Blind Symbol Rate Estimation Using Wavelet Transform and Deep Learning for FSK Modulated Signals
2022 International Conference on Advanced Technologies for Communications (ATC)(2022)
摘要
This paper is focused on the blind symbol rate estimation for the digital FSK modulated signals, based on the classification between three symbol rate classes: 10, 100, and 1000 KSymbol/second using the scalogram images obtained from continuous wavelet transform with Morse wavelet. Pretrained deep learning AlexNet has been transfer learned to classify between symbol rate classes. Training, testing, and validation data sets have been composed of the artificial data generated using Bernoulli binary random signal generator modulated into FSK signal corrupted by additive white Gaussian noise (AWGN) noise with SNR ranging from 1 to 30 dB. Training and validation data sets have been augmented to obtain twice more extensive data set i.e 1800 scalogram images, compared to the original size of 900 samples. The average classification accuracy during validation has reached 99.7 % and during testing 100 % and 96.3 % for the data sets with SNR 25–30 dB and 20–25 dB respectively. The proposed algorithm has been compared with cyclostationary and has shown improved classification accuracy, especially in conditions of low SNR.
更多查看译文
关键词
deep learning,blind symbol rate classification,signal-to-noise ratio,cognitive radio
AI 理解论文
溯源树
样例
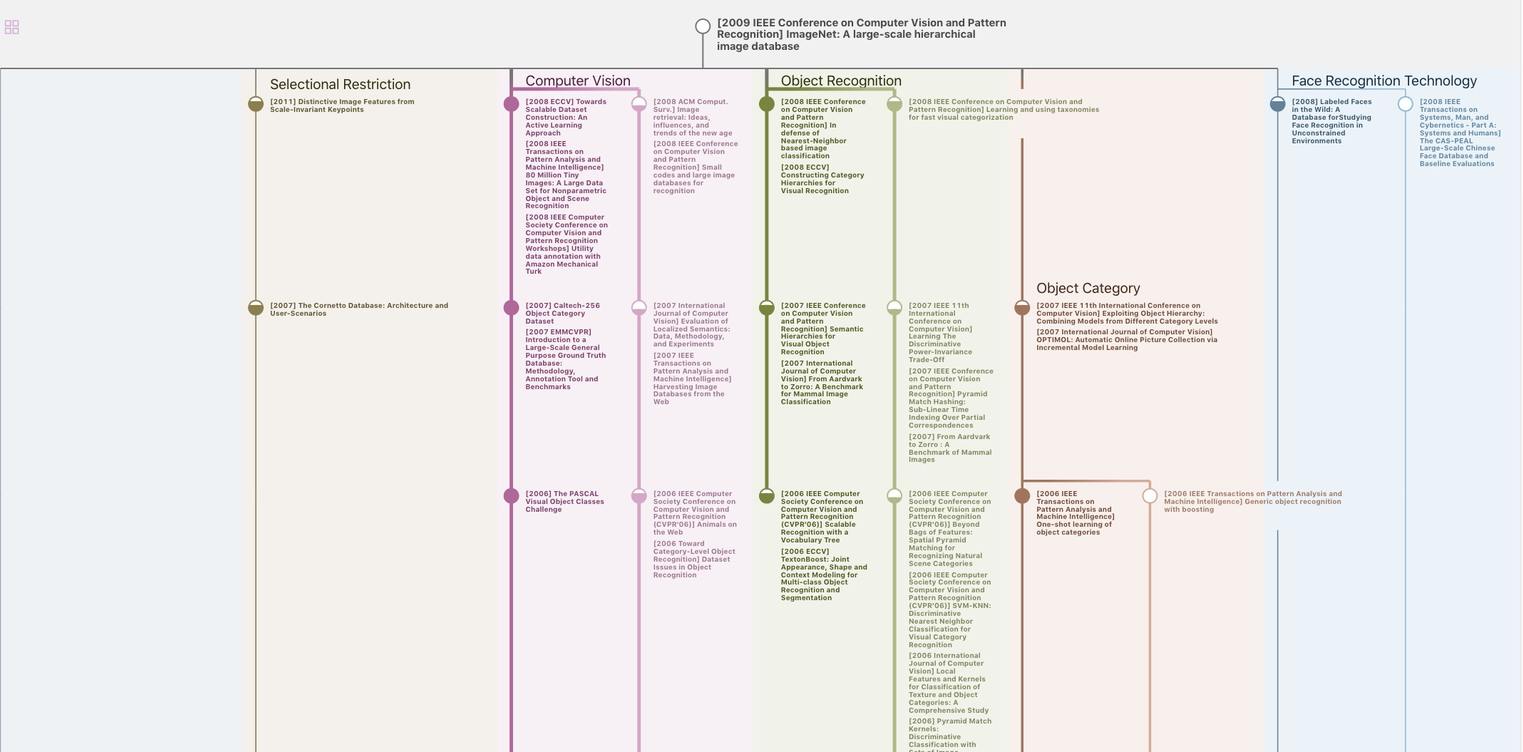
生成溯源树,研究论文发展脉络
Chat Paper
正在生成论文摘要