A Study on Adversarial Attacks and Defense Method on Binarized Neural Network
2022 International Conference on Advanced Technologies for Communications (ATC)(2022)
摘要
Binarized Neural Networks (BNNs) are relatively hardware-efficient neural network models which are seriously considered for edge-AI applications. However, BNNs are like other neural networks and exhibit certain linear properties and are vulnerable to adversarial attacks. This work evaluates the robustness of BNNs under Projected Gradient Descent (PGD) - one of the most powerful iterative adversarial attacks, on BNN models and analyzes the effectiveness of corresponding defense methods. Our extensive simulation shows that the network almost malfunction when performing recognition tasks when tested with PGD samples without adversarial training. On the other hand, adversarial training could significantly improve robustness for both BNNs and Deep learning neural networks (DNNs), though strong PGD attacks could still be challenging. Therefore, adversarial attacks are a real threat, and more effective adversarial defense methods and innovative network architectures may be required for practical applications.
更多查看译文
关键词
Binarized Neural Networks,Adversarial Attacks,Adversarial Training,Edge-AI
AI 理解论文
溯源树
样例
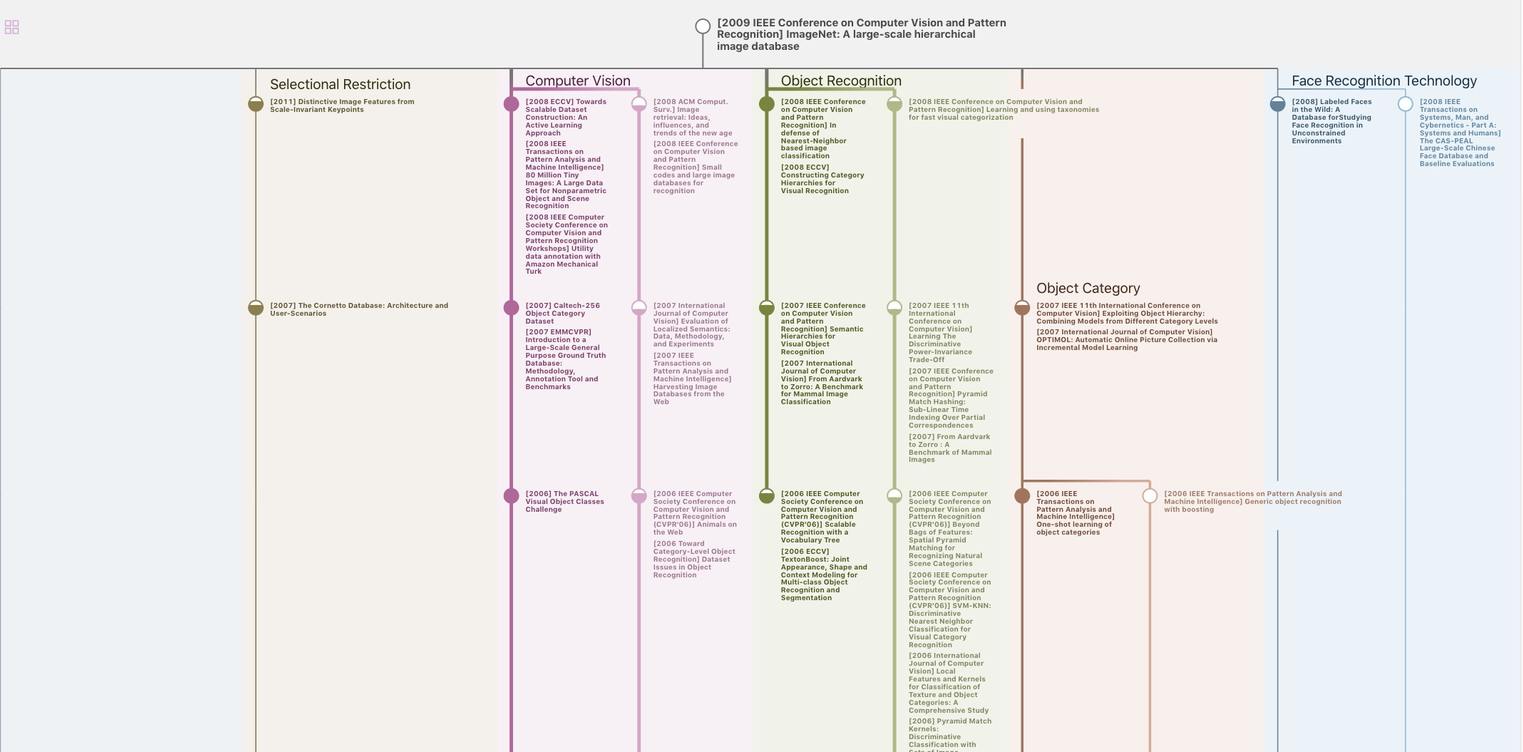
生成溯源树,研究论文发展脉络
Chat Paper
正在生成论文摘要