Domain Adaptation for Stance Detection towards Unseen Target on Social Media
2022 10th International Conference on Affective Computing and Intelligent Interaction (ACII)(2022)
摘要
Stance detection aims at identifying people's stand-point towards a given target. New targets are constantly appearing on social media, making previous annotated data unusable by stance detection models relying on classical supervised machine learning. Thus, cross-target stance detection which uses labeled data from source targets to learn a model that can be adapted to the destination new target, has become a prevailing research direction. However, previous methods rely on manually chosen similar source-destination target pairs and lack generalization to unseen targets with no explicit relation to known ones. To this end, we investigate the problem from a domain adaptation perspective and further propose a novel Unified Target-aware Domain Adaptation method (UTDA) that leverages knowledge transfer capability of transformer-based language model. The proposed method can effectively extract critical target-shared features for detecting stance by feature disentanglement and automatically learn to identify target relations. UTDA can easily be applied to a new unseen target since it does not rely on any pre-defined target pairs. Experimental results on two benchmark stance datasets demonstrate that our method achieves better performance than strong baselines.
更多查看译文
关键词
stance detection,domain adaptation,feature disentanglement
AI 理解论文
溯源树
样例
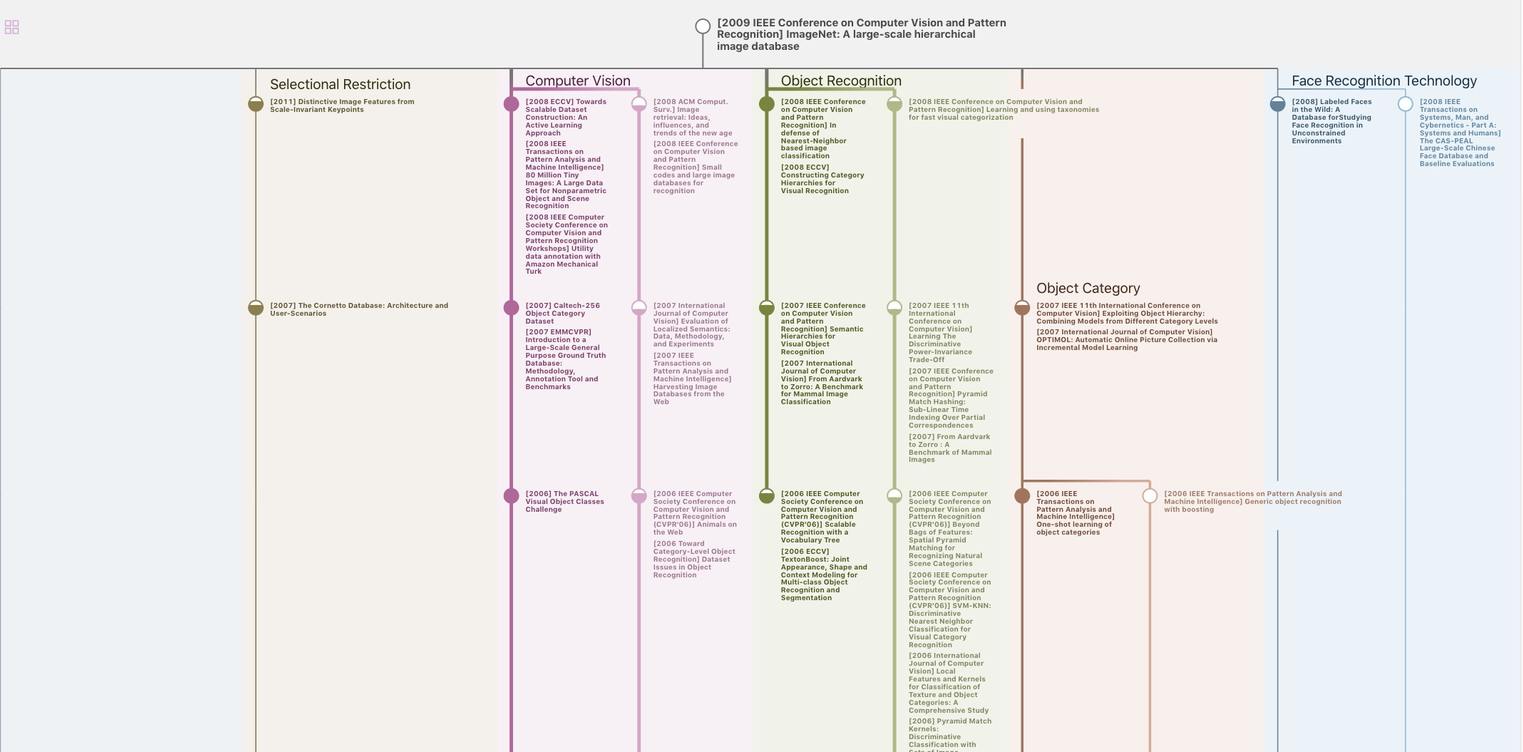
生成溯源树,研究论文发展脉络
Chat Paper
正在生成论文摘要