Development of In Silico Models for Predicting Potential Time-Dependent Inhibitors of Cytochrome P450 3A4
MOLECULAR PHARMACEUTICS(2022)
摘要
Cytochrome P450 3A4 (CYP3A4) is one of the major drug metabolizing enzymes in the human body and metabolizes similar to 30-50% of clinically used drugs. Inhibition of CYP3A4 must always be considered in the development of new drugs. Time-dependent inhibition (TDI) is an important P450 inhibition type that could cause undesired drug-drug interactions. Therefore, identification of CYP3A4 TDI by a rapid convenient way is of great importance to any new drug discovery effort. Here, we report the development of in silico classification models for prediction of potential CYP3A4 time-dependent inhibitors. On the basis of the CYP3A4 TDI data set that we manually collected from literature and databases, both conventional machine learning and deep learning models were constructed. The comparisons of different sampling strategies, molecular representations, and machine-learning algorithms showed the benefits of a balanced data set and the deep-learning model featured by GraphConv. The generalization ability of the best model was tested by screening an external data set, and the prediction results were validated by biological experiments. In addition, several structural alerts that are relevant to CYP3A4 time-dependent inhibitors were identified via information gain and frequency analysis. We anticipate that our effort would be useful for identification of potential CYP3A4 time-dependent inhibitors in drug discovery and design.
更多查看译文
关键词
time-dependent inhibitors,machine learning,deep learning,in silico models
AI 理解论文
溯源树
样例
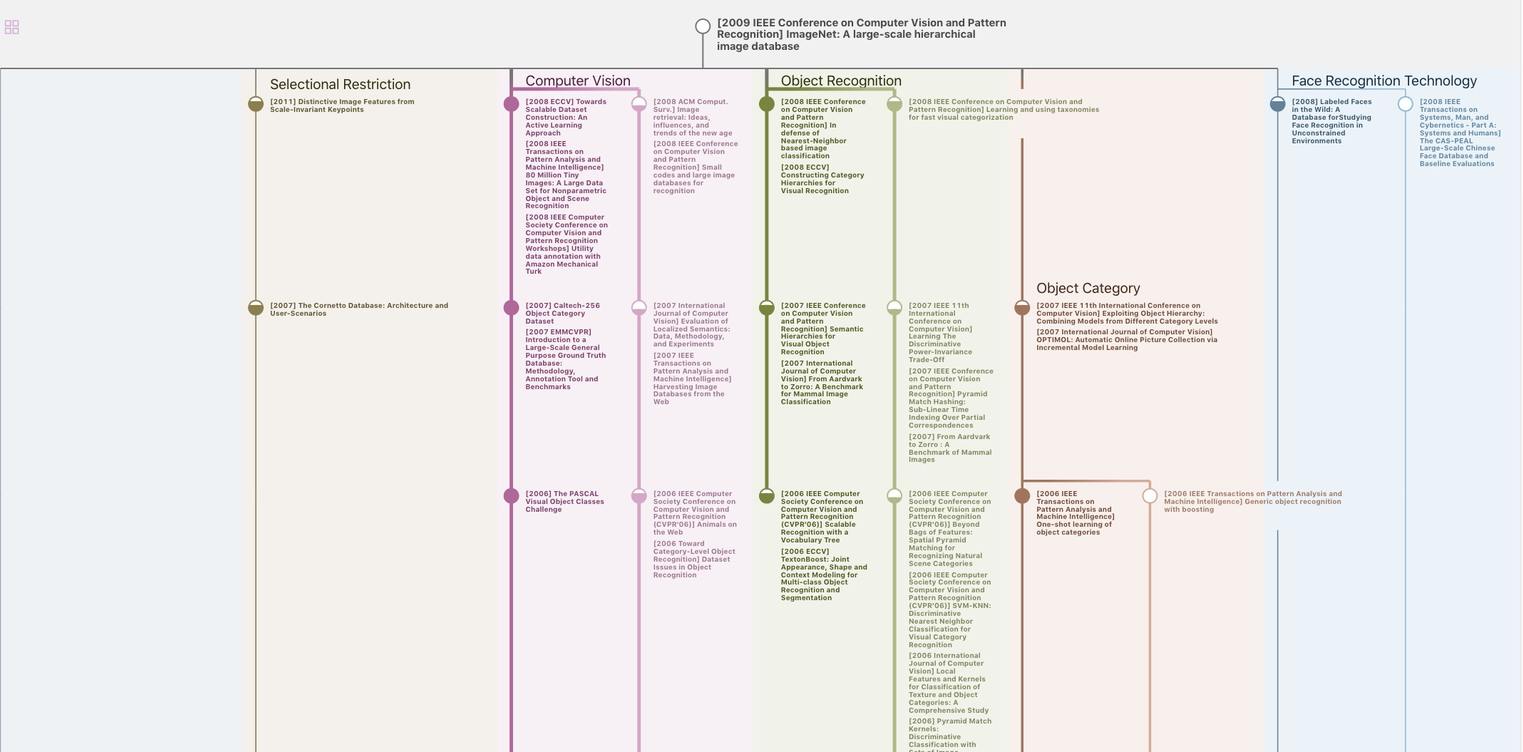
生成溯源树,研究论文发展脉络
Chat Paper
正在生成论文摘要