Cheat Detection Through Temporal Inference of Constrained Orders for Subsequences
2022 IEEE Fifth International Conference on Artificial Intelligence and Knowledge Engineering (AIKE)(2022)
摘要
For select domains and datasets, duplicates may be, in part or in whole, instances of cheating. We may specifically observe this for Sony's PlayStation Network (PSN) that services the world's most popular gaming platform. The key to cheat detection in like domains is the ability to perform temporal deduplication. Temporal data is increasingly prevalent and is not well suited to traditional similarity and distance-based deduplication techniques. We strengthen the well-established Adaptive Sorted Neighborhood Method (ASNM) with an approach for temporal data domains (
$\text{ASNM}+\text{LCS}$
) that applies ASNM, infers attribute metadata, and further detects duplicates through inference of temporal ordering requirements using Longest Common Subsequence (LCS) for records of a shared type. Using LCS, we split each record's temporal sequence into constrained and unconstrained sequences. We flag suspicious (errant) records that are non-adherent to the inferred constrained order and we flag a record as a duplicate if its unconstrained order, of sufficient length, matches that of another record. ASNM and
$\text{ASNM}+\text{LCS}$
were evaluated against a labeled dataset of 22,794 records from PSN trophy data where duplication may be indicative of cheating.
$\text{ASNM}+\text{LCS}$
F1 scores outperformed ASNM at every similarity threshold with at least 32% improvement. ASNM's best performance was an F1 of. 708 at the 0.99 threshold;
$\text{ASNM}+\text{LCS}$
yielded an F1 of. 938. The significant performance improvement costs little overhead as
$\text{ASNM}+\text{LCS}$
averaged only 3.79% additional runtime.
更多查看译文
关键词
cheat detection,temporal data,record deduplication,record linkage
AI 理解论文
溯源树
样例
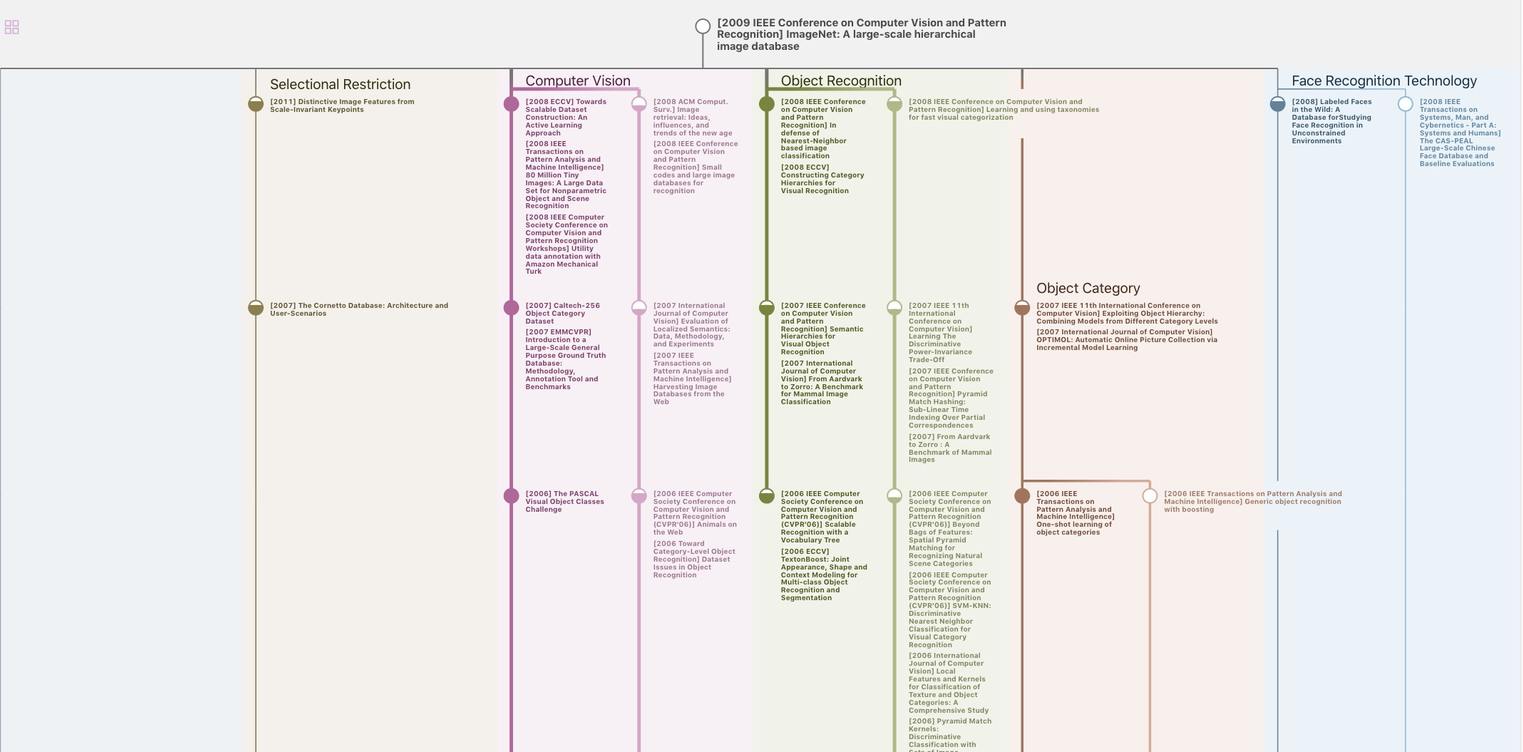
生成溯源树,研究论文发展脉络
Chat Paper
正在生成论文摘要