Guarding Against Universal Adversarial Perturbations in Data-driven Cloud/Edge Services
2022 IEEE International Conference on Cloud Engineering (IC2E)(2022)
摘要
Although machine learning (ML)-based models are increasingly being used by cloud-based data-driven services, two key problems exist when used at the edge. First, the size and complexity of these models hampers their deployment at the edge, where heterogeneity of resource types and constraints on resources is the norm. Second, ML models are known to be vulnerable to adversarial perturbations. To address the edge deployment issue, model compression techniques, especially model quantization, have shown significant promise. However, the adversarial robustness of such quantized models remains mostly an open problem. To address this challenge, this paper investigates whether quantized models with different precision levels can be vulnerable to the same universal adversarial perturbation (UAP). Based on these insights, the paper then presents a cloud-native service that generates and distributes adversarially robust compressed models deployable at the edge using a novel, defensive post-training quantization approach. Experimental evaluations reveal that although quantized models are vulnerable to UAPs, post-training quantization on the synthesized, adversarially-trained models are effective against such UAPs. Furthermore, deployments on heterogeneous edge devices with flexible quantization settings are efficient thereby paving the way in realizing adversarially robust data-driven cloud/edge services.
更多查看译文
关键词
Adversarial machine learning,universal perturbation,edge computing,quantization,hardware acceleration
AI 理解论文
溯源树
样例
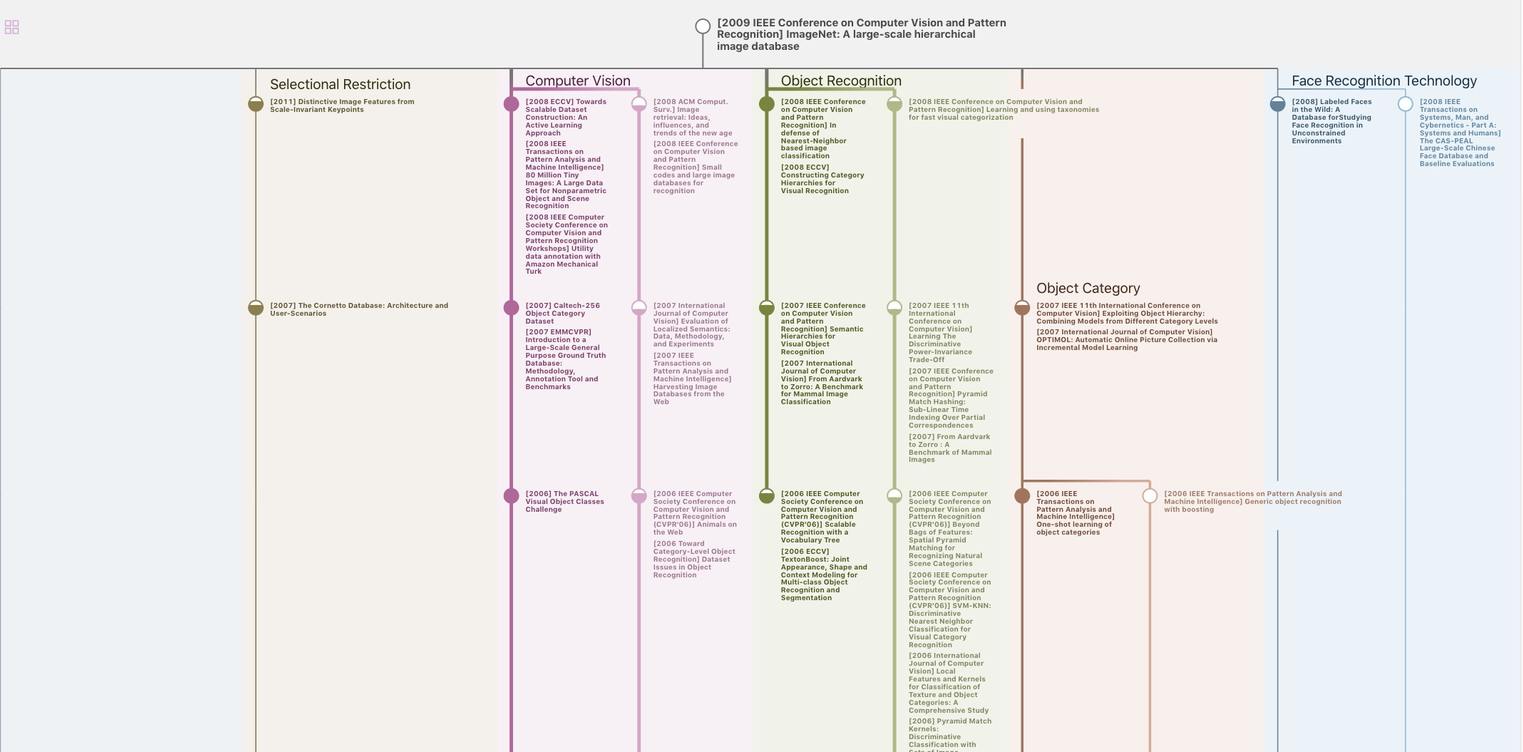
生成溯源树,研究论文发展脉络
Chat Paper
正在生成论文摘要