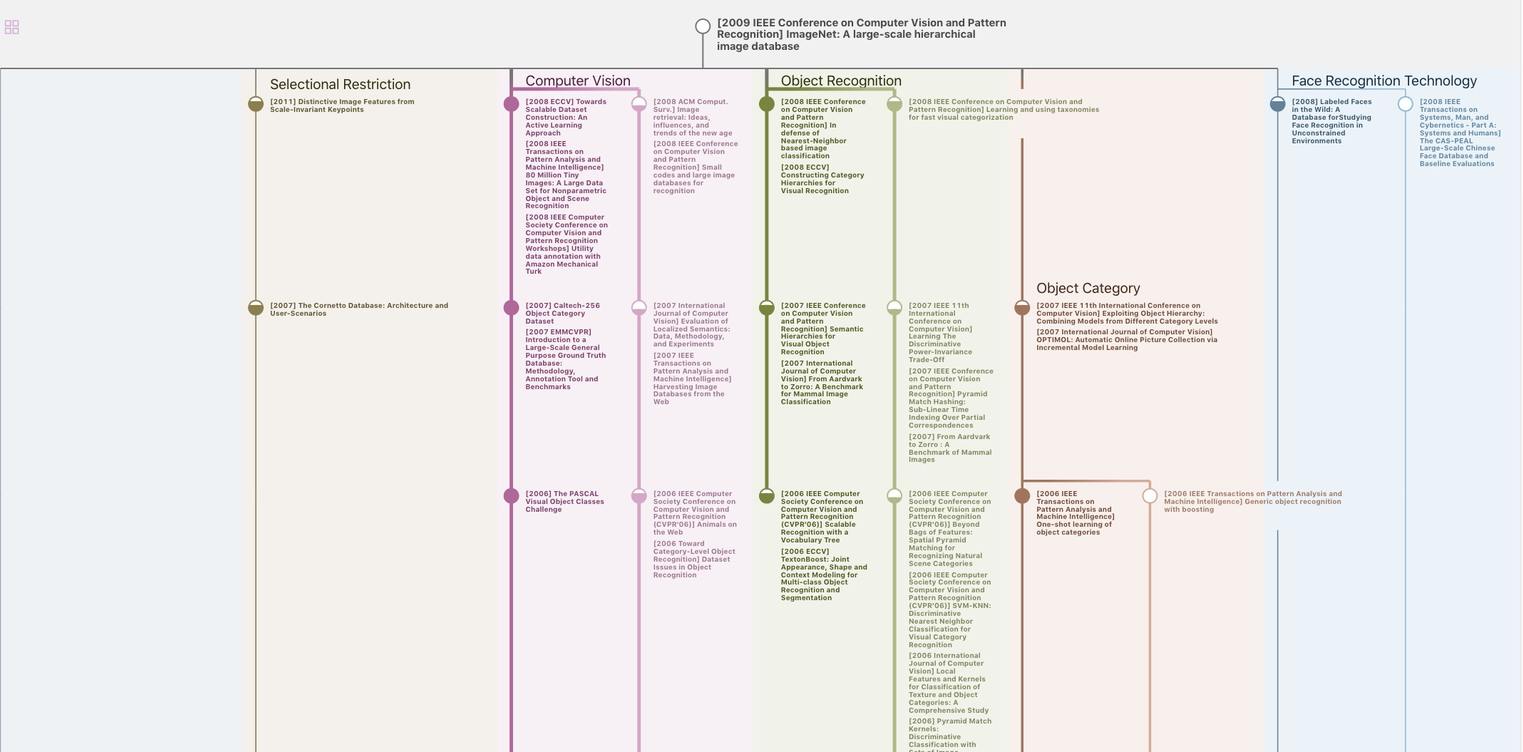
生成溯源树,研究论文发展脉络
Chat Paper
正在生成论文摘要