BEM: Bit-level Sparsity-aware Deep Learning Accelerator with Efficient Booth Encoding and Weight Multiplexing
2022 IEEE 4th International Conference on Circuits and Systems (ICCS)(2022)
摘要
The floating-point weights of multiple trained deep neural networks (DNN) models reveal abundant bit-level sparsity and continuity in the mantissa. To better accommodate these features and hence speed up DNN inference. We Proposed BEM, a hardware runtime-acceleration technique that focuses on bit-level sparsity and bit-continuity. It employs efficient booth-encoding and weight multiplexing to reduce trivial computations during DNN inference and power loss significantly. The fundamental idea is to categorize encoded bits based on their specialized actions, eliminating the need for repetitive operation judgments, and altering calculating methods for different encoded bits. We investigated three standard image recognition models, Resnet18, Densenet121, and Resnext101, and discovered the following results: (1) no accuracy loss (0%), (2) 2.08x inference speedup over the original model, and (3) at least 2.76x efficiency boost on DNN inference over standard booth encoding.
更多查看译文
关键词
bit-level sparsity,efficient booth encoding,weight multiplexing
AI 理解论文
溯源树
样例
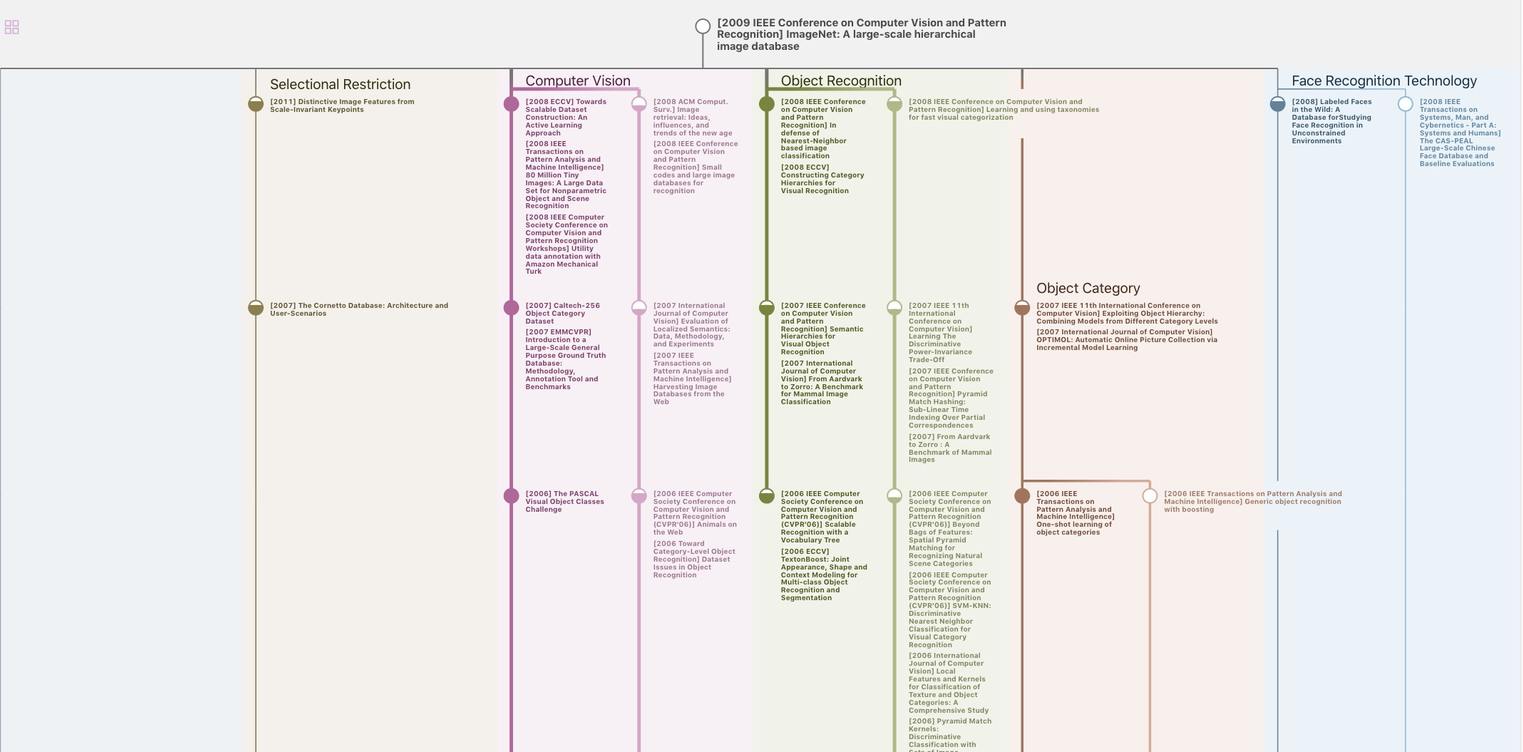
生成溯源树,研究论文发展脉络
Chat Paper
正在生成论文摘要