Ethical and Sustainability Considerations for Knowledge Graph based Machine Learning
2022 IEEE Fifth International Conference on Artificial Intelligence and Knowledge Engineering (AIKE)(2022)
摘要
Artificial Intelligence (AI) and Machine Learning (ML) are becoming common in our daily lives. The AI-driven processes significantly affect us as individuals and as a society, spanning across ethical dimensions like discrimination, misinformation, and fraud. Several of these AI & ML approaches rely on Knowledge Graph (KG) data. Due to the large volume and complexity of today's KG-driven approaches, enormous resources are spent to utilize the complex AI approaches. Efficient usage of the resources like hardware and power consumption is essential for sustainable KG-based ML technologies. This paper introduces the ethical and sustainability considerations, challenges, and optimizations in the context of KG-based ML. We have grouped the ethical and sustainability aspects according to the typical Research & Development (R&D) lifecycle: an initial investigation of the AI approach's responsibility dimensions; technical system setup; central KG data analytics and curating; model selection, training, and evaluation; and final technology deployment. We also describe significant trade-offs and alternative options for dedicated scenarios enriched through existing and reported ethical and sustainability issues in AI-driven approaches and research. These include, e.g., efficient hardware usage guidelines; or the trade-off between transparency and accessibility compared to the risk of manipulability and privacy-related data disclosure. In addition, we propose how biased data and barely explainable AI can result in discriminating ML predictions. This work supports researchers and developers in reflecting, evaluating, and optimizing dedicated KG-based ML approaches in the dimensions of ethics and sustainability.
更多查看译文
关键词
Semantic Processing,Knowledge Graphs,Machine Learning,AI Ethics,Sustainable Machine Learning,Explainable AI,RDF
AI 理解论文
溯源树
样例
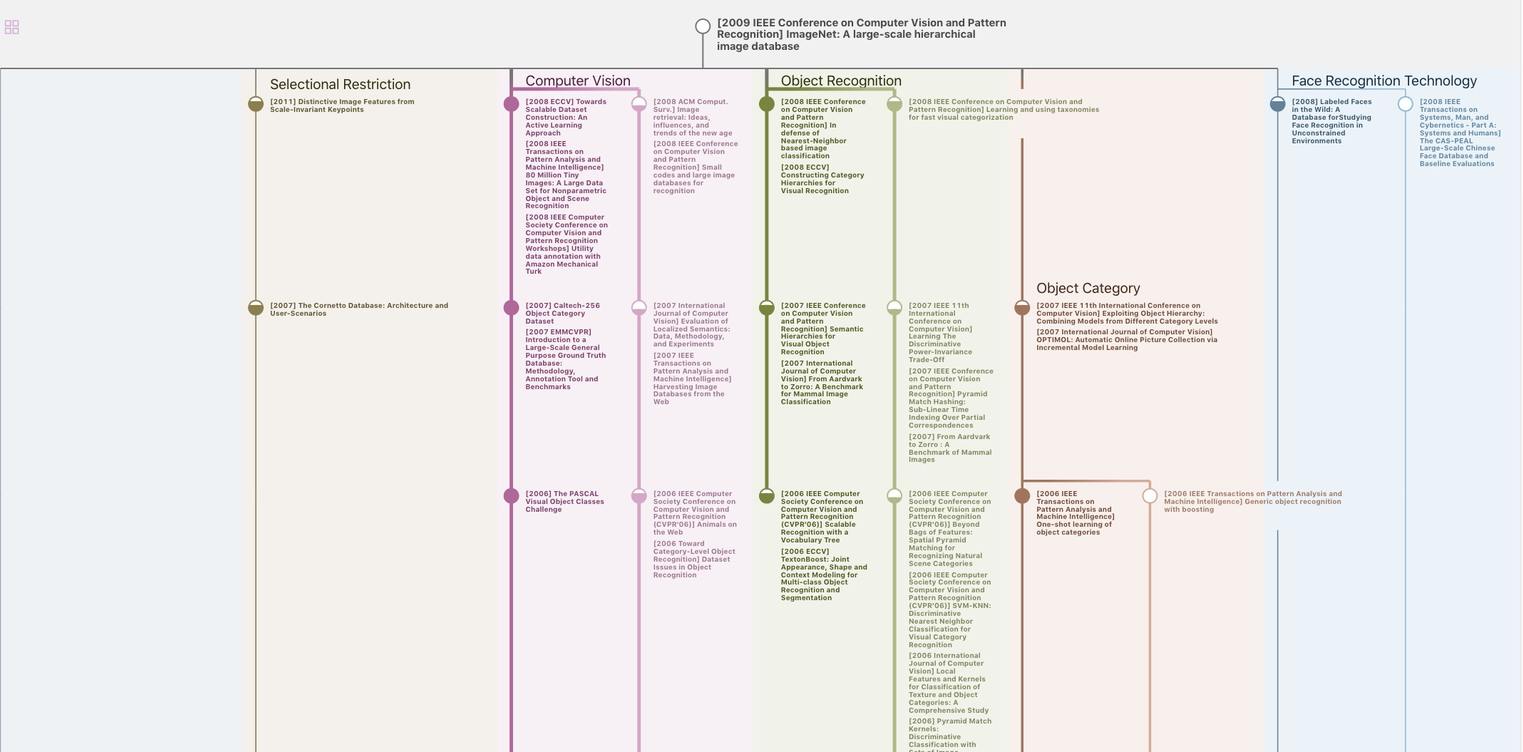
生成溯源树,研究论文发展脉络
Chat Paper
正在生成论文摘要