QFF: Quantized Fourier Features for Neural Field Representations
arxiv(2022)
摘要
Multilayer perceptrons (MLPs) learn high frequencies slowly. Recent approaches encode features in spatial bins to improve speed of learning details, but at the cost of larger model size and loss of continuity. Instead, we propose to encode features in bins of Fourier features that are commonly used for positional encoding. We call these Quantized Fourier Features (QFF). As a naturally multiresolution and periodic representation, our experiments show that using QFF can result in smaller model size, faster training, and better quality outputs for several applications, including Neural Image Representations (NIR), Neural Radiance Field (NeRF) and Signed Distance Function (SDF) modeling. QFF are easy to code, fast to compute, and serve as a simple drop-in addition to many neural field representations.
更多查看译文
关键词
quantized fourier features,representations,field
AI 理解论文
溯源树
样例
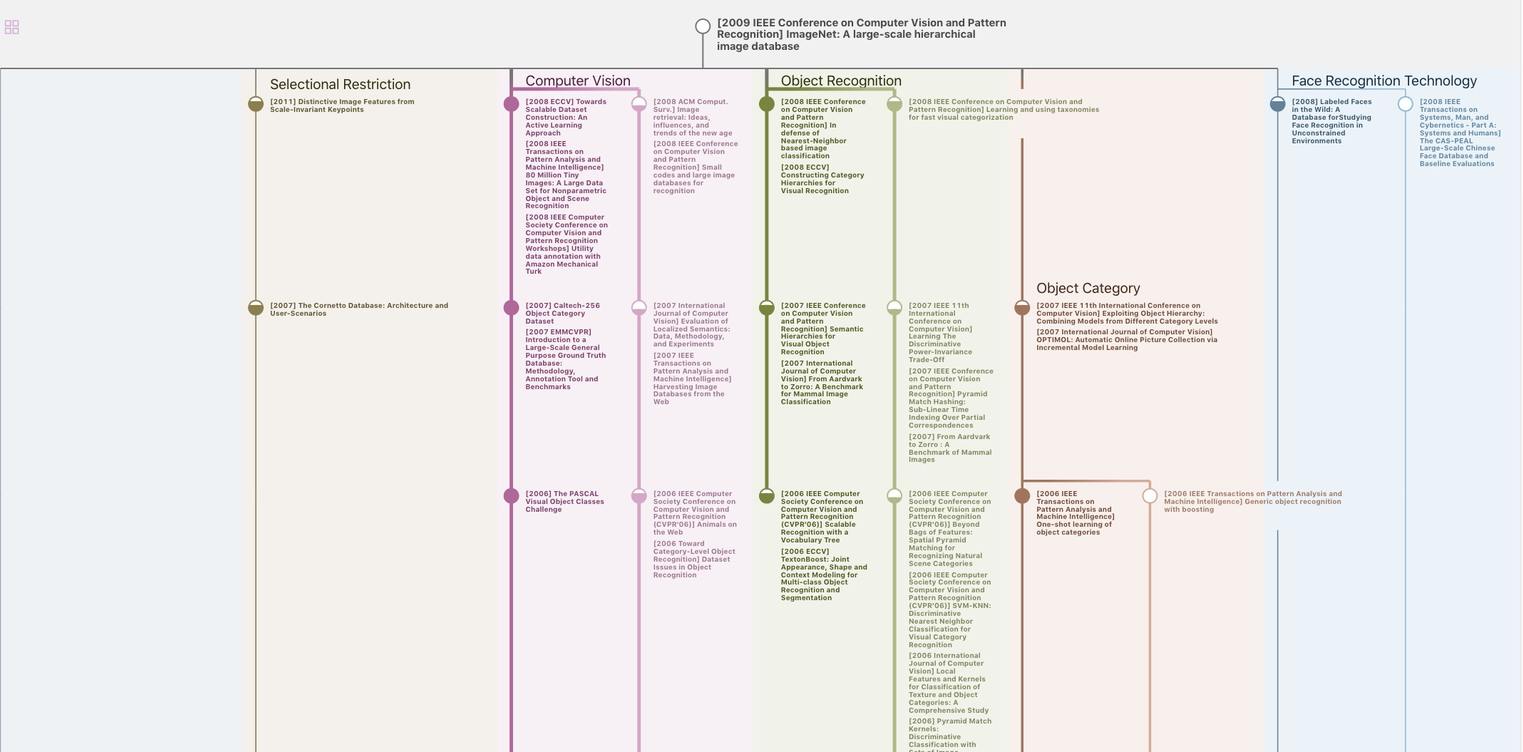
生成溯源树,研究论文发展脉络
Chat Paper
正在生成论文摘要